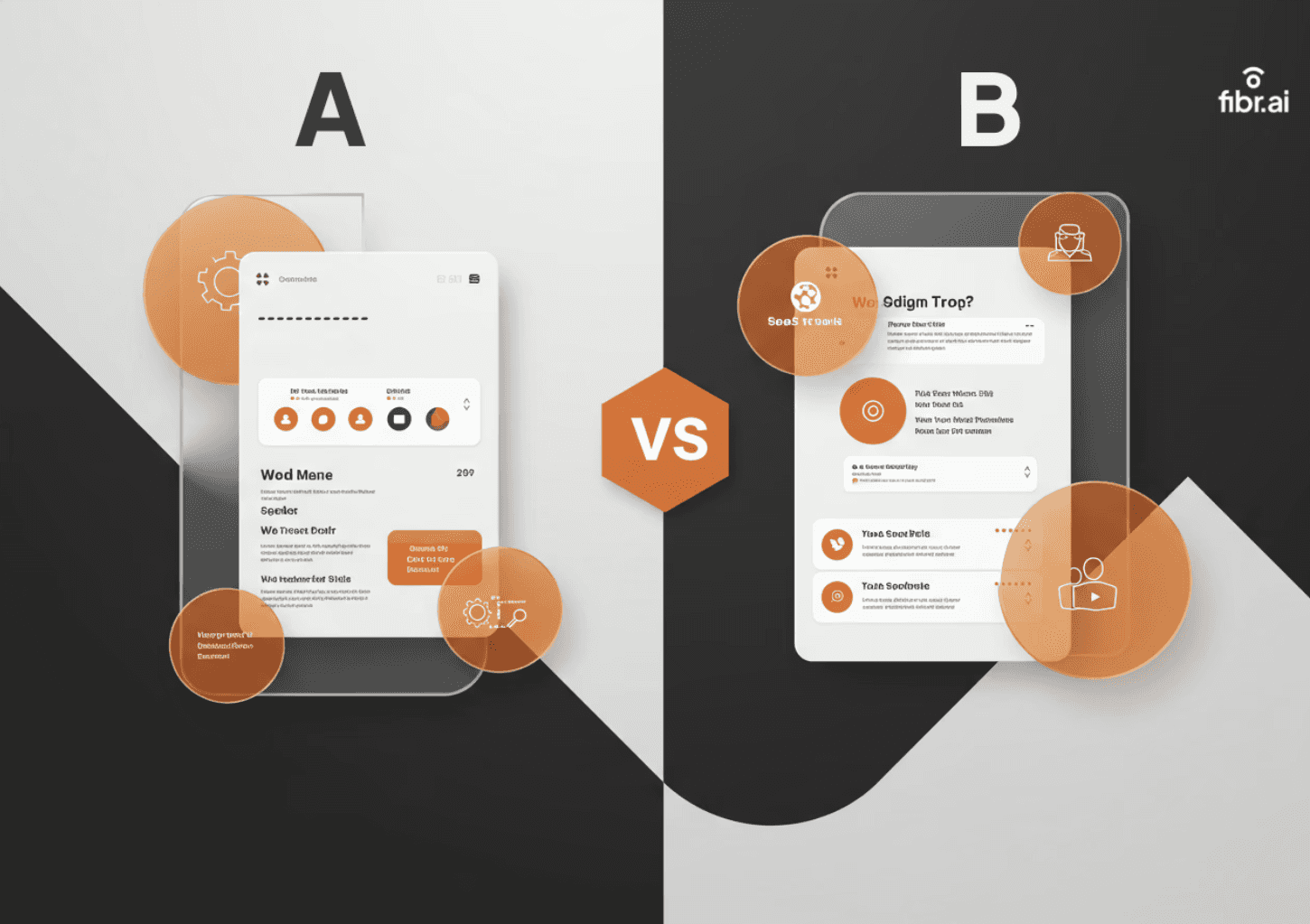

Meenal Chirana
What if you could know the perfect formula for engagement, clicks, and conversions without relying on guesswork? In today’s competitive market space, making data-driven decisions is the difference between a winning campaign and a wasted budget.
This is where AB testing marketing can assist you. It is an essential strategy that allows marketers to test, learn, and optimise their efforts based on real user behaviour. A/B testing, also known as split testing, is the process of comparing two versions of a marketing element.
In this guide, we’ll explore the fundamentals of A/B testing in marketing, how it works, why it matters, and how tools like Fibr AI’s Agent Max can help you test smarter, faster, and more effectively.
What is A/B Testing?
A/B testing, also known as split testing, is a method for comparing two variations of a website, campaign, advertisement, or email campaign to determine which version performs better. This method is widely applied in marketing, user experience (UX) design, and product development to optimise performance and enhance user engagement.
For instance, if you run an online store and are unsure whether a red "Buy Now" button or a blue one will increase sales, you would use A/B testing to show half of your visitors the red button (Version A) and the other half the blue button (Version B).
After collecting enough data, you can analyse which version gets more clicks and ultimately leads to more sales. This removes the guesswork. Instead of making decisions based on intuition, you use real user data to drive improvements.
How A/B Testing Works in Marketing?
Below is the step-by-step process of how AB testing marketing works:
Step 1: Define Clear and Measurable Goals
Before starting an A/B test, clearly define what you want to achieve. A test without a specific goal may provide data, but it won’t lead to actionable insights.
How to set a Goal:
Focus on one primary metric (e.g., conversion rate, click-through rate, email open rate).
Ensure the goal is specific, measurable, achievable, relevant, and time-bound (SMART).
Align the test with business objectives (e.g., increasing sales, improving user engagement, reducing bounce rates).
Example: Instead of a vague goal like “improve engagement,” set a measurable goal such as "Increase email open rates from 20% to 25% by testing subject line variations."
Step 2: Identify the Variable to Test
To accurately determine what influences performance, A/B tests should focus on one variable at a time. Testing multiple elements in a single experiment can lead to inconclusive results because it becomes unclear which change caused the improvement or decline.
Common Elements to Test:
Email Marketing
Subject line wording (e.g., "Exclusive Offer!" vs "Save 20% Today")
Sender name (e.g., "John from XYZ" vs. "XYZ Marketing Team")
Email layout (e.g., single-column vs. multi-column)
CTA button colour and text
Landing Pages
Headline text (e.g., "Limited Time Offer" vs. "Get 50% Off Today")
CTA button colour and placement
Length of the lead capture form (short vs. long)
Use of customer testimonials vs. no testimonials
Digital Ads
Ad copy variations (short and direct vs. detailed description)
Image vs. video-based ads
CTA text variations ("Shop Now" vs. "Get Started")
Targeting different audience segments
Example: If an e-commerce website wants to test both the CTA text and button colour simultaneously, it should run separate tests for each element to isolate its effect on conversions.
Marketers can ensure that test results are accurate and actionable by carefully selecting a single variable. Fibr AI’s Agent Max can simplify and streamline these efforts. It helps you choose the right variable to test, monitors performance in real-time, and provides actionable insights without manual guesswork.
Step 3: Create Two Variations (A and B)
After identifying the variable to test, marketers create two different versions:
Version A (Control): This is the original version that is currently in use.
Version B (Variant): This is the modified version with a single change.
For instance, if testing an email subject line,
Version A might say: "Unlock 20% Off Your Next Purchase!"
While Version B might say: "Your Exclusive 20% Discount Awaits!"
Both versions should be identical in every other aspect, ensuring that only the selected variable differs. This ensures that any change in performance is due to the modified element and not external factors.
Step 4: Determine the Sample Size and Testing Duration
A test needs to reach a statistically significant sample size before conclusions can be drawn. A small sample may produce misleading results due to random fluctuations in user behaviour.
How to Determine Sample Size:
Use A/B testing tools to determine the sample size. A/B testing tools such as Fibr AI offer built-in capabilities to determine the required sample size based on traffic volume and expected impact.
Consider historical conversion rates to estimate the required traffic.
Ensure the sample is representative of your target audience.
Example: If a company wants to test a new homepage design, they should ensure that both versions receive enough visitors (e.g., at least 1,000 users per variant) before analysing results.
Step 5: Randomly Split the Audience
To avoid bias and ensure fair results, the test audience should be randomly assigned to either Version A or Version B. This ensures that external factors like time of day, user demographics, or browsing habits do not influence the outcome.
Best Practices for Audience Splitting:
50/50 Split: Users should be evenly divided to receive each variation.
Use A/B Testing Tools: Platforms like Fibr AI to automate random assignment.
Consistent Testing Conditions: Ensure users in both groups experience the test under similar circumstances.
Example: If testing two different Facebook ad creatives, ensure that both versions are shown to similar audience segments (age, location, interests, etc.) for an accurate comparison.
Step 6: Launch the A/B Test and Collect Data
Once the A/B test is launched, the data-gathering process begins. Marketers must closely monitor the performance of both variations (Version A and Version B) to assess which one delivers better results. This evaluation is based on the Key Performance Indicator (KPI) chosen at the beginning of the test, ensuring that the data collected aligns with the test's overall objective.
Key Metrics to Track Based on Marketing Channel
Email Marketing: Open Rate, Click-Through Rate (CTR), Conversion Rate
Landing Pages: Bounce Rate, Average Time On Page, Form Completion Rate
Digital Ads: Click-Through Rate (CTR), Conversion Cost, ROI
Step 7: Analyse the Results With Statistical Significance
After collecting enough data, marketers need to determine whether Version A or Version B performed better. This analysis helps answer key questions such as:
Which version had a higher conversion rate?
Was the improvement statistically significant?
Are there external factors that could have influenced the results?
Even if one version appears to perform better, don’t assume it’s the best choice until statistical significance is verified. Statistical significance ensures that the observed difference is not due to random chance.
Ways to Check Statistical Significance
Use A/B testing tools like Fibr AI, which calculates significance automatically.
Aim for a 95% confidence level or higher before making a decision.
Avoid "cherry-picking" results – focus on the primary metric defined at the start of the test.
Example: If a new ad variation has a higher click-through rate (CTR) but is not statistically significant, the business should continue testing before rolling it out.
With tools like Fibr AI’s Agent Max, analysing test results becomes even easier. Max not only interprets performance trends for you but also flags when a variation reaches statistical confidence so you can take action quickly and confidently.
It considers multiple variables, detects anomalies, and even alerts you to external influences like time-of-day trends or audience shifts—saving you time while ensuring smarter, data-backed decisions.
If Version B clearly outperforms Version A, it can be confidently rolled out across your campaign. If the results are inconclusive, Agent Max can help you decide what to test next, guiding your optimisation journey step by step.
Step 8: Implement the Winning Version and Keep Testing
Once a winning version is identified, apply it permanently but continue testing other elements for further optimisation. Marketing strategies should always evolve based on new data and changes in user behaviour.
Ways to Continue Optimising
Roll Out the Winning Variation: Apply the successful version site-wide or across campaigns.
Document Insights for Future Tests: Keep a record of what worked and why.
Plan Follow-Up Tests: Test additional variables to refine the strategy further.
Importance of A/B Testing in Marketing
Below are the key reasons why AB testing marketing are as follows:
1. Better User Engagement
AB testing marketing helps improve user engagement by identifying which version of a webpage, app, email, or ad resonates most with your audience. It tracks metrics like clicks, time on the page, and user interactions, enabling data-backed decisions.
Over 77% of businesses worldwide use A/B testing to boost engagement by refining content, layout, and design based on real user behaviour. It also supports personalised experiences by tailoring versions for different audience segments. This leads to higher conversion rates, session duration, and retention.
With Fibr AI’s Agent Max, you can automate these tests across channels and receive real-time insights on what engages different audience segments. Max intelligently adapts testing based on live data, enabling hyper-personalised experiences that result in higher session duration, engagement, and user retention.
2. Improves Conversion Rates
Over 70% of marketers use A/B testing to boost conversion rates, and for good reason. It’s one of the most effective ways to understand what truly drives users to take action, whether it’s making a purchase, signing up, or downloading content.
By testing variations of headlines, CTA buttons, form layouts, images, and pricing, businesses can identify which version delivers the highest conversions. Even small changes, like button colour or CTA text, can lead to significant improvements.
A/B testing removes the guesswork and reveals what actually works, helping eliminate friction points and guiding users toward the desired outcome. Fibr AI’s Max plays a key role here by running automated UX experiments and highlighting friction points based on behavioural patterns.
3. Enhances User Experience (UX)
A well-optimised marketing campaign should align with user preferences and behaviours. AB testing marketing helps improve user experience (UX) by identifying which design, content, or functionality resonates most with the target audience.
A better user experience can increase conversion by 400%. This results in higher engagement, lower bounce rates, and increased customer satisfaction, all of which contribute to long-term business success.
4. Improved User Experience
AB testing marketing significantly enhances user experience by identifying what truly resonates with users through data-driven experimentation. Instead of relying on assumptions, marketers can test variations of design elements, CTAs, headlines, layouts, and navigation.
This enables them to see which version improves engagement, reduces friction, and leads to better outcomes. Studies show that companies that focus on UX see up to a 400% increase in conversion rates, and 88% of users won’t return to a site after a poor experience.
With Agent Max, you can segment your audience and run tests tailored to each group automatically. Max identifies what works best for each user profile and helps personalise pages, emails, and ads on the fly. This ensures every user interaction feels relevant, leading to higher engagement, deeper connections, and improved customer loyalty.
5. Increases Return on Investment (ROI)
A/B testing plays a crucial role in maximising marketing ROI by ensuring that real data back every decision. Instead of investing in ideas based on assumptions, businesses can test and implement only what works best. This minimises wasted spend and boosts efficiency.
For example, testing two versions of a paid ad can reveal which generates more conversions at a lower cost. According to Invesp, companies that use A/B testing see an average 20% increase in ROI.
Moreover, by continuously optimising campaigns through testing, businesses can reduce acquisition costs, improve conversion rates, and get more value from every marketing dollar spent. Fibr AI’s Max makes this process more efficient by automating test cycles, flagging statistically significant results, and recommending the most cost-effective version.
Common use Cases in Digital Marketing
AB testing marketing plays a critical role in enabling teams to optimise their strategies continuously. With Fibminimisesent Max, this process becomes smarter, faster, and fully data-driven. Below are some practical, real-world use cases of A/B testing in digital marketing powered by Max.
1. Hypothesis Generation
Before any test begins, marketers must start with a clear hypothesis. An educated guess about what change might improve performance. This often comes from analysing user behaviour, drop-off points, or underperforming metrics.
Example
If a brand notices a low click-through rate on its landing page, a valid hypothesis might be:
"Changing the call-to-action (CTA) text from ‘Submit’ to ‘Get My Free Guide’ will increase conversions."
A/B testing allows the marketer to test that hypothesis with measurable results. This stage is foundational in ensuring that tests are strategic and focused rather than random.
2. Experimentation in Action
Once a hypothesis is formed, experimentation brings it to life. This is where marketers test different variations of a single element to observe user response. It allows businesses to measure how specific design or content choices affect engagement, conversions, and overall performance.
Examples
Image Testing: Test different product or banner images in ads or on landing pages to see which visual drives more clicks or time on the page. For example, a lifestyle shot vs. a product close-up.
Button Color Testing: Experiment with different button colours (e.g., red vs. green) to identify which one leads to more conversions. For example, test whether a bright-coloured CTA button captures more attention than a neutral-toned one.
Headline Placement: Change the position of a headline, such as at the top of the page vs. below an image to see which layout grabs more attention. For example, moving the product benefit headline above the fold to reduce bounce rate.
This experimentation stage gives marketers concrete evidence of what works and what doesn’t, ensuring campaigns are continuously optimised.
3. Data-Driven Optimisation
After tests are completed, marketers must analyse the data to determine which version performed better based on key performance indicators (KPIs) like click-through rates, conversions, or bounce rates.
This process becomes even more powerful with the help of Fibr AI’s Agent Max. Max automatically tracks performance, highlights statistically significant results, and recommends the best-performing variations in real-time. This enables marketers to implement winning strategies faster and with more confidence.
Example: A company runs a test on two ad creatives and finds that the one with a customer testimonial outperforms the original by 30% in conversions. With Agent Max’s insights, the business can scale that version immediately across platforms for higher ROI.
Types of A/B Testing in Marketing
The types of AB testing marketing are as follows:
1. Multivariate Testing
Multivariate testing (MVT) is a more advanced form of A/B testing that compares multiple variations of multiple elements simultaneously. Instead of testing just one variable, MVT tests different combinations of variables to find the best-performing combination.
When to use
When testing multiple changes at once (e.g., different headlines, images, and button colours on a webpage).
When you want to understand how different elements interact with each other.
When you have high website traffic (since more variations require a larger sample size).
Example
A landing page test includes:
Two headlines ("Get 50% Off" vs "Limited-Time 50% Discount")
Two CTA buttons ("Shop Now" vs. "Claim Your Discount")
Two images (Product Image A vs. Product Image B)
Instead of just two versions (A/B), MVT tests all possible combinations (e.g., Headline 1 + CTA 1 + Image A, Headline 1 + CTA 2 + Image B, etc.), analysing which combination leads to the highest conversions.
2. Split URL Testing
Split URL testing is similar to A/B testing, but instead of changing elements on the same webpage, it compares two entirely different web pages with unique URLs. Visitors are randomly directed to either URL A or URL B, and performance is compared.
When to use
When testing a completely new webpage or design.
When comparing a new website layout vs. the old one.
When testing different funnels or user flows.
Example
An e-commerce store wants to test a new checkout experience:
Version A (Current Checkout Page): www.store.com/checkout-old
Version B (New Checkout Page): www.store.com/checkout-new
Visitors are randomly sent to either page, and metrics such as purchase completion rate and cart abandonment rate are analysed to determine which version performs better.
4. Multi-Page Testing
Multi-page testing (also called funnel testing) involves testing changes across multiple pages within a website or a user journey (e.g., signup flow, checkout process).
When to use
When testing a user journey across multiple pages (e.g., from the homepage to checkout).
When optimising conversion funnels in e-commerce, SaaS, or lead generation.
When trying to reduce drop-offs between steps in a process.
Example
An online subscription service wants to optimise its signup funnel:
Version A: Existing flow (Homepage → Signup Form → Payment Page → Confirmation).
Version B: New flow with fewer steps (Homepage → Signup & Payment on the same page → Confirmation).
By analysing drop-off rates at each stage, the company can determine which flow leads to higher signups.
5. A/A Testing
A/A testing is used to compare two identical versions of a webpage, email, or ad to ensure that testing tools are functioning correctly and to check for random variations in traffic.
When to use
When verifying that an A/B testing platform is working properly.
When ensuring that the audience segmentation is truly random.
When checking for natural fluctuations in data before running an actual A/B test.
Example
A company tests two identical landing pages with no changes. If the results show significant differences, this may indicate errors in the testing tool or traffic distribution, which should be fixed before running an actual A/B test.
Conclusion
A/B testing is no longer an optional marketing strategy. It is a critical tool for success in a competitive digital world. By leveraging AB testing marketing, businesses can optimise their campaigns, improve conversions, enhance user experience, and maximise return on investment (ROI) based on real user data rather than assumptions.
From email marketing and landing page optimization to digital advertising and website UX improvements, A/B testing provides valuable insights that help businesses fine-tune their messaging, design, and targeting strategies. It empowers marketers to make smarter, data-driven decisions, reducing risks and ensuring that every marketing effort is backed by concrete evidence.
If you’re looking to take your A/B testing to the next level, Fibr AI’s Agent Max is here to help! Max is an advanced AI-powered assistant that automates and optimises your A/B tests, ensuring you get faster insights, more accurate results, and higher conversions without the manual hassle.
Smart Test Automation: Set up and analyse A/B tests with ease.
Real-Time Insights: Get instant feedback and recommendations.
Data-Driven Optimisation: Max helps you refine your strategy with AI-powered precision.
Try Fibr AI today and see the difference!
FAQs
1. What is A/B testing in marketing?
A/B testing, also known as split testing, is a method of comparing two versions of a marketing element—such as a web page, email, or ad—to determine which one performs better based on real user data.
2. Why is A/B testing important for marketing?
A/B testing helps marketers make data-driven decisions, optimize user experience, improve conversion rates, and maximize return on investment (ROI) by identifying what works best for their audience.
3. What elements can be tested using A/B testing?
Common elements include:
Email subject lines, sender names, and layouts
Website headlines, CTAs, and images
Landing page designs and form lengths
Digital ad copy, creatives, and audience targeting
4. How do you run an effective A/B test?
To conduct an A/B test:
Define a clear goal (e.g., increase conversions or engagement).
Select a single variable to test.
Create two variations (A - control, B - variation).
Split your audience randomly and evenly.
Run the test for a statistically significant sample size.
Analyze the results and implement the winning version.
5. How long should an A/B test run?
The duration depends on traffic volume and the desired confidence level. A test should run long enough to collect statistically significant data, usually for at least 7–14 days, depending on traffic and engagement levels.