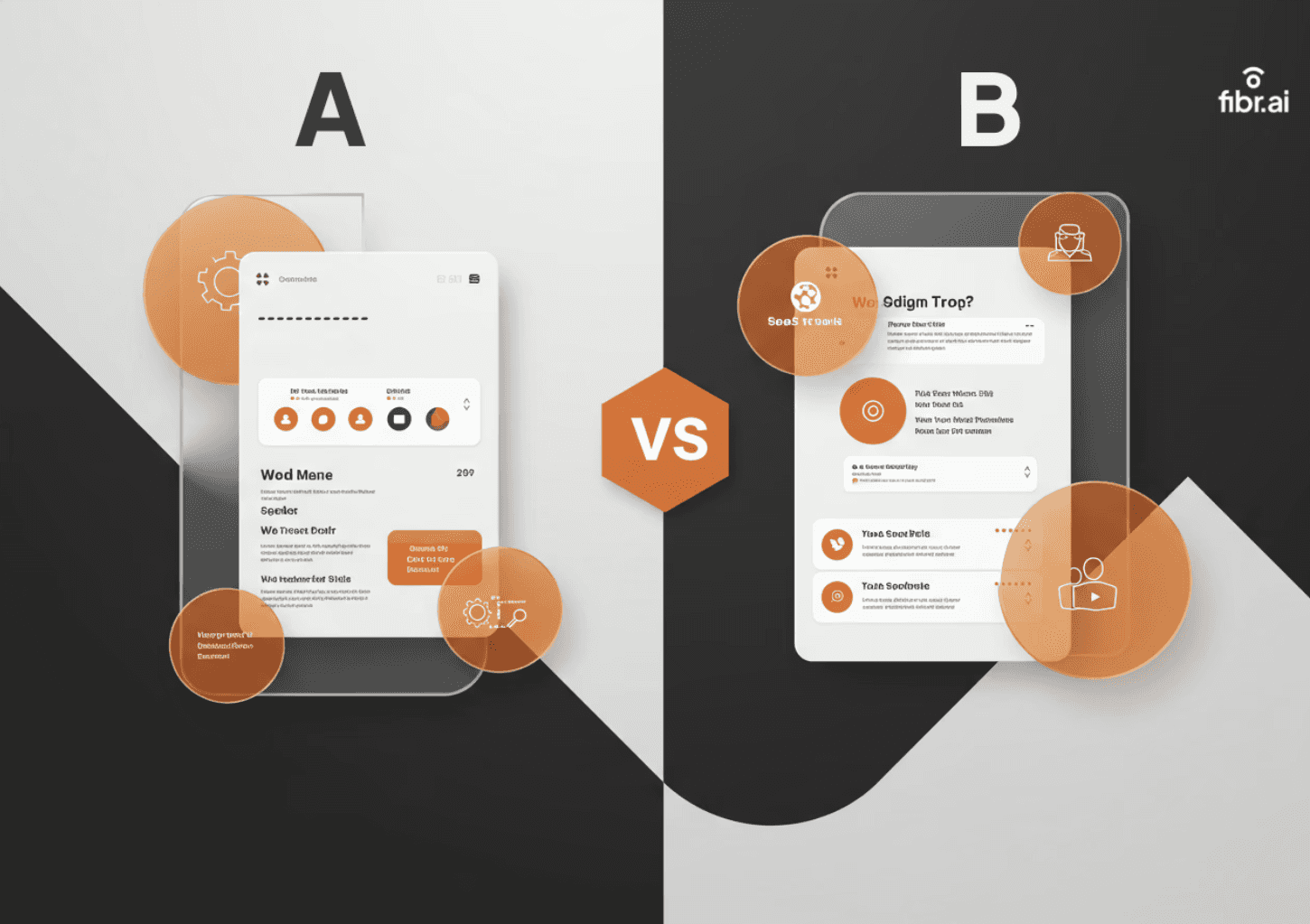

Pritam Roy
If you’ve ever sat with your team discussing why the sign-ups are low or why the conversion needle is almost never moving, then it’s most likely that you are discussing a hypothesis problem.
Hypothesis is the base of why you conduct an A/B test. You find an issue, and then you form a hypothesis regarding how to fix the issue. Without a proper hypothesis, you’re just guessing away at your conversion problems, and guessing rarely leads to success.
If you’re looking to craft a strong A/B testing hypothesis deeply and understand how it works in real life, you’ve come to the right place. In this guide, we explain hypothesis generation in simple terms–no jargon–just clean, actionable steps to help your experiments take off.
What is an A/B testing hypothesis?
A/B testing hypothesis can simply be defined as making an ‘educated guess’ on a specific theory or change to see how it impacts user behavior or performance metrics. You can also think of it as a statement that predicts how an A/B test will perform, or what results the test would bring.
For instance, imagine you’re testing the subject line of your festive sale marketing email. You ‘assume’ or ‘make a guess’ or ‘predict’ that having a subject line like— ‘Hey, there, did you check your 20% discount coupon’ can increase your opening rates because it is more personalized and comes with an exciting offer. This is your ‘hypothesis.’
Did you note how here you are predicting the outcome (higher opening rates) and also providing an answer or explanation (more personalization) as to why it would work? That’s what the A/B testing hypothesis does. It predicts results/outcomes and provides a rational explanation of what you can achieve by making small or big changes.
Your hypothesis can be anything–maybe changing the CTA button size can increase conversions could be one hypothesis you think might work. Or making the headline shorter, changing the CTA wording, adding a video–literally anything!
Remember that hypothesis is the basis of any A/B testing or experiment. It gives your experimentation processes a proper start, without which you may be conducting random tests with no means to measure success.
And the best part about a hypothesis is that it ensures you learn something valuable, regardless of whether the hypothesis turned out to be a success or not. For instance, if your hypothesis turns out to be correct, you have found a change that works. In case, it fails, you gain insights into what’s not working–either way you move closer to your audience’s expectations and improve results!
Now, you may have a lot of questions:
How is a hypothesis formed?
Does the hypothesis only rely on guesswork and random thoughts?
How do I know that my hypothesis will work?
Do all hypotheses work?
How do I create a strong hypothesis?
Don’t worry, we cover all these questions, basic doubts, and more as we progress in this blog. Let’s first figure out how you carve out a strong hypothesis. That should answer many of the above-mentioned questions.
Building a strong A/B testing hypothesis
Now, that you understand how a hypothesis typically works, let’s understand how to build a strong A/B testing hypothesis–
1.Rely on data and not guesswork
‘Increasing the size of the CTA button could increase conversions’
Or
‘Increasing the size of CTA by 20% could increase conversions by 5%’
Of the two sentences, which hypothesis looks solid to you? One that is vague and random and does not let you measure success properly or the other that speaks with data and numbers? You are most likely to say yes to the latter. The reason is it is clear, is more reliable, and eliminates guesswork.
Data is quite literally (and arguably) the best way to ensure you’re hypothesis is solid, has a good chance to bring in positive changes (higher CTR, conversions), and eliminate any guesswork.
Dive deeper into Google Analytics, heatmap, session recordings, interviews, forms, and more to understand user behavior and spot out pain points and theories.
For instance, let’s say you discover that more than 50% of customers do not move from the second last step to the checkout page. That’s a good stage to build a hypothesis. Why is this happening? Is there some friction on the page? Is the ‘CTA’ or ‘Move to the next page’ not clear? By asking these questions, you are trying to figure out the reason; and if you do find a solid doubt or issue, your hypothesis can come from the same.
Taking the same example, assume that on thorough analysis, you realize that a trust factor is missing on the page. Use this insight to form a hypothesis – ‘Adding rating and social proof can reduce page bounce rate up to 10% and increase conversions by 7%’
You must have noted how the hypothesis speaks on numbers– ‘10% and 7%.’ They come from data and data alone. So, instead of making vague statements like ‘Adding rating and social proof can reduce page bounce rate and increase conversions’ data analysis ensures you’re not throwing random darts in the dark and have a solid reliable theory to stand on.
Fibr AI’s AI-powered experimentation expert, Max is changing traditional A/B testing methods, right from hypothesis generation to execution. Rely only on data and eliminate fluff of any type, all in an automated format. No more guesswork and making prayers!
Book a demo with Fibr AI today.
2.Be clear and specific
Imagine for a second your car is having some trouble. What do you think is going to help your car? Is it correctly diagnosing different parts to spot issues or just random generic analysis? You already know the answer. As far as A/B testing is concerned, your website, app, or landing page is no different.
Instead of going in circles, you are better off being super clear and specific in your hypothesis. Remember that a good hypothesis is not confusing.
Stating a hypothesis like–‘Maybe changing the CTA button color and the image size a bit could increase business’ is the starting point of a failed A/B testing.
Maybe changing the CTA button: Why the ‘maybe’ and ‘CTA’ buttons? The issue could also be that the CTA is misplaced or not visible. Or the CTA may not be an issue at all!
Image size a bit: Again, what do you think ‘image is the issue’ or the ‘size of the image’ is a problem?
Increase business: What business? What is your end goal out of the A/B testing? Is it to increase CTRs, boost conversions, or anything else?
Do you see the issue here? There is no point in moving forward with this kind of A/B testing hypothesis. It’s obviously not going to work out.
Now, look at this hypothesis–‘Changing the CTA color from blue to red can help boost conversions by 6%.’ Don’t you think this is a more clear and clean hypothesis? The element in question is clear (CTA), the change you’re going to make is clear (blue to red) and the result you can expect is also clear (6% conversion increase).
3.Avoid multivariate testing at the beginning
It can be tempting to test many variables together to get faster results. But this might not be the wisest thing to do because testing too many variables together can make it difficult to spot which element was actually creating friction and which change was bringing in the results, if any.
For instance, assume through data analysis, you form a hypothesis that placing the CTA button at the center of the page can boost conversions by 10%. You also learn that adding a video can increase conversions by 15%. Let’s say you went ahead and changed the CTA and also added the video.
Upon analysis, you learn that the conversion rates increased by 13%. Now, how do you know which element has caused this change? Or what element confirmed your hypothesis?
Understand that multivariate testing is an amazing methodology to simultaneously test several elements together and get faster results. But, if you’re just beginning and do not have solid data analysis to rely on, changing too many elements in one go can create confusion for you (and even your customers in some cases) and can make hypothesis creation processes super tricky.
Making a change by keeping all other elements unaltered and removing any external disturbance is the best way to test your hypothesis for its worth.
4.Keep it actionable, simple, and testable
You definitely want to keep your hypothesis simple, to the point, and something that can be actually tested in real life.
Let’s say you predict that changing the font of your website can impact conversion rates. This is a very vague sentence. Plus, many CRO experts would agree that font is not that important a factor. There is a good chance that such a change may not impact conversions or revenue in any form.
In similar cases, you risk wasting time and resources on hypotheses and theories that most likely would yield nothing. It is thus super important that your theories are actionable and have the potential to bring in a positive business impact.
Key components of a strong A/B testing hypothesis
So far, we have understood what an A/B testing hypothesis is and how to build a strong hypothesis. But what if we tell you that your business will never have to worry about getting a hypothesis wrong through a tried and tested formula? Yup, that’s right!
Certain components ensure your hypotheses are strong, actionable, solution-oriented, and clean. What are those components? Read below:
1.Detecting a problem
The first component required for any hypothesis formulation is detecting a problem. This could involve deeply analyzing common metrics like conversion rates, CTRs, bounce rates, average session duration, cart abandonment rate, and more.
For instance–
Our website sign-up rate is 3% lower than the typical industry standards, or
Our cart abandonment rate is 20% higher than X, Y, and Z competitors or
Once you locate a problem, whether through generic analysis, or data deep dives, you have formed a base for your hypothesis. For instance, in the first example above, you suddenly have clarity that sign-up rates being lower than the industry average is a problem and you need to address it to increase revenue and conversion rates.
2.Presenting a solution
Once you gain insights into the problem you can narrow your focus quickly to address it. Outline the specific changes or interventions you believe could help resolve the issue. But ensure that it should be actionable and measurable (like we discussed above).
Taking the above example, assume that you discover that the CTA button is extremely small/nearly invisible. You also assume that this could be the reason for low conversions on the website. Now, after completing the research, you propose increasing the size of the CTA button.
This solution is what you’re going to test against the existing variables.
3.The outcome
Your outcome is where you outline what you expect from conducting the A/B testing. Again, taking the same example, here your outcome could look like–increasing the sign-ups by 8%.
By specifying the outcome, you provide a metric for results to be tested against. If the sign-ups increased only by 2%, you know something went wrong–whether it was the hypothesis, the element, or the chosen problem and solution. It could so happen that the CTA was never the problem, maybe it was not having social proof.
Do you see how by integrating 3 ingredients–problem, solution, and outcome, you solidify your hypothesis generation and have a better chance at success?
Where to find hypothesis ideas?
Good question! Let’s be honest, coming up with a strong, meaningful hypothesis can be hard; but as far as the A/B testing hypothesis is concerned, data is your true best friend. There is arguably no better place to detect anomalies and find customer pain points.
The minute you convert a pain point into a hypothesis, you positively boost your chance of higher conversions. But if your data is not telling a story, start looking around. What problems do you typically face when you use an app or website? Is that ‘typical’ problem present on your page too? Often, the problem is right in the front, but our biases can prevent us from seeing the gaps and inconsistencies.
Competitor analysis can be another excellent source to craft a hypothesis. Conduct deep audits of what’s working for your competitors and what’s not. This can help you better understand the behavior of your target audience, which in this case is the same.
Also, do not negate the importance of a good academic paper, article, or case study. These are sometimes goldmines for hypothesis ideas. Don’t just stick to one field. Even if you come from the SaaS industry, there is no harm in understanding how the eCommerce industry formulates and tests its hypotheses. In fact, a shift like this can spark curiosity and help test unique ideas.
Conversations with peers, customers, experts, and even those outside your field can also give you a good chance to listen to their challenges and curiosities. You never know, a simple chat can lead to a groundbreaking thesis.
And let’s not forget artificial intelligence (AI) and tech. In 2025, if you’re not employing the latest in these fields, you’re missing out. Test different APIs, prompts, and more to conduct deeper analysis and have a continuous flow of fresh ideas always.
Last but not least, stay curious. Follow trends, and news and always ask ‘Why?’ Sometimes the best A/B testing hypothesis can come from personal experiences and just looking in plain sight.
Testing, measuring, and iterating
It is very important to understand that formulating a hypothesis is quite literally the first step of the entire A/B testing process. Testing, measuring, and iterating, all of which are integral to A/B testing help you understand what’s working, what’s not, and how to improve. Let’s quickly understand how:
Testing: This is putting your hypothesis in motion. For instance, ‘Changing the size of the CTA by 20% will increase conversions by 10%’. You create two versions–variations A and B. A is the original and B is the new CTA. You present both versions to a set of audiences.
Measuring: Once the test is completed, you collect the data and analyze it. How many people clicked on the CTA button? Did variation B perform better than A? If yes, by how many percentage points, if no, why? Measuring the results is the ultimate test of your hypothesis.
Iterating: Based on the results, you understand what to do next. If variation B worked, great, you can implement it. If you want to further the experiment or change the size further, you may do that too. If not, you can tweak your hypothesis, and test again. Maybe it was not the size, but the color or the button. You get the gist, right?
A/B testing hypothesis example
Now, it’s time to see how companies in real time are employing A/B testing hypotheses to increase their conversion numbers.
1.Hubspot
HubSpot Academy's Homepage was in soup. On analyzing data, the leading CRM and inbound marketing software found that on the HubSpot Academy page, only around 0.9% of visitors of the 55,000 were actually spending time on the homepage video. Not to mention, messaging was all over the place.
Hubspot decided to change this. Hubspot deployed 3 variants–A (controlled version), B and C. The variant B included more colorful text and images and an animated headline too. Variant C experimented with placements of the headline and images.



Result? Variant B was recorded to outperform Variant A by almost 6%! Variant C however underperformed by 1%. The 6% increase meant 375 more signs for Hubspot.
Partner with Fibr AI to experience A/B testing like never before
Testing waters with unclear and vague hypotheses can waste resources, time, and money. That’s something no business would want, and certainly not yours.
With Max, Fibr’s AI-powered experimentation expert, you’re A/B testing processes are going to be transformed forever. You’ll never have to wonder which element needs to be optimized to what extent; when to conduct multivariate testing; or when to test, and iterate. All processes are automated, and every aspect of your landing page, app, or website is optimized 24/7.

What makes Max special is that it can instantly identify which areas are ripe for improvement and generate specific hypotheses; meaning? You never run out of hypothesis ideas and are continuously refining your assets for better conversions and revenue.
Want to try Max? That’s a smart call! Book a quick demo with Fibr AI today and let Max take over your A/B testing worries!
FAQs
1.What is the hypothesis of the A/B test?
A hypothesis is a statement or prediction that modifying a certain element in an app or website can impact user behavior and help bring in more conversions.
2.What is AB testing with an example?
A/B testing or split testing is the process of comparing two variations–A and B of a certain element to see which one positively impacts conversions and CTRs.
3.What is alpha testing and beta testing?
Alpha testing is performed internally to check for bugs and errors. Beta testing conversely involves testing changes with limited, real time users to study their reactions and feedback. Both refine decisions based on data.