
Run Autonomous A/B Tests with Fibr’s AI
Run Autonomous A/B Tests with Fibr’s AI
Run Autonomous A/B Tests with Fibr’s AI
Let Fibr’s AI take care of your A/B tests end to end. From generating testing themes, identifying elements and generating test variations in one go.
Let Fibr’s AI take care of your A/B tests end to end. From generating testing themes, identifying elements and generating test variations in one go.
Let Fibr’s AI take care of your A/B tests end to end. From generating testing themes, identifying elements and generating test variations in one go.



Backed by leaders from
Benefits
Boost Your Campaigns with Smart A/B Testing
Features
Fibr: Your All-in-One A/B Testing
Automation Platform
Fibr: Your All-in-One A/B Testing Automation Platform
Effortless Variation Creation
Fibr's AI analyzes your ad creatives and automatically generates high-performing variations. No coding or design expertise needed.
Data-Driven Decisions, Faster
Get instant insights into winning variations. Optimize campaigns continuously with Fibr’s intuitive analytics dashboard
Focus on Strategy, Not Busywork
Ditch the manual edits. Fibr handles the entire process, freeing you to strategize and innovate.
Tailored Testing
Customize your testing parameters such as audience segmentation, traffic allocation, and more to get greater control over your experiments.
Effortless Variation Creation
Fibr's AI analyzes your ad creatives and automatically generates high-performing variations. No coding or design expertise needed.


Focus on Strategy, Not Busywork
Ditch the manual edits. Fibr handles the entire process, freeing you to strategize and innovate


Data-Driven Decisions, Faster
Get instant insights into winning variations. Optimize campaigns continuously with Fibr’s intuitive analytics dashboard


Tailored Testing
Customize your testing parameters such as audience segmentation, traffic allocation, and more to get greater control over your experiments.


wall of love
Our customers
us

This app just knows the exact pain points D2C businesses have and are trying to solve it. It's not just about customized landing pages, it's also about amazing service by the team. Would recommend the product to anyone and everyone in the D2C community.

Dhruv
CEO & Founder
This app just knows the exact pain points D2C businesses have and are trying to solve it. It's not just about customized landing pages, it's also about amazing service by the team. Would recommend the product to anyone and everyone in the D2C community.

Dhruv
CEO & Founder
This app just knows the exact pain points D2C businesses have and are trying to solve it. It's not just about customized landing pages, it's also about amazing service by the team. Would recommend the product to anyone and everyone in the D2C community.

Dhruv
CEO & Founder
Fibr sounds like a gamechanger! We're looking forward to using fibr to elevate our customer conversions!

Siddhi Nagre
Founder
Fibr sounds like a gamechanger! We're looking forward to using fibr to elevate our customer conversions!

Siddhi Nagre
Founder
Fibr sounds like a gamechanger! We're looking forward to using fibr to elevate our customer conversions!

Siddhi Nagre
Founder
Optimizing marketing efforts requires precision, and Fibr has become our precision instrument. Fibr’s no-code Ad connect feature seamlessly has resulted in a more efficient funnel, reducing costs and maximizing the impact of our campaigns.

Derek Anderson
Software Engineer
Optimizing marketing efforts requires precision, and Fibr has become our precision instrument. Fibr’s no-code Ad connect feature seamlessly has resulted in a more efficient funnel, reducing costs and maximizing the impact of our campaigns.

Derek Anderson
Software Engineer
Optimizing marketing efforts requires precision, and Fibr has become our precision instrument. Fibr’s no-code Ad connect feature seamlessly has resulted in a more efficient funnel, reducing costs and maximizing the impact of our campaigns.

Derek Anderson
Software Engineer
I've explored multiple tools for landing page testing and Fibr is most impressive. Took barely a few mins to deploy & saw great results: ~15% reduction in CAC. The team is very easy to work with & implement feedback - if you are a DTC brand, I highly recommend Fibr!

Rishabh Bothra
VP, Growth
I've explored multiple tools for landing page testing and Fibr is most impressive. Took barely a few mins to deploy & saw great results: ~15% reduction in CAC. The team is very easy to work with & implement feedback - if you are a DTC brand, I highly recommend Fibr!

Rishabh Bothra
VP, Growth
Fibr is designed to maximize conversions! The user experience was seamless, making it incredibly easy to use. Fibr has an innovative approach to creating personalized shopping experiences, while ensuring that making landing pages is as easy as possible. It makes A/B testing so easy and scalable. I'm optimistic about the benefits it offers

Karan Dubey
Entrepreneur
Fibr is designed to maximize conversions! The user experience was seamless, making it incredibly easy to use. Fibr has an innovative approach to creating personalized shopping experiences, while ensuring that making landing pages is as easy as possible. It makes A/B testing so easy and scalable. I'm optimistic about the benefits it offers

Karan Dubey
Entrepreneur
Fibr's landing pages with all collections-in-one make selling a total breeze. It's so easy for our consumers to quickly review all our products, and for me to track my conversions across channels and specific ads. The onboarding process was also a breeze, thanks to their super helpful team for step-by-step guidance. Would highly reccomend!

Rohit Ganapathy
Head, Middle East
Fibr's landing pages with all collections-in-one make selling a total breeze. It's so easy for our consumers to quickly review all our products, and for me to track my conversions across channels and specific ads. The onboarding process was also a breeze, thanks to their super helpful team for step-by-step guidance. Would highly reccomend!

Rohit Ganapathy
Head, Middle East
Ads and our website were like strangers before Fibr. High bounce rates, low conversions. Fibr changed everything. Now, our ads and landing pages match seamlessly. We witnessed our conversions go up by almost 2X. It is a must-have for every marketer.
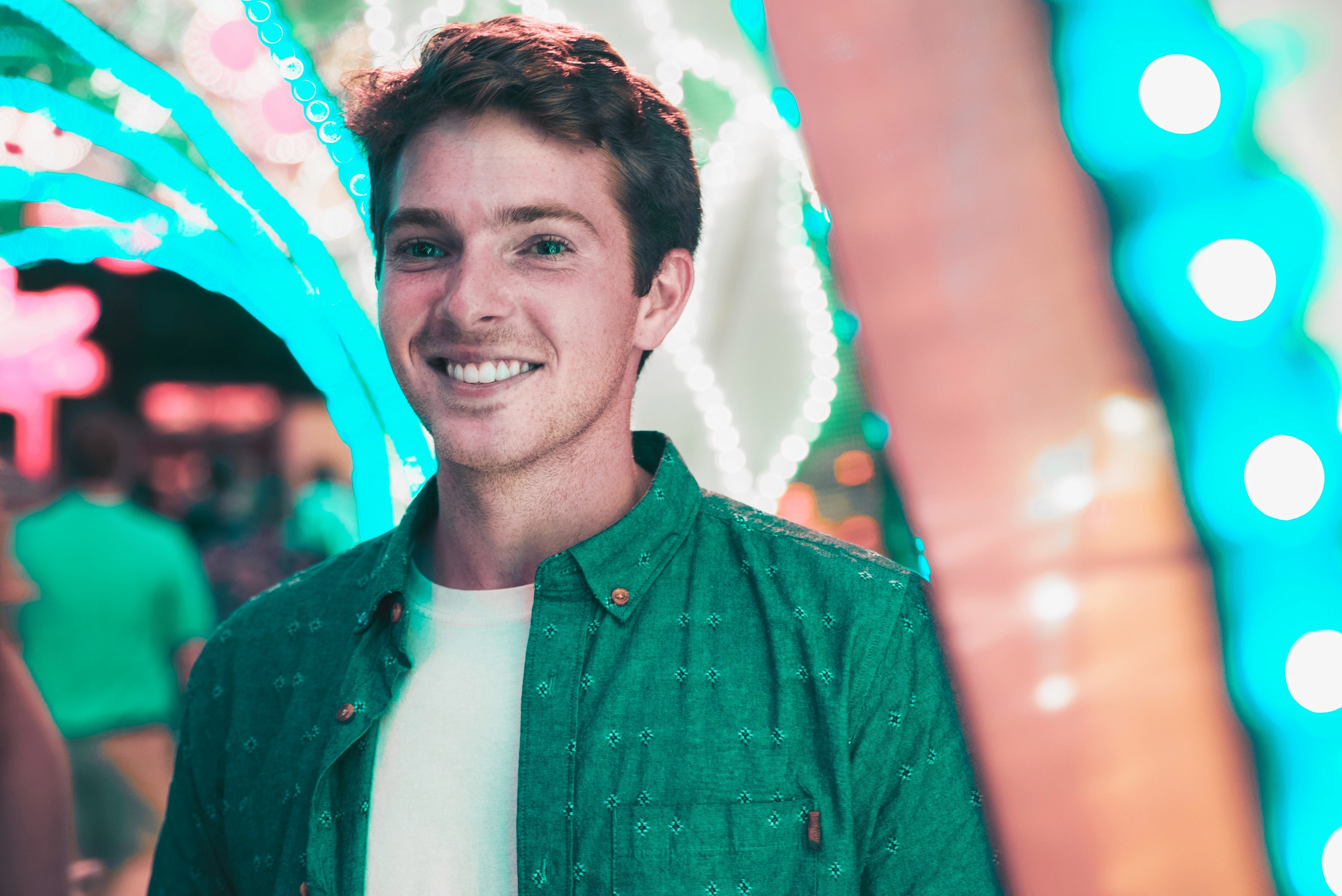
Evan Turner
Online Marketing Specialist
Fibr is a conversion booster! No more disconnect between ads and landing pages. It's user-friendly and powerful. Our ROI has never looked better. Fibr is the ace up our marketing sleeve.

Alex Carter
Performance Marketing Lead
As a brand with heavy influencer marketing, fibr has been a game-changer. With fibr, we now make super personalised influencer specific shops & track sales contribution of each influencers, giving us much better influencer ROI. So easy to make informed decisions! Overall, highly recommend fibr to anyone looking to accelerate their influencer marketing!

Garima Juneja
CEO & Founder
As a brand with heavy influencer marketing, fibr has been a game-changer. With fibr, we now make super personalised influencer specific shops & track sales contribution of each influencers, giving us much better influencer ROI. So easy to make informed decisions! Overall, highly recommend fibr to anyone looking to accelerate their influencer marketing!

Garima Juneja
CEO & Founder
As a brand with heavy influencer marketing, fibr has been a game-changer. With fibr, we now make super personalised influencer specific shops & track sales contribution of each influencers, giving us much better influencer ROI. So easy to make informed decisions! Overall, highly recommend fibr to anyone looking to accelerate their influencer marketing!

Garima Juneja
CEO & Founder
Our journey with Fibr has been nothing short of transformative. The reduction in customer acquisition costs and the boost in conversions are evident, and our overall ROI has never looked better. A must-have for any growth-focused team.

Michael Harris
Chief Growth Officer
Our journey with Fibr has been nothing short of transformative. The reduction in customer acquisition costs and the boost in conversions are evident, and our overall ROI has never looked better. A must-have for any growth-focused team.

Michael Harris
Chief Growth Officer
Our journey with Fibr has been nothing short of transformative. The reduction in customer acquisition costs and the boost in conversions are evident, and our overall ROI has never looked better. A must-have for any growth-focused team.

Michael Harris
Chief Growth Officer
Fibr turned lead generation into a science. Creating personalized landing pages at scale is no longer a challenge. It's user-friendly, efficient, and our leads are more qualified than ever.

Oscar Bennett
Lead Generation Expert
Fibr turned lead generation into a science. Creating personalized landing pages at scale is no longer a challenge. It's user-friendly, efficient, and our leads are more qualified than ever.

Oscar Bennett
Lead Generation Expert
Fibr turned lead generation into a science. Creating personalized landing pages at scale is no longer a challenge. It's user-friendly, efficient, and our leads are more qualified than ever.

Oscar Bennett
Lead Generation Expert
Blogs
fibr insights
Start your personalization journey with Fibr
In a world without cookies, Fibr's AI-powered personalization hub delivers unique experiences like no other. Boost your ROAS, cut down CAC, and increase conversions!

Start your personalizalion journey with Fibr
In a world without cookies, Fibr's AI-powered personalization hub delivers unique experiences like no other. Boost your ROAS, cut down CAC, and increase conversions!

Start your personalizalion journey with Fibr
In a world without cookies, Fibr's AI-powered personalization hub delivers unique experiences like no other. Boost your ROAS, cut down CAC, and increase conversions!

Copyright ©SeamlessAI. All rights reserved.
Copyright ©SeamlessAI. All rights reserved.
Copyright ©SeamlessAI. All rights reserved.