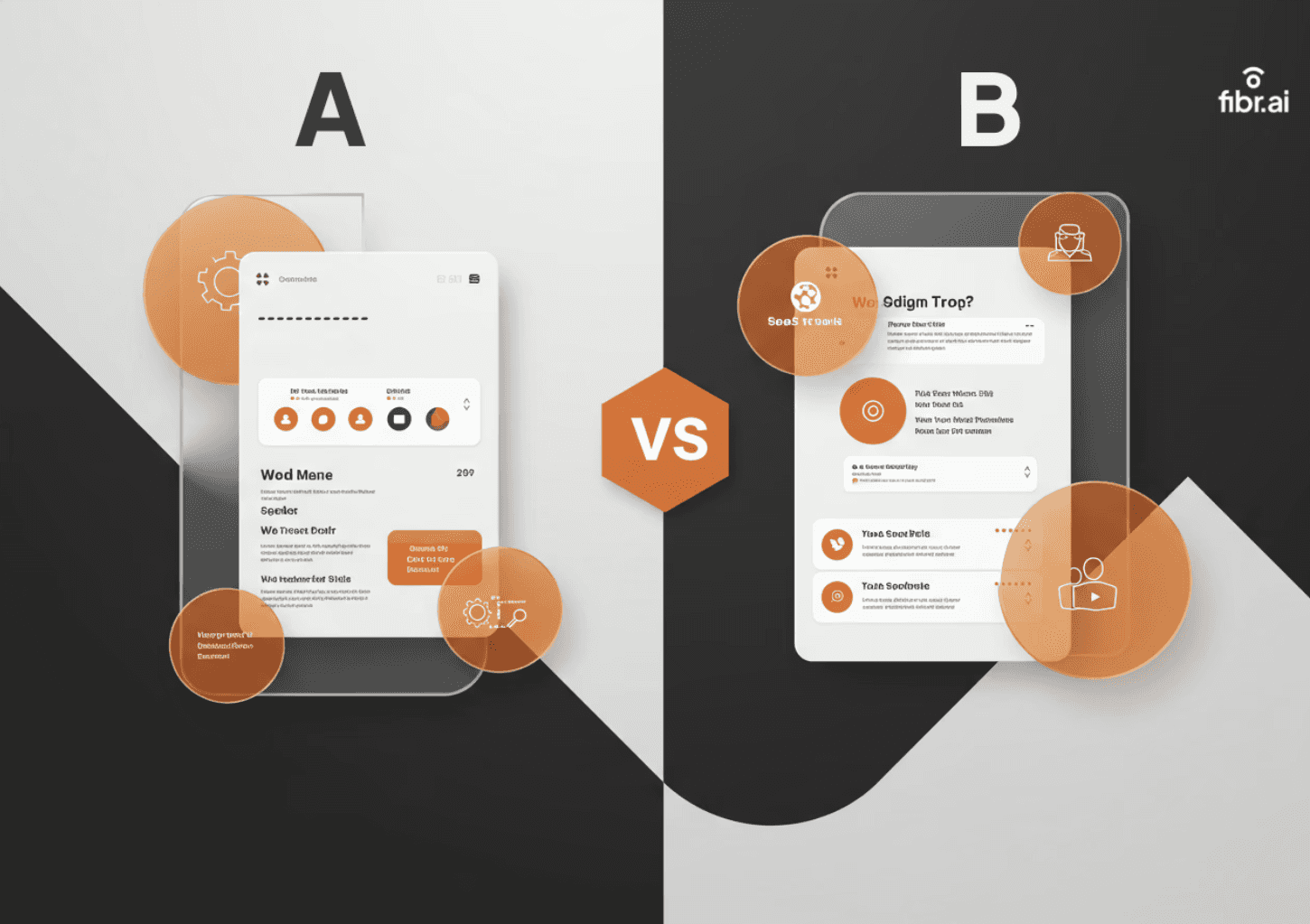
Master A/B testing with 27 actionable tips, best practices, and strategies. Optimize campaigns, improve ROI, and make data-driven decisions.

Pritam Roy
Let's say you're running a marketing campaign.
The goal is simple: boosting conversions.
But when you're targeting millions of users, it can often be difficult to understand what will resonate the most with them. Will a shorter headline work better? Should the CTA button be red or blue?
The details can leave you scratching your head.
And let's be honest, guessing your way to conversions won't cut it here. It can waste resources and cost you valuable leads and revenue.
This is where A/B tests come in. They help you pit two versions of a webpage, ad, or email against each other, letting real user behavior determine the winner. A/B tests empower you to go beyond guesswork and use data-driven insights to pinpoint what works and what doesn't.
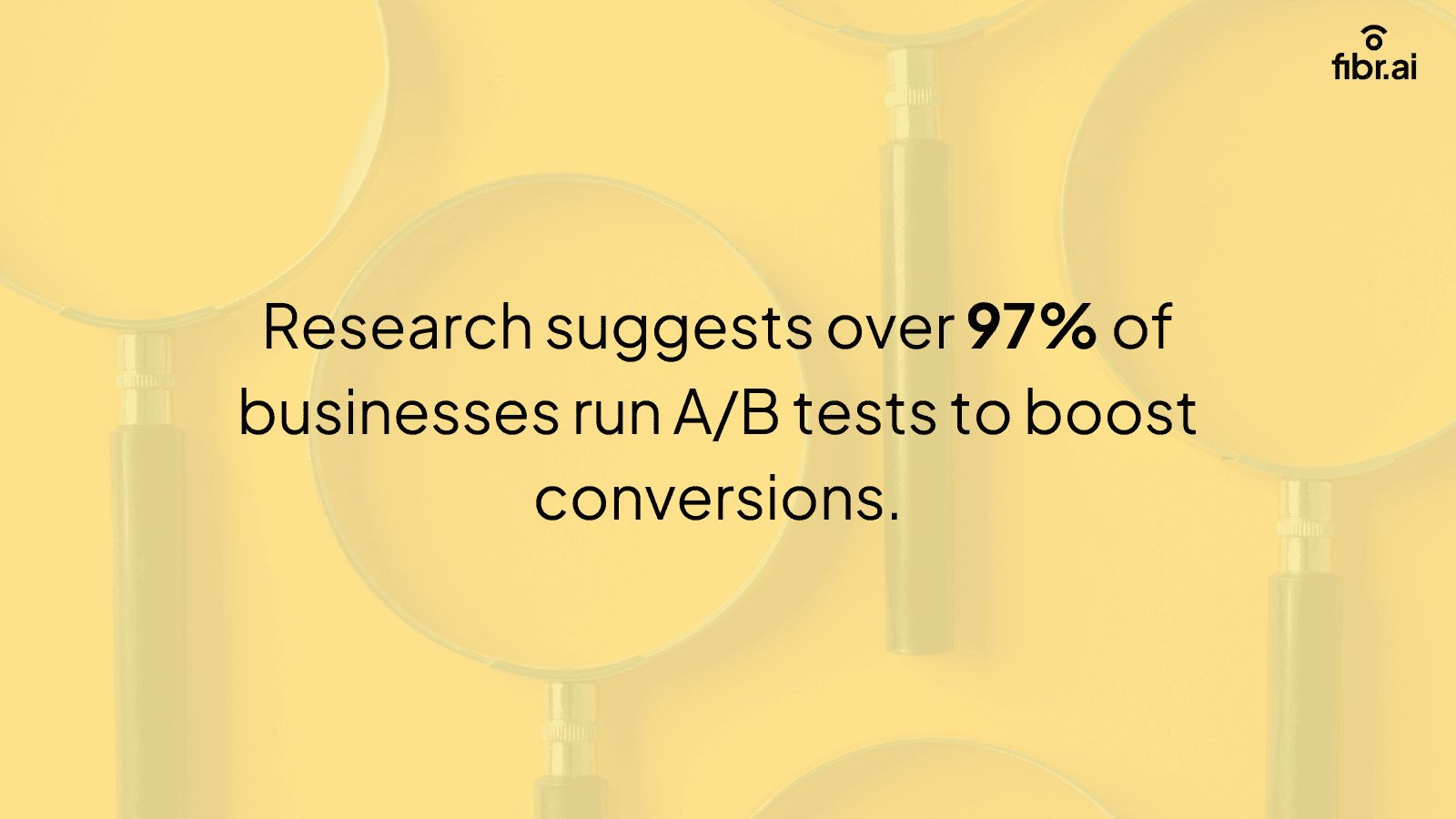
Don't just take our word for it. Research suggests over 97% of businesses run A/B tests to boost conversions.
But there's a catch.
While A/B testing is relatively straightforward, maximizing its impact requires strategy. Defining goals, identifying KPIs, and understanding statistical significance can make the difference between actionable insights and misleading results.
To help you stay on the right side of these tests, we've compiled this comprehensive list of the top A/B testing best practices that will help you drive measurable improvements across campaigns.
Let's dive right in!
27 best practices for A/B testing
Yes, A/B testing is a powerful tool. However, its success hinges on being strategic and following proven best practices. Without a clear roadmap, you can end up with inconclusive results that can lead to misguided decisions. Whether you're testing headlines, layouts, or audience segments, here are 27 A/B test best practices that will help you run successful experiments and drive conversions:
1. Define your goals
It's simple—without clear, measurable goals, you're essentially running experiments without direction. Goals give your test a purpose, helping you optimize for metrics that align with the overall business objectives.
For example, if you're testing a landing page, is the primary goal to boost conversion rates or click-through rates (CTR) or perhaps reduce bounce rates?
Clearly defining these goals will help you prioritize test elements for successful results. Here's how you can do this:
Align goals with business outcomes: For example, if you're running an e-commerce store, your ultimate goal can be increasing purchases. On the other hand, SaaS businesses may want to drive sign-ups.
Be specific and measurable: Instead of aiming for 'improved engagement,' aim for 'increasing CTR by 15% in the current quarter.'
Analyze existing metrics: If your current average bounce rate is 50%, use this as a baseline to set realistic targets.
2. Prioritize what to test
Remember, not everything on your webpage or campaign is worth experimenting with. So, focus on elements that have the maximum impact on your goals. These can be the CTA buttons, headlines, visual elements, etc.
Follow these tips to identify the key elements:
Leverage data-driven insights: Use user behavior tools to identify recurring patterns or common bottlenecks. These tools offer advanced features like heatmaps, click tracking, and funnel analytics, making it easier to understand how users interact with your content. In fact, businesses that leverage customer behavior data enjoy 85% more sales and a 25% increased gross margin.
Gather customer data: Another effective way to identify test-worthy elements is by obtaining direct feedback from customers through surveys or reviews. If customers frequently mention unclear navigation or slow page load times, you know where to focus your efforts.
Analyze industry trends and benchmarks: Look at industry standards for metrics like click-through rates or bounce rates. If your numbers fall behind, prioritize testing elements that influence those KPIs.
3. Create a hypothesis
A hypothesis gives your A/B tests a clear direction. Simply put, it is a data-backed assumption about how a change will affect your key metrics.
With a clear hypothesis, you can prioritize experiments, ensuring optimal resource allocation. A simple way to create compelling hypotheses is by using the "If-Then-Because" approach:
If we make a suggested change,
then we can achieve the desired result,
because the current version has a specific issue.
For example, suppose your website's average bounce rate is 50%, higher than the industry average of 40%. In this situation, your hypothesis might be:
"If we improve the site load speed to under 3 seconds, we can reduce bounce rates by at least 10% because slow load times frustrate users and drive them away."
4. Isolate test variables
Running too many experiments at the same time can cause unnecessary confusion, making it difficult to understand which one actually made the most impact. Therefore, it's important to isolate your variables to ensure accurate, reliable insights.
Here are three simple ways to do this:
Focus on one change at a time. If you're testing a landing page, experiment with just the headline before moving to other elements.
Analyze data to identify what influences user behavior the most. If most users drop off after viewing your CTA, prioritize experimenting with its copy, placement, or color.
Leverage multivariate testing to experiment with multiple variables at once. But remember, it requires a larger audience sample.
5. Define your KPIs
Running A/B tests without defined KPIs is like going on a road trip without a map—you might end up somewhere, but it won't necessarily be where you thought you would. You see, running A/B tests is just half the job done. The other half is measuring the results.
KPIs help you evaluate the results against clear metrics so you can quantify success, identify trends, and make data-driven decisions.
Here are some simple tips for defining KPIs for A/B testing:
Align KPIs with goals: If your goal is to increase conversions, track metrics like conversion rate, click-through rate, or cost per acquisition.
Be specific: Avoid vague metrics like "better performance." Instead, aim for quantifiable results like a 15% increase in product purchases.
6. Segment your audience
Not all visitors to your website will behave the same way. Segmentation helps you divide your audience into smaller groups based on shared characteristics—like demographics, behavior, or purchase history—for more targeted and meaningful A/B tests.
Segmentation also helps you hyper-personalize your campaigns, boosting ROI and increasing conversions by up to 50%.
By segmenting your audience, you can:
Test different elements or offers for different personas.
Experiment with region-based messaging.
Address common pain points for different segments like first-time visitors, long-time subscribers, etc.
If you have a large user base, remember not to get carried away. Prioritize segments that significantly impact your business goals, like high-value customers or users who abandoned their carts.
Inline CTA:
Optimize your A/B tests with Fibr AI
Ensure consistency between your ad copy and landing pages while leveraging AI-driven personalization.
Get started today!
7. Select your sample carefully
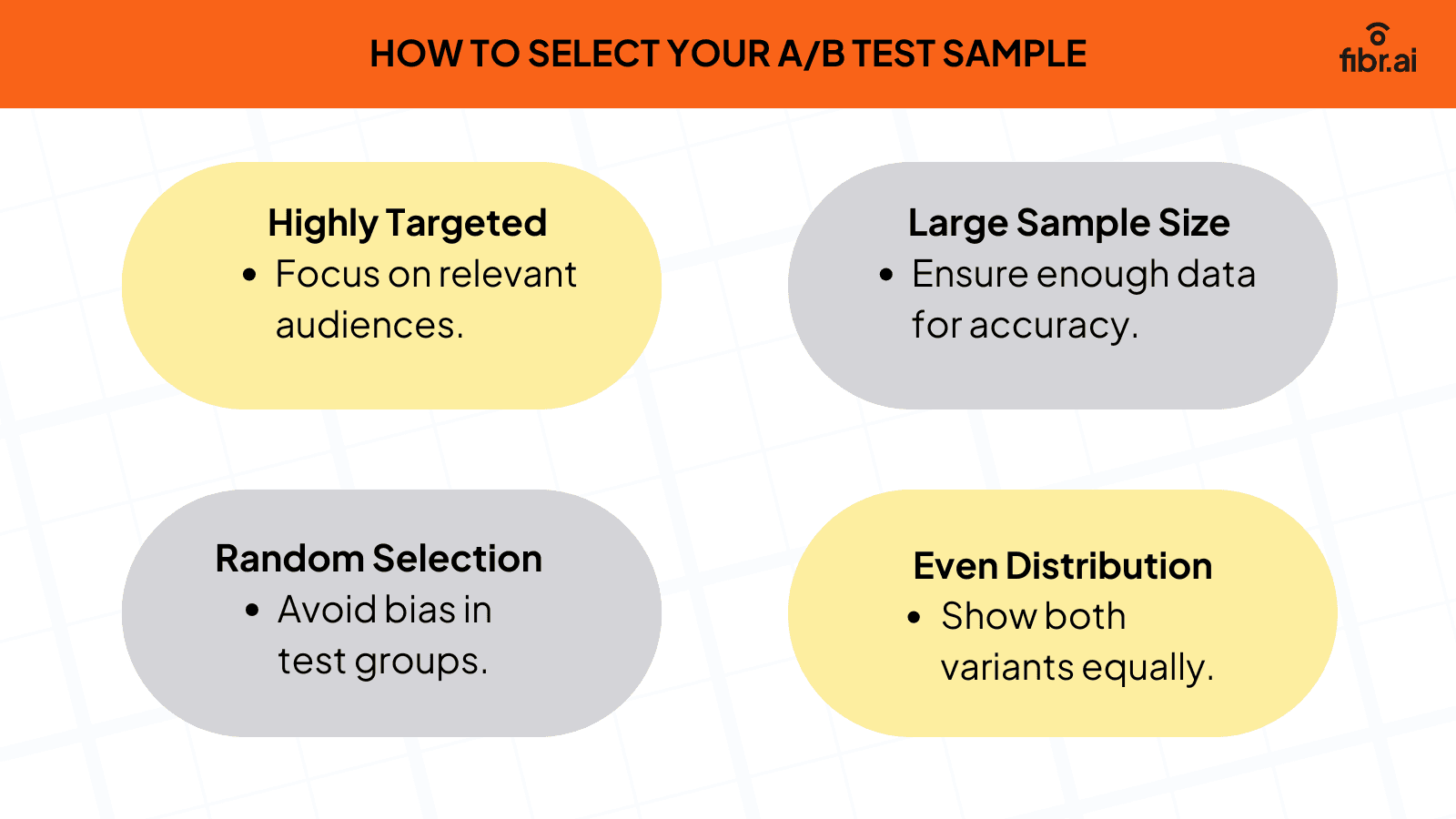
Selecting the right sample for your A/B test is crucial to obtaining reliable and actionable results. Here's a handy guide to doing this:
Highly targeted: Focus on selecting the most relevant audience for your test. Instead of testing broad, generalized groups, select a segment that aligns with your ideal customer.
Large sample size: A large dataset reduces the chances of random error, ensuring your conclusions are based on real trends rather than anomalies.
Random: Make sure your sample is randomly selected to avoid bias. This will give you a more accurate representation of customer behavior.
Even distribution: Make sure both variations of the test are shown to an equal number of unique users. If one variant is shown to more people than the other, your results will be biased.
8. Outline your sample size and test duration
Before you run A/B tests, make sure you have a large enough sample size. This will ensure the results reflect the true behavior of your audience, not just a few outliers. Drawing conclusions from a small sample size can lead to Type I or Type II errors—false positives or negatives.
As a rule of thumb, the larger your sample, the more reliable your findings.
But how long should you run a test to ensure reliable results? At least till you reach 25,000 visitors.
Ben Heath, ads expert at Facebook, explains, "For me, the appropriate length of time to assess a new Facebook Ad or Instagram Ad is about three to seven days. That will vary a lot depending on how many conversions you're generating through that ad. The more conversions, the faster you can make a decision."
This will help you minimize variability and ensure your findings are statistically significant.
Which brings us to the next best practice.
9. Understand statistical significance
Understanding statistical significance is one of the most important A/B testing tips. Without it, you risk incorrect decision-making based on random data fluctuations. Simply put, statistical significance helps you determine if your test results are due to the changes you made or just random chance.
The statistical significance of your experiments is calculated using a p-value that shows the probability of an outcome being a result of chance.
Therefore, when running A/B tests, aim for a p-value of less than 0.05. This means there's less than a 5% chance that the results you're seeing are due to random variation. In other words, you can be 95% sure your results are accurate.
Pro tip: Use a statistical significance calculator to avoid complex manual calculations and generate instant results.
10. Create technically identical variations
Another important tip when running A/B tests is to create technically identical test variations. This will ensure the results are only influenced by the changes you're testing, not other factors.
For example, if you're experimenting with a landing page, create a duplicate version with all the identical elements. Now, if you want to experiment with the CTA, layout, or images, make the changes only on the duplicate page and keep the original unchanged.
Remember not to make changes in other aspects, such as the server location, site speed, etc. This will help you better understand the impact of different variables and obtain valuable insights.
11. Monitor data in real-time
Monitoring your data in real-time is an essential A/B testing best practice as it helps you identify and address issues promptly. For example, technical issues or unexpected performance drops. Detecting these problems early can:
Prevent you from making inaccurate decisions based on faulty data
Maintain test integrity
Keep an eye on bounce rates, load times, or conversion trends
Generate accurate insights that reflect genuine user behavior
Analyze funnel performance for different versions
12. Run tests for the full duration
One of the biggest mistakes that you can make while running A/B tests is ending them prematurely. While a quick 80% variation may seem like a win, it often reflects random noise rather than true performance changes.
"Here's a common scenario, even for companies that test a lot: They run one test after another for 12 months, declare a bunch of winners, and roll them out. A year later, the conversion rate of their site is the same as it was when they started. Happens all the damn time. Why? Because tests are called too early and/or sample sizes are too small," explains CRO expert Peep Laja.
He suggests stopping an A/B test only when:
You have enough data to make a call.
You're taking a convenient sample, not a representative sample if you're stopping the test within a few days.
You get a statistical significance of at least 95%.
13. Understand the collected data
So you've followed all best practices, run a couple of tests, and collected data. What's next? Interpreting it correctly to determine if the tests were successful. Without a proper understanding of the collected data, you risk implementing changes based on inaccurate assumptions.
For example, if a variation shows a 1% increase in conversions, it might be statistically significant but adds no value if it doesn't significantly impact revenue.
Let's look at an example to understand this further. If a new checkout button color on your e-commerce site increases conversions by 1%, it may be statistically significant. However, if it translates to negligible revenue growth—say $100 on a million-dollar baseline—it might not justify the costs.
Therefore, it's important to analyze data based on not only the hypothesis but also on the impact it has on your overall business goals.
14. Share results with your team
A/B testing is a collaborative effort that can drive business growth. As such, sharing test results with the team is important to:
Ensure everyone is on the same page
Foster a culture of data-driven decision-making
Enable everyone to chip in their ideas and opinions
For example, suppose a landing page redesign increased conversions by 25%. Sharing the results with your team will help product developers understand user preferences and guide content creators on messaging strategies.
15. Keep an open mind
A/B testing often challenges preconceived notions. This means even if you think you have a solid hypothesis, the results may differ. Or maybe you didn't have a solid hypothesis to begin with. It's okay, we won't judge.
You see, pre-existing biases can skew how you interpret results. For example, a headline variation you assumed would perform better might underperform, while an unconventional design could surprisingly drive higher engagement.
Therefore, it's important to keep an open mind and understand that user preferences can be dynamic.
Make sure to:
Start tests without emotional attachment to one variation.
Avoid cherry-picking results to fit expectations.
Treat conflicting results as opportunities to learn and refine tests.
16. Always check for factors that may skew results
Inaccurate A/B test results can lead to poor decision-making, costing you valuable time, resources, and money. According to Gartner research, businesses lose a whopping $15 million every year due to poor data quality.
For example, a sudden spike in traffic from a one-time campaign, like a holiday sale or viral post, can overinflate performance metrics. To avoid making important decisions on this information, always check if the results can be skewed by:
Paid ads
Seasonal trends
Referral traffic
Site updates, etc.
17. Implement a phased rollout for changes
Once you're satisfied with your test results, it's time to implement the changes across your user base. The best way to do this is by implementing a phased rollout. It helps you make the changes slowly, ensuring no potential issues disrupt the entire system and reducing the strain on your team and tech infrastructure.
For example, let's say you've tested a new website navigation design that increased conversions by 15% during testing. Before applying it to all users, deploy it to 10% of your audience first. During the rollout phase, monitor key metrics like:
Bounce rates
Time on site
Conversions, etc.
This will help you identify potential technical or performance issues that may not have appeared during testing.
18. Remove invalid traffic for better accuracy
Accuracy is the most important factor for running successful A/B tests. However, invalid traffic can significantly skew your results.
Research reveals that nearly 70% of respondents face fake or spam leads from their paid media campaigns. These leads often originate from bots or non-human traffic that interact with your ads—clicking them but offering no genuine engagement or conversion.
As such, it becomes extremely important to take steps to remove or filter out invalid traffic. Some effective methods include:
Using bot protection tools
Leveraging traffic filtering features offered by platforms like Google Analytics
This will help you identify and eliminate invalid traffic, improving the reliability of your insights.
19. Keep testing
A/B testing is not a one-and-done activity. It is an ongoing process that you must continue despite finding successful variations. By constantly testing new ideas, you can:
Improve user experience
Enhance engagement, and
Boost conversion rates
Remember that just because you achieve success with one set of tests, it doesn't mean there isn't room for further improvements. New industry trends, shifting customer preferences, and evolving technologies constantly create new opportunities.
This means an A/B test that performs well today may not remain as relevant a few months from now.
20. Document results and follow-ups
One of the most critical A/B testing best practices is documenting test variations, results, and follow-up actions. This will help you create a knowledge base to guide future decisions and improve your marketing strategy over time. It also helps:
Track performance over time
Identify patterns and trends
Avoid repeating mistakes and leverage successful strategies
For example, if a variation shows that a specific CTA consistently outperforms others across different campaigns, documenting this result can help you apply it to other aspects of your marketing and optimize your efforts.
Inline CTA:
Take the guesswork out of personalization
Align your landing page messaging with user expectations and deliver experiences that convert.
Try for free today!
21. Ignore daily data
When running A/B tests, it's easy to get caught up in the day-to-day fluctuations of your data. However, daily data can be misleading.
Small changes, such as a sudden drop in conversions or a spike in website traffic, could just be random blips and don't indicate any meaningful patterns. So, instead of obsessing over daily data, focus on the long-term trends that will give you a clearer picture of your test's performance.
22. Make changes only after the test ends
We know it can be tempting to make changes during the test if one variation seems to be outperforming the other. But remember, patience is key. Interrupting the test early can lead to inaccurate conclusions and poor decision-making. You see, early results may fluctuate due to random variance or external factors. Making premature changes can lead to biases and misinterpretations.
So, let your tests run their full course. This will give the data enough time to stabilize and become reliable, ensuring the changes you make are based on solid evidence, not short-term fluctuations.
23. Don't stop at just one test
It's tempting to consider one A/B test as the final answer to your questions. However, running the same test again with the same variables can give you more accurate, reliable results.
But why isn't one test enough? Because the outcomes can fluctuate due to various factors, such as:
Seasonality
Traffic changes or
Random trends
Repeating tests eliminates this risk, ensuring consistency. Moreover, multiple tests can uncover insights that a single test may overlook. For example, maybe the change only works for a certain segment of your audience, or the effect is noticeable during specific times of the day. Repeating the test gives you a clearer picture and makes your conclusions robust.
24. Avoid common mistakes
While there's no one-size-fits-all approach to A/B testing, there are definitely some common pitfalls every marketer must avoid at all costs:
Insufficient sample size: Testing on a small group can lead to false results, as it doesn't capture diverse user behavior.
Testing multiple variables simultaneously: Testing several changes at once makes it difficult to determine what impacted performance.
Ignoring external factors: Seasonal trends, promotions, and market fluctuations can skew results.
Stopping tests too early: Ending a test prematurely can prevent you from reaching statistical significance.
Avoiding these pitfalls can help you run more effective and reliable A/B tests and generate actionable insights that drive success.
25. Ask users for feedback
One of the most powerful ways to enhance your A/B testing process is to directly ask users for their feedback. This can provide you with qualitative insights that complement the quantitative data from your tests. Here's how:
Use post-test surveys to ask users for their opinions to understand why a particular variation performed better.
Conduct one-on-one interviews to better understand user experiences and identify pain points or elements that weren't obvious from the data alone.
Use on-page feedback widgets to capture real-time user input.
Instead of asking broad questions like "Was this useful?", ask targeted questions such as, "Did the new checkout flow improve your purchase experience?"
26. Start A/B testing early
Running A/B tests early in your marketing or product cycle can help you optimize your strategy before you invest too many resources. It helps you:
Prevent potential problems early, avoiding larger issues down the line.
Make informed, data-driven decisions right from the beginning.
Create a cycle of improvement, where each test informs the next.
Save time and resources on strategies or designs that might be less effective.
For example, suppose you run an e-commerce business. Running A/B tests during the website design phase can help you maximize your ROI and ensure your strategies align with user expectations.
27. Optimize winning versions
The last but most important A/B test best practice is to optimize and scale the winning version. This means refining your best-performing version and using the insights to optimize other areas of your site.
Here's why this is important:
Maximize impact: The winning variation reflects the most effective change. By optimizing it further, you can capitalize on the improvements and maximize your results.
Consistency across touchpoints: When you find success with one element, roll it out across all relevant touchpoints to deliver a consistent user experience.
Expand testing: For example, you might have tested a headline, but now that you have a winning headline, you can test other elements such as CTAs, images, or layouts.
Now that we've seen the top A/B testing tips and best practices, let's look at how you can implement them in different phases.
A/B testing best practices for different phases
1. Planning phase
This phase focuses on setting a strong foundation for your A/B test to ensure reliable and actionable results. Here are some key best practices for this phase:
Define your goals
Create a hypothesis
Figure out what to test
Isolate test variables
Define your KPIs
Segment your audience
Outline sample size and test duration
Understand statistical significance
2. Implementation phase
Follow these A/B testing best practices in this stage to ensure smooth execution and data accuracy:
Create technically identical test variations
Monitor data in real-time
Run tests for the full duration
Keep testing
Ignore daily data
3. Post-test analysis
This phase focuses on extracting and implementing insights. Key best practices include:
Understanding the collected data
Sharing results with your team
Documenting results and follow-ups
Implementing a phased rollout
Optimizing winning versions
Conclusion
A/B testing is a powerful tool for data-driven decision-making. However, it's important to take a structured approach and follow industry best practices for accurate results.
One important aspect that you can't miss while A/B testing is personalization. With AI-powered tools like Fibr AI, you can personalize your landing pages at scale, ensuring they mirror the ad copy. What's more? You can personalize both text and images to ensure your message resonates with users.
Leave the hard work to Fibr AI while you focus on what matters most—crafting exceptional strategies and building meaningful connections with your audience.
FAQs
1. What are A/B tests?
A/B testing is a method of comparing two versions of a webpage, email, or other content to determine which performs better.
2. How to run A/B tests?
To run A/B tests, you need to:
Define your goals
Create variations
Select relevant KPIs
Segment your audience
Run the test
Analyze results
Implement changes
3. What is the role of A/B testing?
A/B testing helps marketers:
Improve user experience
Increase ROI
Deliver personalized experiences
Make data-driven decisions