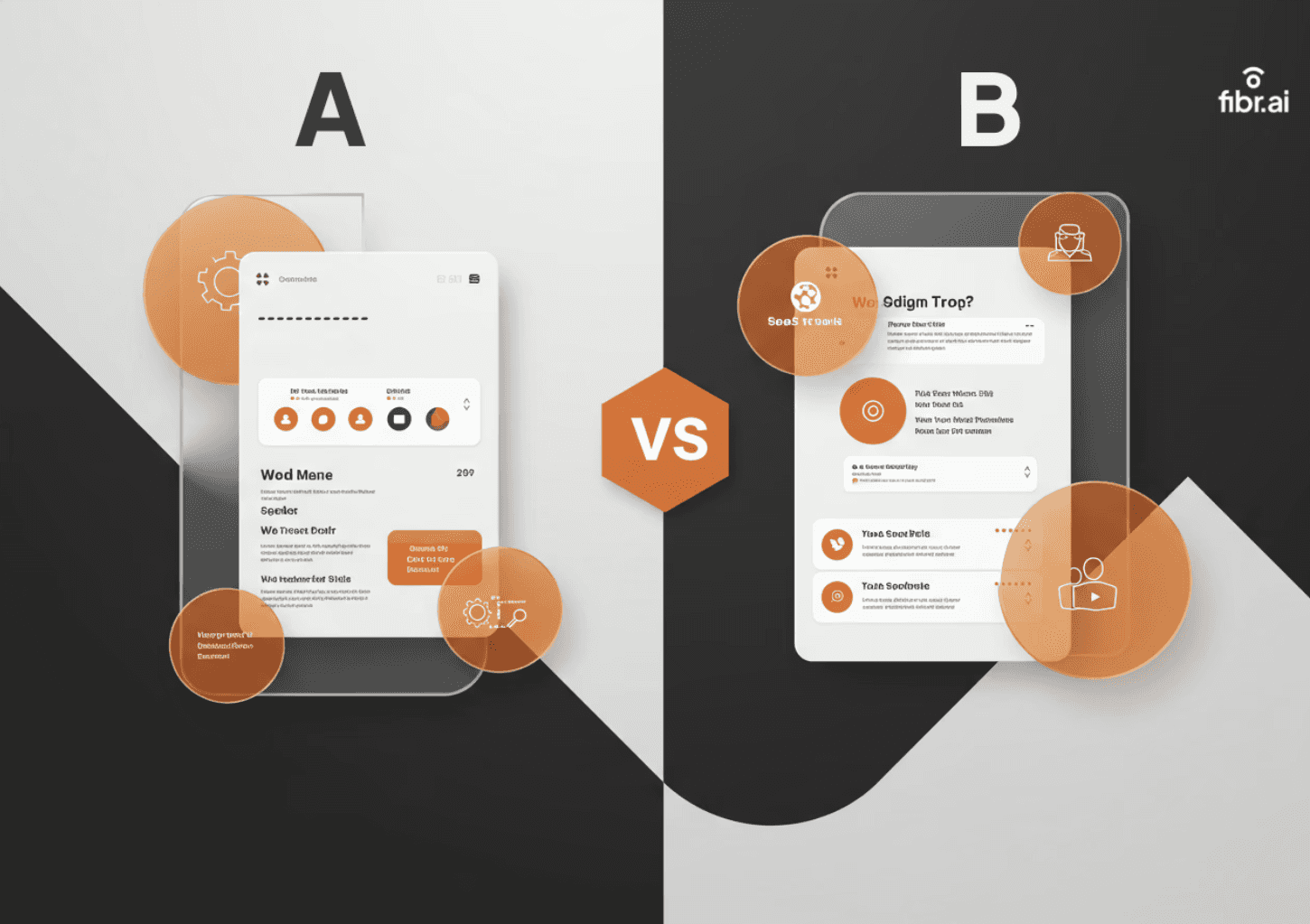

Pritam Roy
Let’s be honest. A/B testing sounds simple. You form a theory, try two versions of something, pick a winner, and implement it. But, if you’ve ever run an A/B test before, you’ll agree it’s never this straightforward. Is it? But why? Because A/B testing is not just choosing between red and blue colors.
No two tests are the same. One can bring results right away, other, not even on maximum optimization. So, what’s the difference? It often comes down to maturity–how well you understand the tools and techniques, statistics, and processes, besides your audience.
In this blog, we break it all down. What the latest A/B testing statistics say, the challenges, and the different A/B maturity stages. Can’t wait? Neither can we! Let’s start right away.
Key Takeaways
Most A/B testing statistics point toward how companies are increasingly relying on tech, performing more tests than ever, and that the trends are stronger in eCommerce, SaaS, and similar industries.
A/B testing is not devoid of challenges–sample size and statistical significance implementation errors, resource constraints and more can hinder the experimentation process.
A/B testing stages begin with basic testing based on intuition progressing toward a highly advanced and data-backed experimentation process.
For successful testing, it’s important to test for impact, segment users, measure for long-term results, and run 100 and 1000s of experiments. It’s also super important to watch out for statistical significance.
2025 A/B testing statistics and trends point toward growing AI integration, focus on data privacy law, multi-arm bandit, and personalization.
A/B testing statistics: The growth and adoption of A/B testing
Let’s start this blog with some super cool statistics to help you understand what trends are shaping which industries and how A/B experiments are turning out as a whole:
1.Around 44% of businesses rely on split testing software for experiments (99 Firms)
Is it not shocking that more than 50% of businesses do not have dedicated tools, software, and strategies when it comes to A/B testing? It could also point toward the fact that a large chunk of companies still rely on guesswork instead of data. This can be due to a lack of awareness, or resource crunch.
2.Nearly 77% of companies conduct A/B tests on their websites (99 Firms)
As digital competition and presence become fierce, this stat reconfirms that most businesses are testing different elements and variations of their website to enhance user experience and increase conversions. However, some businesses may still be reluctant to experiment due to fear of negative results, lack of team and expertise or not knowing where to start.
3.Only 1 in 8 A/B tests leads to a meaningful impact (99 Firms)
This is a stat that every marketer must make note of–if you’re conducting 10 tests, likely only 1-2 are going to produce meaningful results. It is thus paramount that you focus on a high number of data-backed experiments rather than low-impact changes like making the headline bold or changing the color of the CTA button.
4.About 58% of businesses use A/B testing to improve conversion rates (Firm 99)
Not all businesses commit to A/B testing to gain conversions. Each experiment can have a different purpose and end goal. Conversion rates directly impact revenue, so it does make sense that more than 50% of companies prioritize A/B testing for CRO.
5.Industries like SaaS, Tech, Retail, and eCommerce where conversions are crucial have the most advanced A/B testing strategies (Speero)
For industries like SaaS, tech, and eCommerce that rely on digital sales and interaction, rigorous A/B testing is no longer a choice. Unlike other B2B companies where sales cycles are longer, these businesses compete in a space where even the smallest of changes can bring in big revenue turnover.
Challenges in running A/B tests
If you think just creating a hypothesis and running the experiment is what A/B testing is, you are wrong. A/B testing is a valuable tool for marketers to understand and optimize for what the audience wants, but it is not a magic wand.
You’re going to run into some challenges, which if not addressed rightfully can hurt your tests.
Statistical significance and sample size
You’re going to hear this term quite a lot. So, let’s simplify it first. Statistical significance means that the results you derive are not by chance. In simpler terms, it means the results you see are real are not by fluke.
If you understand statistical significance, you’ll understand why it is one of the biggest bottlenecks in A/B testing processes. It is super important that your tests reach statistical significance, meaning your results are not random. But it’s not that straightforward. If your sample size is small, you can get false positives or negatives. On the flip side, a sample size that’s too large can make even tiny differences appear large.
Calculating the right sample size will require an expert level of understanding of metrics, sample size, and more and a lot of times, this expertise may be too expensive.
🙂Fun Fact: In an experiment, it was documented that only 20% of tests achieve the 95% statistical significance threshold!
External variables
External factors can impact A/B testing more than you’d like to think. Holidays or even sudden app updates can influence user behavior.
For instance, if you are running a sale during the Christmas holiday, the surge in traffic may not reflect normal user behavior. Or, if you’re launching a new feature during a Black Friday sale, isolating the impact may almost be next to impossible
Implementation errors
A lesser spoken factor, but even best-designed tests can fail if not conducted properly. One single bug, a misplaced line of code, or a flawed randomization process can lead to biased results.
For instance, if your tool does not split the traffic evenly, one variant might get more engagement and conversion, skewing the results completely. Once the tes
Resource constraint
Traditional A/B testing demands a lot of resources–time, experts, money, specialized tools and tech, and of course patience! For smaller teams, this can be an issue.
Resource constraint? Can’t make time for experiments?
Don’t worry. Fibr AI’s smart and super-efficient AI CRO agent Max optimizes all your experiments without breaking your wallet!
Book a demo now!
Ethical concerns
Not much discussed, but A/B testing comes with its own set of ethical concerns.
Manipulating price points to see user reactions, deploying user emotions for profits, or using personal data may sometimes have consequences. It’s important to understand what’s important to your users, the privacy laws of the land, and legalities to avoid ethical issues.
Stages of A/B testing maturity
So far, we’ve covered quite a bit of A/B testing adoption across industries and challenges. Now, let’s study the important stages of A/B testing maturity. The maturity of A/B testing progresses as it moves from simple, basic testing to advanced testing that deploys data and statistical principles deeply.
Below is a basic breakdown for quick understanding:
1.Ad-hoc (basic) testing
Typically, when companies are just getting started with their A/B tests, they are likely to be informal and well, largely unstructured. Teams may run small tests without a schedule or randomly–think changing button colors or headlines.
The tests are based on guesswork and statistical significance is rarely calculated. No attention is paid to sample size, window period, confidence intervals, or any potential biases. You’d note that in such testing, the focus is always on quick wins rather than long-term optimization.
A confidence interval gives you a range in which the true result might actually fall. For instance, instead of saying ‘Variation A can increase conversions by 3%’, the confidence level might say ‘Variation A can increase conversion between 2%-6% with 95% accuracy.’
2.Structured testing
At this stage, the testing gets more structured. A more systematic approach is adopted toward hypothesis formation, defining success metrics, and ensuring there is proper randomization between control and variation groups.
Proper randomization between control and variation groups implies that users are assigned to each group randomly or completely by chance, ensuring results are not biased.
Concepts like confidence intervals, probability, and p-values may be introduced at this stage. However, teams can still struggle with insufficient sample size or worse, misinterpretation of results.
P values basically tell you how likely your test result happened by chance rather than because of a real difference between A and B. A p-value below 0.05 could mean that the change made a real impact. If it’s higher, it might indicate that the difference is random and not worthy of implementation.
The emphasis does shift from intuition to data, but the process may still largely remain manual and reactive.
➕Did you know? A staggering 94% of beginner testers fail to set clear priorities for their experiments!
3.Scaled testing
This is a stage where A/B testing starts showing its magic, becoming a core part of the testing workflow. Teams run several experiments simultaneously–Bayesian method and frequentist method both, invest in advanced tools and techniques to manage and gain a better understanding of statistical significance, p values, and more.
At this stage, the Bayesian method may also step in to challenge the traditional frequentist approach.
Bayesian method does not work with fixed data and updates its conclusions as new data comes in. Conversely, the frequentist method treats data as fixed and makes conclusions based on the experiment data in hand. It also requires a larger sample size and time period to determine if A is better than B.
And while the testing does move to advanced stages, it is not devoid of problems. False positives, failed hypotheses, and sample size issues may still arise, calling for adjustments.
4.Data-driven testing
At this stage, testing is completely data-driven and teams start prioritizing long-term results and impact over short-term gains. Teams religiously gather and use data, statistical significance and even employ the Bayesian method to interpret results.
You’d note here that teams even start accounting for external factors such as seasons, user segmentation, and more to get more actionable results. At this stage, experimentation becomes a strategic tool to grow and propagate business rather than just optimizing random variables.
5.Advanced optimization and testing
This is the final stage in A/B testing and the most mature of all. Here teams deployed the most advanced tools, techniques, and statistics available to achieve meaningful results faster. Think of non-stop optimization, AI-designed systems, strategic and innovative methodologies and ultimately rewriting and challenging traditional A/B testing.
In 2009, Google ran an A/B experiment, testing 41 shades of blue to determine which shade of blue (for their search result link) would bring in maximum user engagement.
After analyzing the result, Google implemented a purplish-blue shade across all its platforms. Result, a tidy $200M in profits!
The example here highlights how companies on higher A/B testing maturity stages have the power to invest in unique experimentations, challenge existing systems, and think of out-of-the-box strategies to boost their earning.
Lessons from top companies using A/B testing
Wondering how Amazon, Google, Netflix, or any other top company in the world conducts A/B testing? Eager to understand what you can replicate from them? Well, below listed are a few pointers that all leading companies across the globe adopt when it comes to A/B testing:
Test for impact, not variables
Often, many teams get stuck with testing superficial changes–swapping images or adjusting font. By a stroke of luck, sometimes such changes do work out. We’re not denying this. But, not always. In fact, a lot of time such testing can waste time, resources, and money without driving any impact whatsoever.
Understand that the real value of A/B testing comes when applied to core offerings, features, pricing, algorithms, systems, backend optimization, and more. The likes of Google or Amazon are not wasting time on elements that cannot bring in conversions and neither should your business.
Remember, top companies always focus their experiments on elements that can shift metrics and not just surface-level tweaks.
Segment your users
A/B testing can widely vary across user segments. Advanced companies do not just look at aggregate numbers; they break them down into device type, location, user behavior, acquisition channel, and more. Why? Because they understand that not all users behave the same way and that what works for one group may not work for another.
But there is a catch: over-segmentation, you lose insights, low segmentations, and key findings are diluted. So, what’s the best approach? Balance. Use segmentation to optimize smartly, and save resources, or else you’ll be left with a pile of data that means nothing.
Measure and work for long-term results
It’s easy to slip and consider microwins as a success. Understand that small wins are good and well but long-term results are what you should be optimizing for. A new pricing range may attract users today but may increase the churn rate in the future. Do you see where we’re pointing at?
Top companies look beyond small-term gains and optimize for long-term retention, revenue impact, and even secondary metrics before rolling out changes.
Don’t stop a few experiments
Arguably the most important pointer you must remember is this–the likes of Amazon, Facebook, Bing, and other top companies do not run one or two A/B tests. They run 100s and 100s of experiments. A/B testing and optimizing is a part of their core system.
These companies and their likes automate entire setups, run experiments every second, and deploy engineers, marketers, and product teams to test their ideas. Further, they understand the value of time and money and would experiment 1000 times before implementing even a simple change.
Remember, one A/B test won’t change a business, but thousands of tests can.
➕Did you know? Microsoft runs more than 1000 A/B tests on Bing search every month!
Let statistical significance be a deciding factor
Guesswork kills good testing and the best of teams and companies understand this. They wait until statistical significance is achieved before drawing any conclusions; and also rely on p-values, confidence levels, and other metrics to understand if a test has yielded anything value.
Don’t be in a rush to analyze results or run experiments. Avoid guessing, let experiments have their run, and then analyze the results thoroughly to derive any conclusions.
A/B testing trends in 2025
Wondering what 2025 holds for A/B testing. You’re not alone. Marketers, A/B, and CRO experts predict some trends for this year, which we list for you below:
1.AI-powered experimentation
The biggest trend following A/B testing from 2024 into 2025 is artificial intelligence (AI).
As AI integrates every aspect of A/B testing, right from hypothesis generation, and sample size estimation to running automated tests, it is further predicted to identify human behaviors and patterns for better refinement, segmentation, and experimentation.
Max, Fibr AI’s top AI CRO agent is pushing all boundaries on AI-based experimentation.
You optimize for every second and without human error!
Don’t miss to check out Max here.
1.Multi-armed bandit gaining traction
Multi-armed bandit. What does that mean? In simpler terms, multi-armed bandits use machine learning and advanced models to analyze data collected and send traffic to the variation that’s performing better. In other words, good variation gets more traffic and the ‘not-so-good’ gets less traffic.
As these advanced models dynamically allocate traffic to the better-performing variant, businesses reduce wasteful spending on other variants. Multi-armed bandits are predicted to become more mainstream in 2025, especially in industries like eCommerce, SaaS, and more.
2.Ethical experimentations
Ethical considerations have taken center stage globally as different countries define their data privacy policies, whether the USA with CCPA (California Consumer Privacy Act) or Europe with GDPR (General Data Protection Regulation).
Companies have started to recognize this and have been making big adjustments to their A/B testing processes to accommodate data privacy laws and not use personal customer information without their complete consent.
Now, the laws regarding data privacy and consumer protection are changing constantly and businesses in 2025 are predicted to work and invest more in experts and tech to ensure proper compliance.
3.Personalization at scale
A/B testing has traditionally always been about finding the ‘best’ option for the majority. But 2025 predictions say otherwise. Personalization, already riding high on the AI and tech advancement wave is projected to grow manifold.
Personalization based on user history, purchase patterns, algorithm search, demographics, and more is going to take center stage. Now, it may require businesses to invest in more sophisticated software but with the promise of significantly higher returns.
How Fibr AI is breaking traditional A/B testing shackles?
For a long time, Fibr AI has been helping businesses of all sizes with their experimentation process successfully. And today with Max, an AI-powered experimentation expert, Fibr AI takes A/B testing to an all-new level.

Want to optimize multiple elements together? Done. Looking for complete automation of traditional A/B testing processes for better efficiency and conversions? Easy. And that’s not it. Max can also help you with hypothesis generation and spot areas for improvement with zero manual effort! Result? More personalization, more conversions, and more experiments.
Want to try out a demo? Book a call with us today and see how your A/B testing processes are reformed and refined forever.