A/B Testing
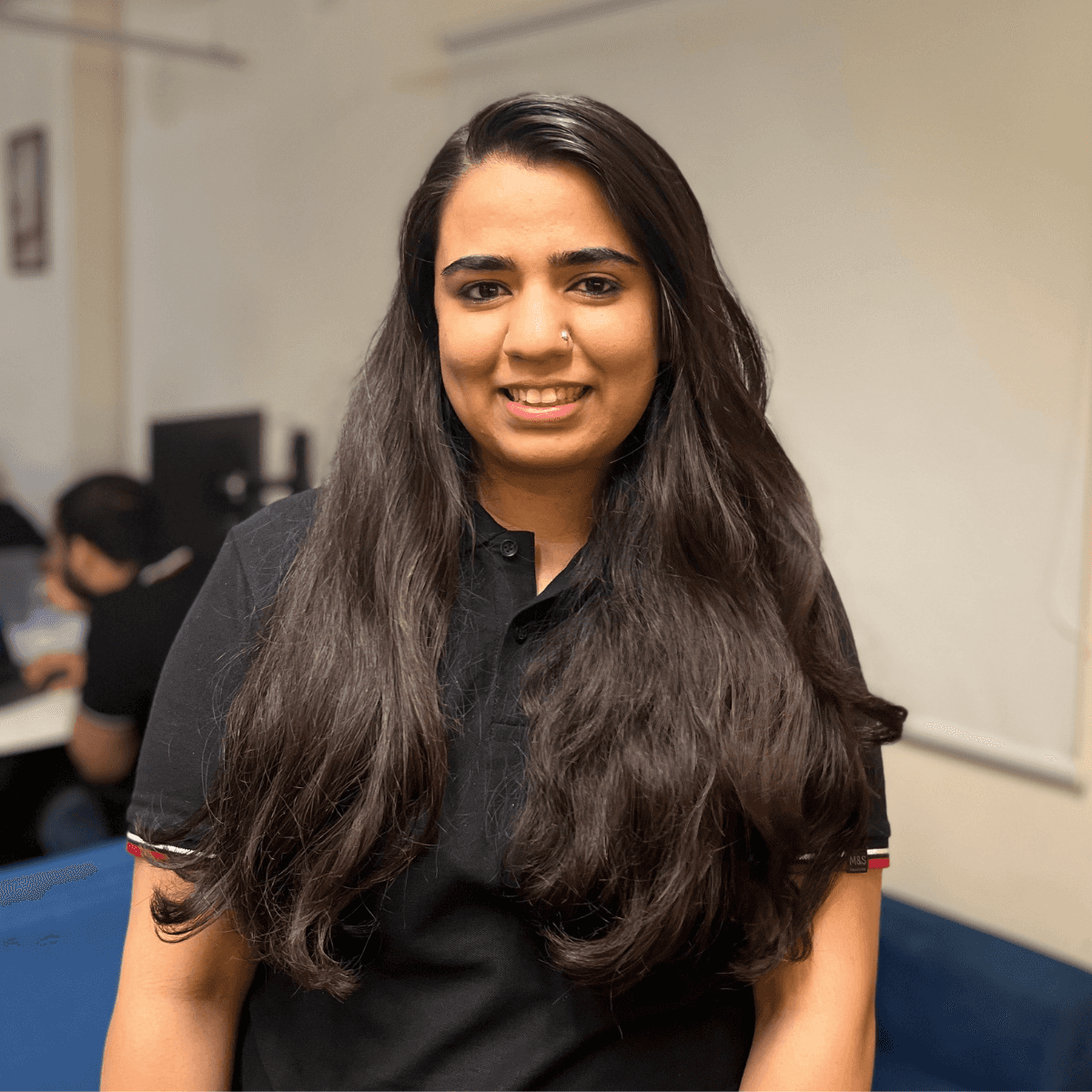
Meenal Chirana
You’re confused, right?
Which one should you use between A/B and multivariate testing? These are the tricky questions that plague CRO professionals and marketers during conversion rate optimization.
While both AB and multivariate testing can help you make data-driven decisions, there are district differences in their approaches and what the tests tell you.
In this guide, we’re going to break down each method, exploring the key differences as well as testing processes. We’ll also look into their pros and cons, when and how to conduct both tests.
After reading this guide, you’ll be able to know which test to use in your conversion rate optimization strategy.
A Quick Summary: Multivariate Testing VS A/B Testing
A/B testing is ideal when determining the effective version of a webpage and is used on a low-traffic site.
On the other hand, multivariate testing is ideal for analyzing complex interactions between variables and is used on a high-traffic site.
Here’s a clear and concise table differentiating multivariate testing and A/B testing:
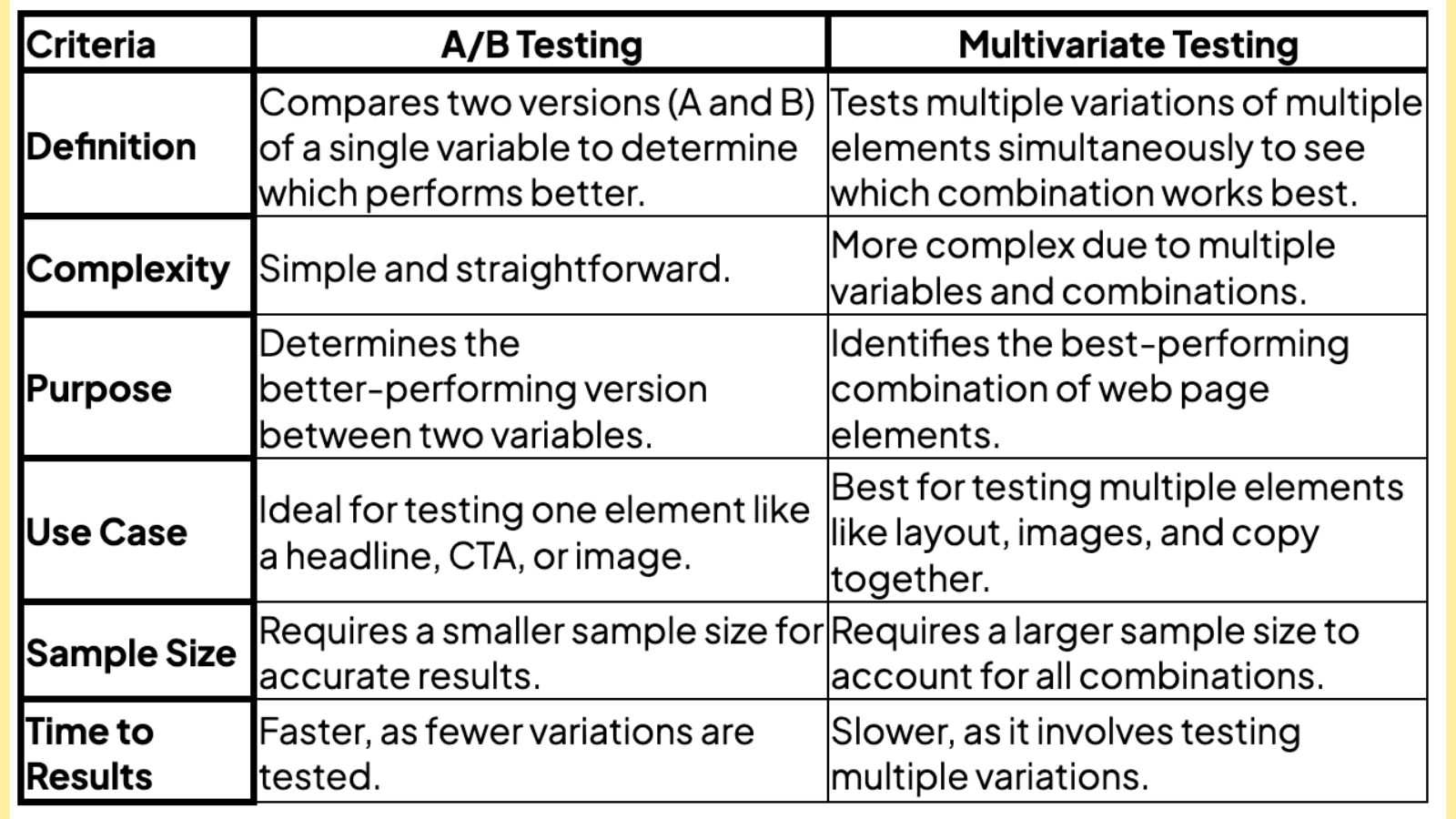
That said, let’s get the multivariate testing vs A/B testing basics straight first before we proceed.
What Is AB Testing?
A/B testing, also known as split testing, is a conversion rate optimization strategy that involves comparing two versions/variations of a webpage (A and B) to determine which performs better in achieving specific goals, like increasing conversions.
It works by showing two variants of a page to a random audience segment and analyzing performance metrics to determine which variation achieves better results for your conversion goals.
For instance, after comparing two variations of their homepage’s CTA button “Sign up for free” versus “Trial for free”., Going noticed that the second variation with the “Trial for free” CTA increased trial signups by 104%.
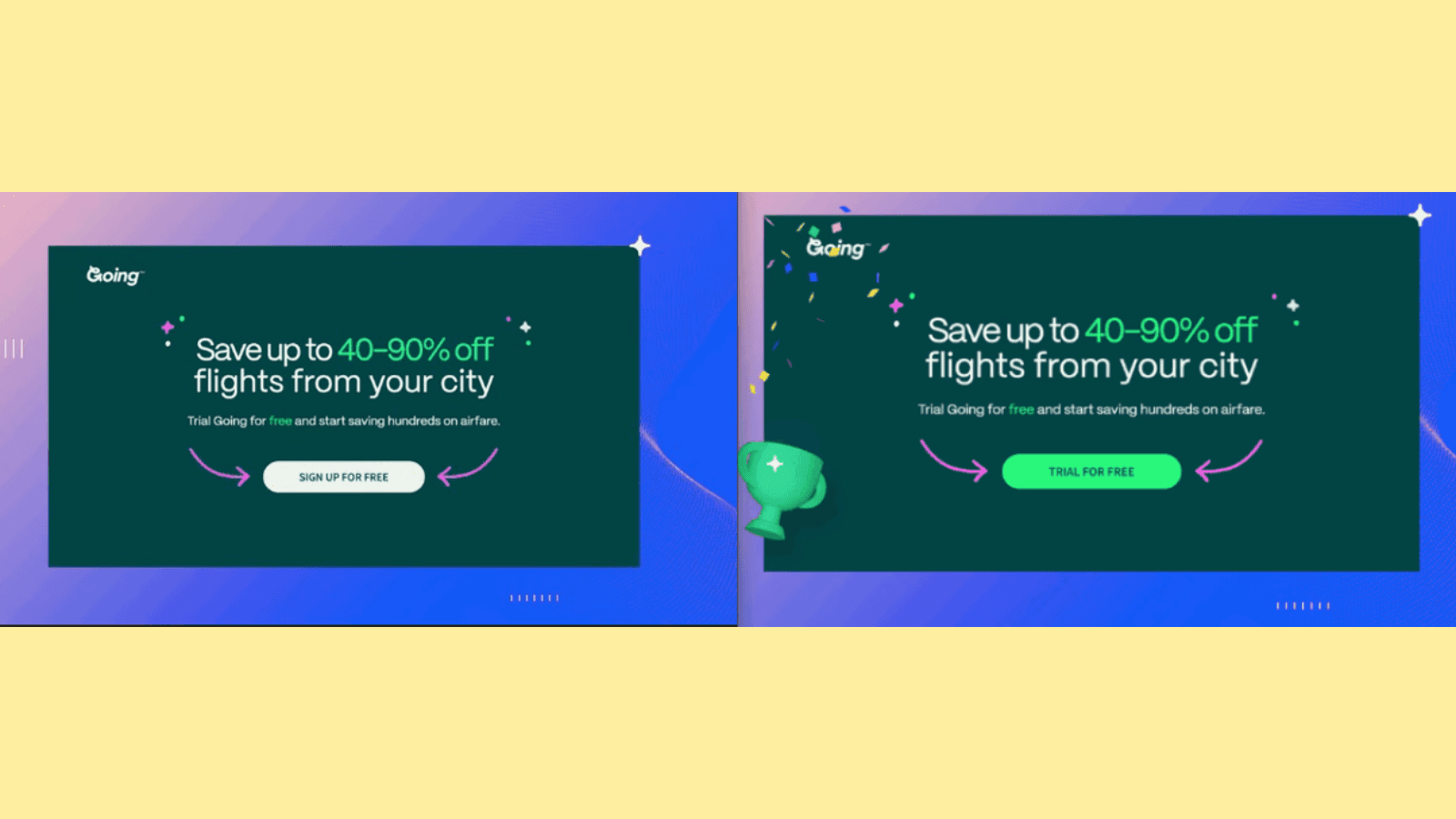
Source: Unbounce
For CRO (conversion rate optimization), A/B testing identifies which design, content, or feature changes drive better results. Key aspects include testing one variable at a time, tracking data accurately, and running tests on significant traffic to ensure reliable results. This approach enables data-driven decisions to improve user experience and business outcomes.
Want to take your conversion rate optimization game to the next level? Partner with a reliable CRO agency like Fibr to maximize conversions.
What Is Multivariate Testing?
Multivariate testing, also called multivariate or multivariable testing, is a method of evaluating multiple elements on a webpage simultaneously to determine which combination delivers the best performance.
Unlike A/B testing, which compares two versions, multi variant testing experiments with multiple variations of elements like headlines, images, layouts, or call-to-action buttons.
Like this:
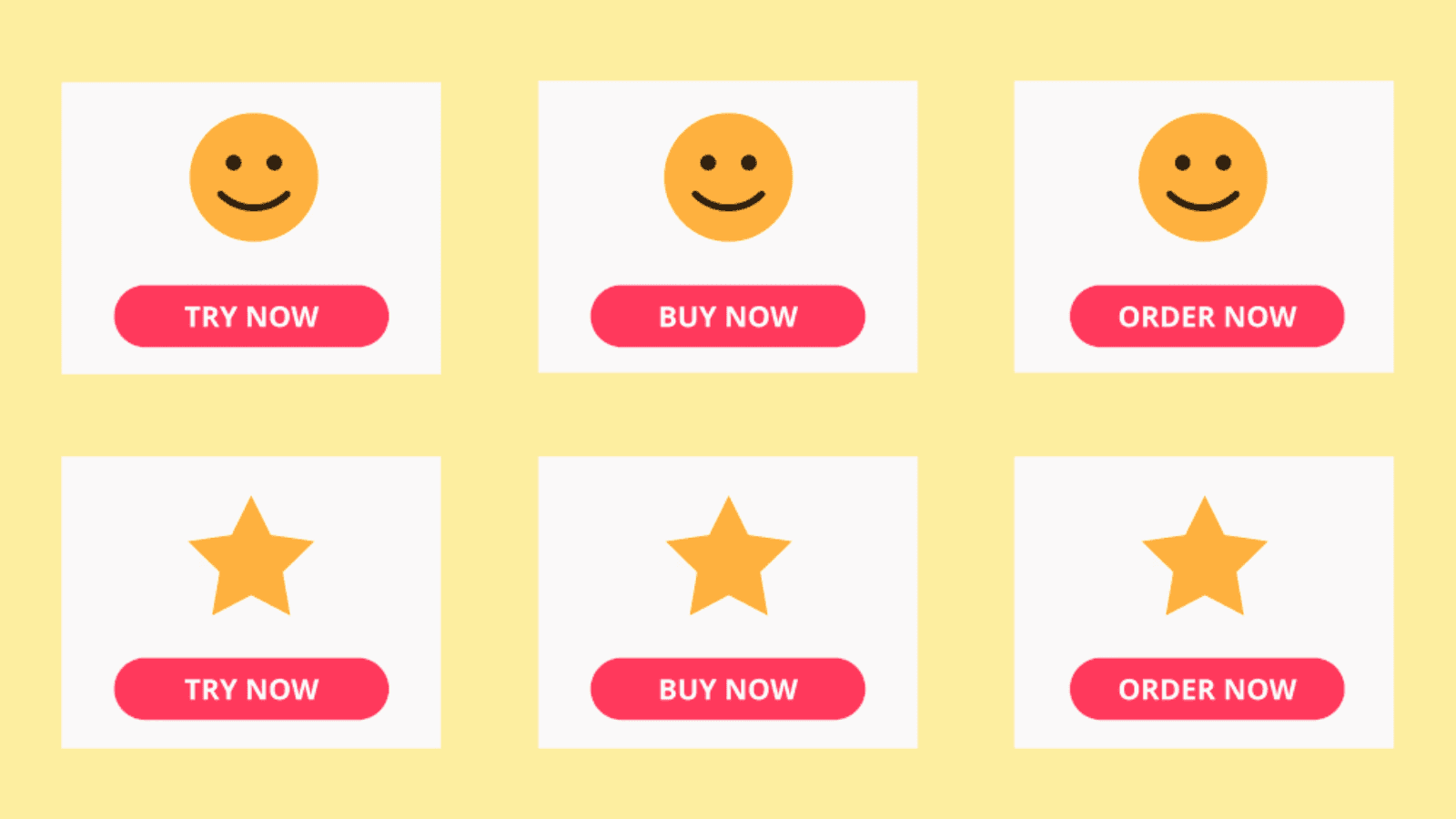
Multivariate tests help CROs and marketers understand how different elements work together to improve conversions. For example, a multivariate testing project could involve testing three headlines with two images, creating six unique combinations.
Multivariate testing provides data-driven insights to enhance user experience and maximize website effectiveness.
What are the Differences Between AB Testing & Multivariate Testing?
A/B and multivariate testing are both effective conversion rate optimization (CRO) tactics. However, the two differ based on the number of variants, purpose, complexity, traffic requirements, test duration, insights, and use cases.
Let’s take an in-depth look into the differences between A/B and multivariate testing.
1. Number of Variants
In terms of variables, A/B testing compares two or more variations (e.g., Version A vs. Version B) of a single variable, such as a headline, button, or image, to determine which version performs better.
For example, AB testing multiple variants might involve testing two call-to-action buttons with different colors to see which one drives more clicks. The test isolates one element to provide clear, actionable insights into how that specific change impacts user behavior. This simplicity makes A/B testing a preferred choice for straightforward optimization tasks that focus on improving a specific metric.
On the other hand, multivariate testing, or MV testing evaluates multiple elements on a webpage simultaneously. Unlike A/B testing, MVT assesses the performance of combinations of elements, such as headlines, images, and buttons, to understand how they interact.
For instance, multivariate tests can determine how a particular headline and image pairing impact conversions. While MVT provides deeper insights into user preferences and element interactions, it also requires more complex tools and advanced analytical capabilities to interpret the results effectively, making it suitable for detailed testing scenarios.
2. Purpose
A/B and multivariate testing also differ based on the purpose you want to achieve.
Here is how:
The primary purpose of A/B testing is to identify which version of a single element or page performs better overall. It’s a simple yet powerful approach to improving specific metrics like click-through rates or conversions.
A/B multivariate testing focuses on providing clear insights by testing one change at a time, allowing marketers to measure the direct impact of that modification.
For example, in AB testing multiple variants of a headline, the goal is to determine which version drives more clicks or user engagement. This clarity makes A/B testing ideal for targeted optimization efforts where you need quick and actionable insights.
Conversely, multivariate testing is used to understand how multiple variables interact to produce the best results. Rather than isolating one change, MV testing examines the combined impact of various elements, such as a headline, image, and button.
For instance, a multivariable test could involve testing different layouts to find the combination that leads to higher conversions. This method is ideal for more complex optimization goals, offering a deeper understanding of how multiple changes influence user behavior on a webpage.
3. Complexity
The two conversion rate optimization tactics also differ in complexity.
A/B testing is relatively straightforward, making it easier to implement and analyze. Focusing on one variable at a time, allows marketers to gain clear, actionable insights without requiring advanced tools or expertise.
For example, an AB test of multiple variants might involve comparing two different designs for a call-to-action button, ensuring the results are easy to interpret. This simplicity is why A/B testing is often recommended for those new to conversion rate optimization or for websites with limited resources.
In contrast, multivariant testing is more complex due to its multi-variable approach. MVT analyzes multiple elements simultaneously and evaluates their interactions, requiring advanced tools to manage and interpret the results.
For example, multi-variable testing might assess how a new headline, a different image, and a revised button text work together to boost conversions.
The complexity of multivariate testing means it’s better suited for larger websites or projects with high traffic, where the goal is to uncover detailed insights into how various design elements influence overall performance.
4. Traffic Requirements
Another aspect where A/B testing and multi-variate testing differ is the amount of traffic required.
Let’s see the difference.
A/B testing requires less traffic, making it accessible for smaller websites or campaigns with limited visitors. By splitting the audience between two or more versions of a single variable, such as a headline, AB testing can achieve statistically significant results without needing a massive audience.
For marketers or businesses working on modest scales, A/B testing offers a practical way to gather actionable insights efficiently.
Contrarily, multivariate testing, or multivariant testing, demands significantly more traffic because it involves testing numerous combinations of variables.
For example, a multivariate test might examine how three different headlines, two images, and two button styles interact, resulting in multiple combinations to test.
Like this:
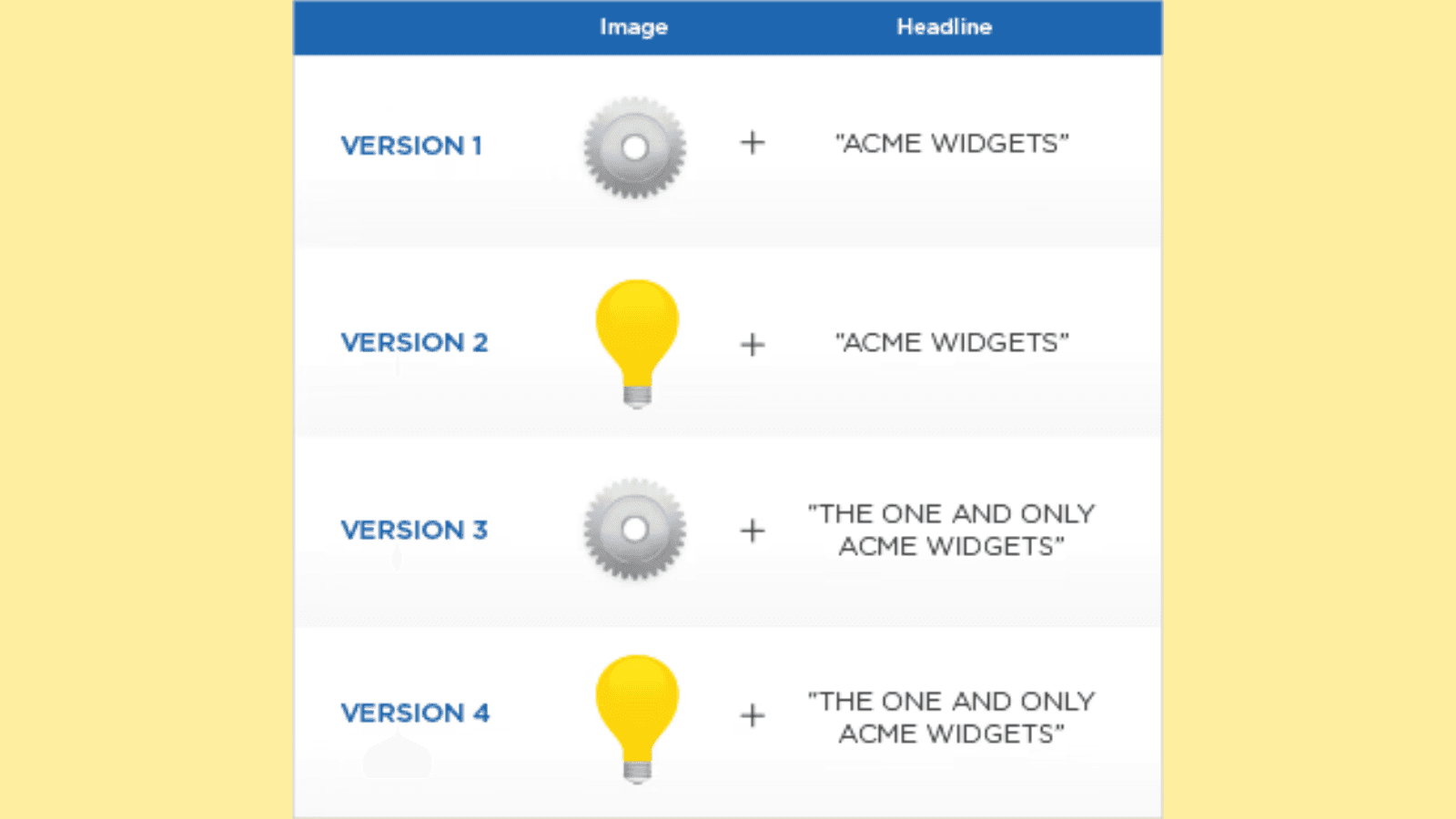
Each combination requires sufficient traffic to produce statistically valid results. This high traffic requirement makes A/B and multivariate testing better suited for different use cases, with MVT being ideal for large-scale websites or campaigns. By addressing these requirements, MVT ensures accurate and reliable data to inform complex optimization strategies.
5. Test Duration
The duration required to run AB testing and multivariate testing is also another key difference.
A/B testing generally requires a shorter duration because it involves fewer variants and a simpler analysis process.
For example, testing two versions of a headline or button can yield results quickly, especially if the website receives a steady flow of traffic. The straightforward nature of AB testing makes it suitable for marketers who need quick insights to inform decisions.
Besides, with fewer combinations to test, achieving statistical significance is faster, reducing the overall time required for experimentation and allowing teams to implement improvements promptly.
On the other hand, multi-variable testing typically takes longer due to the need to evaluate multiple combinations of variables. Each variation, such as different headlines, images, and call-to-action buttons, must receive enough traffic to ensure reliable data.
For instance, multivariate testing is used for complex scenarios, like redesigning a web page layout, where multiple changes interact.
The extended test duration ensures comprehensive insights but requires patience and a significant audience size, making MVT more suitable for high-traffic websites and long-term optimization efforts.
6. Insights
Another multivariate testing vs AB testing difference is the insights derived from the tests.
A/B testing provides broad insights into which version of a single element performs better. For instance, an AB test of a call-to-action button might reveal that one color drives more clicks than another.
For instance, Performable conducted a simple A/B test by changing the color of their CTA button from green to red.
The results? The CTA button with red color increased conversion by 21%.
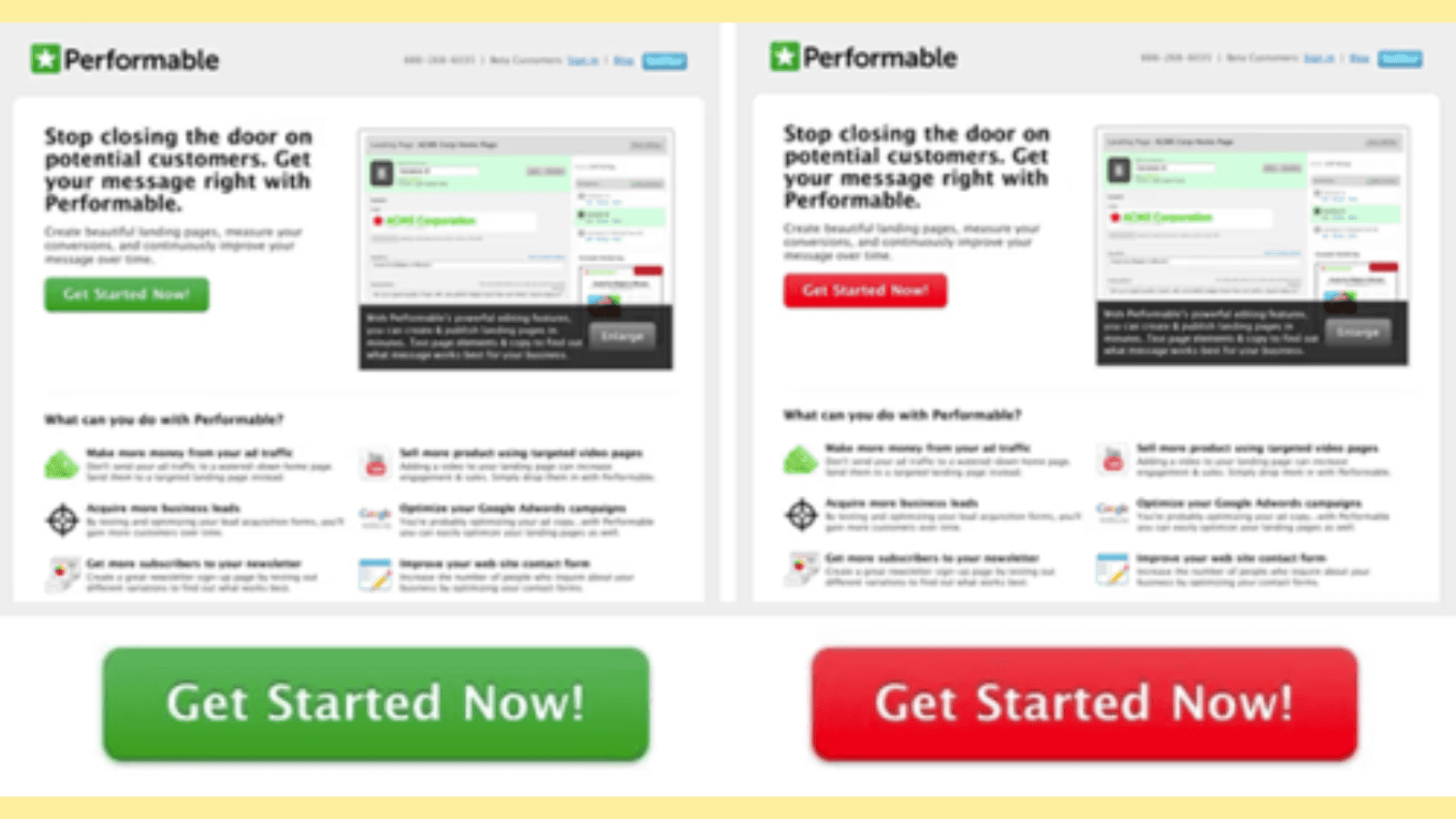
While useful for identifying clear winners, A/B testing does not analyze how different elements interact. This makes it ideal for targeted improvements, such as tweaking one aspect of a page to enhance conversions. A/B and multivariate testing both generate valuable insights, but A/B testing focuses on overall effectiveness rather than granular details.
Multivariant testing offers deeper, more detailed insights by evaluating how individual elements contribute to performance and how they interact with each other. For example, a multivariate testing project might show how a specific headline combined with a particular image impacts conversions.
Marketers can use this granular data to understand which combinations of elements work best, making multi-variable testing a powerful tool for comprehensive optimization. Overall, while more complex, MV testing uncovers insights that go beyond surface-level changes, helping refine every aspect of a webpage for maximum effectiveness.
7. Use Cases
Lastly, AB and multivariate testing differ in their use cases.
A/B testing is ideal for evaluating a single change, such as a new call-to-action button, headline, or image. For example, AB testing multiple variants of a banner design can quickly reveal which option resonates better with users.
This simplicity makes it perfect for campaigns with one clear focus, such as improving click-through rates or driving more downloads. A/B and multivariate testing have distinct strengths, but A/B testing excels in situations where marketers seek fast, actionable insights for specific elements.
Multivariate testing, on the other hand, is used for optimizing more complex scenarios, such as testing a web page's overall layout or multiple variables simultaneously.
For instance, a multi-variable testing experiment might assess how different headlines, images, and buttons interact to impact user engagement.
This makes MVT particularly effective for redesigns or strategic projects where understanding the combined effect of various elements is crucial.
AB Testing Process Vs Multivariate Testing Process
When it comes to optimizing websites, marketing campaigns, or product features, testing plays a crucial role. While A/B testing and multivariate testing share similarities, they are distinct in how they approach and solve problems. Here is a breakdown of the A/B testing process versus the multivariate testing process.
1. Defining Objectives
The first difference between the AB and multivariable testing process is defining objectives.
As usual, the first step in any testing process is to define the objective of the test.
In A/B testing, the goal is usually very specific—improving a single aspect of a webpage or an app.
You’re A/B testing goals could include:
Increasing click-through rates (CTR)
Improving conversions or user engagement
Reducing bounce rates.
For example, if you’re testing two versions of a landing page, your objective might be to see which version leads to more sign-ups.
In multivariate testing, the objectives can be more complex because multiple elements of a webpage or an app are tested simultaneously. The goal is to understand how different combinations of elements (such as headlines, images, and buttons) work together to impact the overall user experience or performance metrics.
Multivariate testing is used to identify the best combination of variables on a single page or within a single campaign.
For instance, your goal could be to increase sign-ups, but this time you want to test different combinations of headlines, images, and CTA buttons to see which combination of variables helps you achieve that goal.
2. Selecting Variables
The AB and multivariant testing process also differs in the way variables are selected.
A/B testing focuses on one primary variable—such as the headline, the button color, or CTA. You compare two versions (variable A: the control/original) and (variable B: the variation), to understand which one performs better in terms of the defined objective.
For example, if you want to test whether changing the headline on your landing page increases sign-ups. Your variable is the headline text.
In multivariate testing, the process involves selecting multiple variables to test at the same time. These variables can include different combinations of images, headlines, call-to-action buttons, colors, and layout changes.
For example, a multivariate test may explore how various combinations of three different headlines and four button colors affect conversion rates.
3. Creating Variants
Creating variants in A/B testing is different from multivariate testing as well.
In A/B testing, you create two variants: the control (A) and the variation (B). The variant (B) includes one specific change to the element you're testing, while variant A remains unchanged. The key is that only one element is altered to see its impact clearly. The process is straightforward, making it easier to analyze and draw conclusions.
For example, if you want to increase your signups your variants could be:
Version A (Original): "Join Today for Free"
Version B (Variant): "Sign Up Now for Exclusive Access"
On the other hand, the multivariate testing variants creation process involves creating multiple variants, as it tests multiple combinations of different elements. Each combination is treated as a separate variant.
For example, if you’re testing two headlines and three button colors, the multivariate test would generate six different variants, each with a unique combination of those elements. These variants are then tested simultaneously to determine the best-performing combination.
Your variants could be like this:
Variant 1: A1 + B1 + C1
Variant 2: A1 + B1 + C2
Variant 3: A1 + B2 + C1
Variant 4: A1 + B2 + C2
Variant 5: A2 + B1 + C1
Variant 6: A2 + B1 + C2
Variant 7: A2 + B2 + C1
Variant 8: A2 + B2 + C2
4. Splitting Traffic
Splitting traffic is also different in multivariate testing vs A/B testing.
Once you have created the variants, the next step is to split the traffic. In A/B testing, you divide the traffic evenly between the control (A) and the variation (B).
For example, if you have 1,000 visitors, 500 visitors will see version A, and 500 will see version B. This ensures that both variants are tested under similar conditions, allowing you to compare their performance accurately.
In multivariate testing, you split traffic across all the different variants, which can be more complex depending on how many variables and combinations are being tested. If you’re testing three variables with two options each, your traffic might be split into six groups, with each group seeing a different combination of those variables.
The larger the number of variables and combinations, the more traffic you need to ensure each variant receives enough data to be statistically significant.
5. Running the Test
When it comes to running the test, things get interesting in multivariate vs AB testing.
Running the A/B test is relatively simple. After splitting the traffic, you run the test for a predetermined period or until you collect sufficient data to make a decision. It’s essential to run the test for a sufficient duration to account for variations in user behavior, such as weekday vs. weekend traffic. Typically, an A/B test can run for around 1-2 weeks, depending on your traffic volume.
Multivariate testing is more complex to manage. Because you are testing multiple combinations of variables, the test needs to run for a longer period and with more traffic to gather statistically significant results for all the combinations.
The process of analyzing multivariate tests also requires more advanced tools and techniques to assess how different elements and their combinations impact the test's outcomes.
Here, you need to allow the test to run for a few weeks, ensuring that each combination gets enough traffic to ensure statistical significance.
6. Analyzing Results
Once you have collected sufficient data in your test, analyzing the results of an A/B test is straightforward. Since there are only two variants, you simply compare the performance metrics of each. You’ll look at conversion rates, click-through rates, or other relevant metrics to see which version performed better.
For example, if Version B (the new headline) has a higher conversion rate than Version A (the original), then it’s clear that the new headline worked better.
Results analysis of multivariate tests is more complicated. You’ll need to evaluate the performance of each variable in isolation, as well as how the different variables interact with each other.
You may require advanced statistical techniques, such as regression analysis or factorial design, to understand how combinations of variables impact the overall outcome. Multivariate testing allows you to see not just which element works best, but how multiple elements work together to affect the result.
7. Decision Making
Now into the decision-making process of multivariate vs A/B testing.
The decision-making process in A/B testing is simpler: based on the results, you can choose the variant that performed better and implement it. If version B led to more sign-ups or conversions, you can confidently adopt it as your new version.
In multivariate testing, decision-making can be more complex. You may need to analyze various combinations to identify which one has the most significant impact. The decision often involves picking the combination that maximizes your objective (e.g., conversion rate) while considering how each variable works together.
When & How To Conduct A/B Testing
While it is recommended to conduct A/B tests regularly, there are scenarios where it is most suitable to do it.
Below are the instances when conducting A/B testing is necessary and how to do it in each scenario:
1. When Optimizing Website or App Elements
When you want to improve specific elements on your website or app—such as buttons, headlines, forms, or images—A/B testing helps you understand which variations resonate best with your visitors. Optimizing individual components can have a huge impact on the user experience and conversion rates.
In this case, you might want to improve conversion rates, click-through rates(CTR), or engagement metrics.
Here is how to conduct A/B tests to optimize your website or app elements:
Step 1: Identify the element to test: Choose one element to optimize at a time. For example, you might want to test the color of a CTA button, the wording of a headline, or the layout of a form.
Step 2: Create two variations: Create two versions of your web page. Version A (the original) and Version B (the variation with a small change). Example: Version A might have a blue button with the text “Sign Up Now,” while Version B has a green button with the text “Join Today.”
Step 3: Split the traffic: Direct 50% of your website visitors to Version A and the other 50% to Version B. Ensure the traffic is split randomly and evenly.
Step 4: Monitor the performance: Track the conversion rates or user interactions for both versions. In this case, you might be measuring how many people click the CTA button.
Step 5: Analyze the results: After enough data is collected, compare the performance of both versions. The version with the higher conversion rate or more engagement is the winner.
2. When Launching New Features or Designs
When you introduce new features or redesign elements on your website, it's essential to test how these changes affect user behavior. A/B testing allows you to see if your new design or feature is better than the old one or if further adjustments are necessary.
For instance, you might want to compare the old vs. new homepage design.
Follow these simple steps to AB test your new features or designs:
Step 1: Define the change: Whether you’re testing a new feature, design, or layout, identify exactly what’s changing. For example, you might want to test a new homepage design or a new checkout process.
Step 2: Create variations: Build two versions of your page—Version A (the old design or feature) and Version B (the new design or feature).
Step 3: Set up split testing: Direct visitors to one of the two versions, making sure each version is shown to an equal portion of your audience.
Step 4: Track key metrics: Measure the relevant metrics such as user engagement, bounce rates, and conversion rates. For example, if you are testing a new checkout process, track how many visitors complete the checkout.
Step 5: Analyze and decide: Lastly, evaluate the results of your test. If Version B (the new design or feature) performs better, consider implementing it permanently. If not, refine the design or feature and test again.
3. When Improving Marketing Campaigns
The other scenario to conduct A/B tests is when improving marketing campaigns.
A/B testing is crucial when you’re running marketing campaigns, whether you’re using emails, landing pages, or ads. Testing different versions of your campaigns helps ensure you're reaching your audience with the most effective message.
For example, you might want to compare two ad headlines to see which generates higher clicks to improve your campaigns.
Here is how to run the test:
Step 1: Set clear campaign goals: Understand what you're trying to achieve with your marketing campaign—such as higher open rates, more clicks, or better conversions.
Step 2: Create two campaign variants: For email campaigns, this might mean testing different subject lines, content, or CTAs. For landing pages or ads, you might test images, headlines, or offers.
Step 3: Split the audience: For emails, send one version to half of your list and the other version to the other half. For web pages or ads, split traffic equally between the two variations.
Step 4: Measure the results: Track the specific metrics you set out to improve. For email campaigns, you may track open rates or click-through rates. For landing pages, track conversion rates.
Step 5: Optimize based on results: Once you have enough data, choose the version that outperforms the other and roll it out to the rest of your audience.
4. When Enhancing User Experience (UX)
Statistics are as clear as day:
According to PriceWaterhouseCoopers (PWC), 32% of customers would leave a brand they loved after just one bad experience.
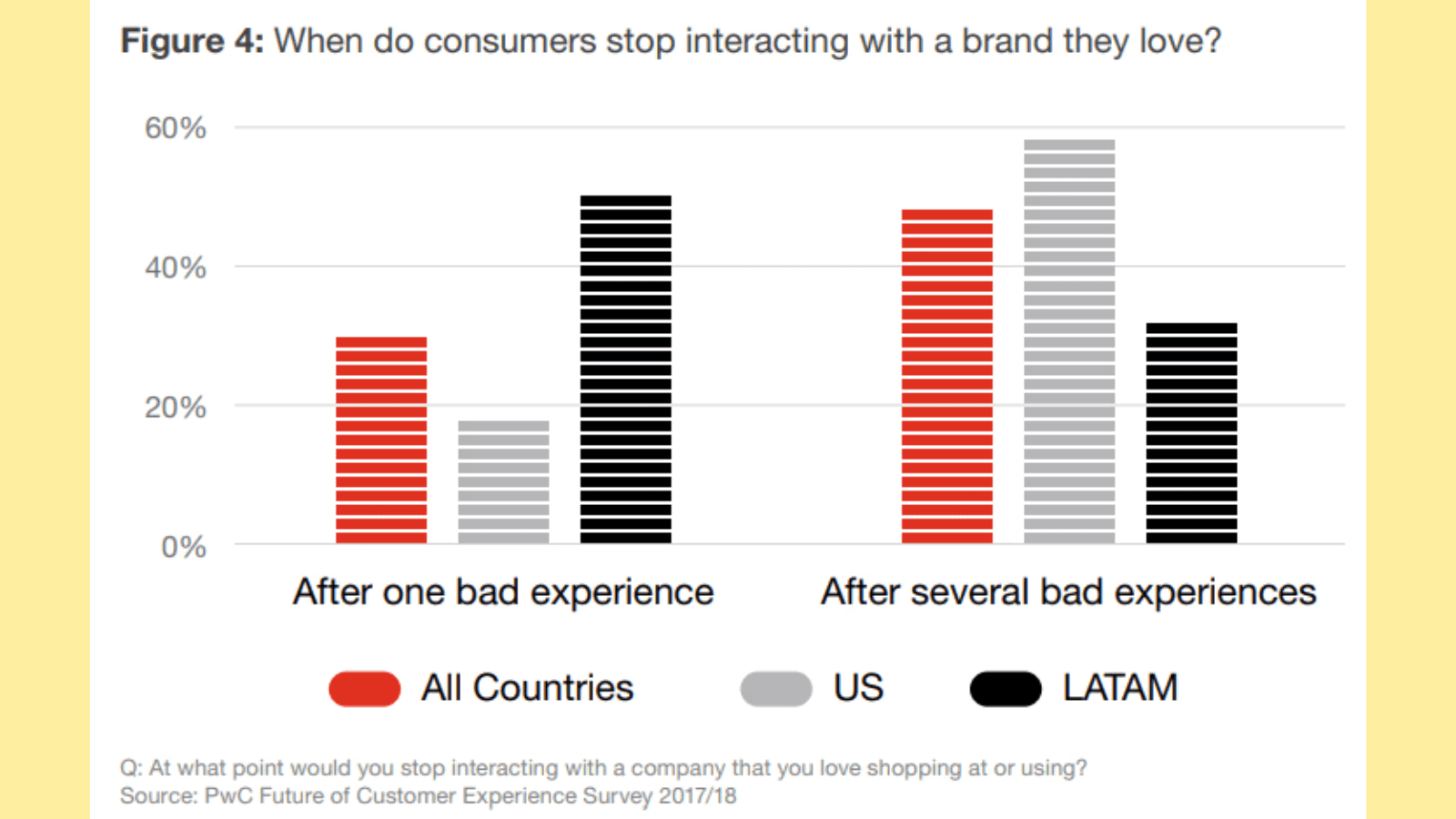
88% of online customers say they wouldn’t return to a website after having a bad user experience.
8 in 10 customers are willing to pay more for better customer experience.
What do all these stats mean for marketers?
Customers want nothing but a good experience when interacting with your digital assets. Hence, you must improve the overall user experience on your website or app at all times.
A/B testing is an excellent way to make data-driven decisions for this. It allows you to test things like page load times, navigation flow, content placement, and more to enhance UX and usability.
Here is how to run A/B tests to improve user experience:
Step 1: Identify UX areas to improve: Focus on areas of your website that affect the user experience. For example, you could test the placement of your navigation menu or the speed at which your page loads.
Step 2: Create variations: Build Version A (the original UX) and Version B (the variation with your improvement). For instance, you could try testing a faster page load time by optimizing images or scripts.
Step 3: Split traffic: Send half of your visitors to Version A and the other half to Version B. Ensure you're not overwhelming your audience with too many variations.
Step 4: Collect user data: Look at user behavior metrics like session duration, bounce rate, or how easily users navigate through your site.
Step 5: Make improvements: If Version B (the improved user experience) leads to lower bounce rates and more engaged visitors, consider making the change permanent.
5. During Data-Driven Decision Making
Lastly, A/B testing is essential for making data-driven decisions.
As Dan Zarrella from HubSpot famously said, “Marketing without data is like driving with your eyes closed.”
But how do you get this marketing data?
A/B testing can be a great tool for this.
Hence it should be an integral part of any data-driven decision-making process. When making important decisions about your website or app, use A/B testing to validate assumptions and ensure that any changes you make will lead to positive results.
Here is how to run A/B tests to make data-driven decisions:
Step 1: Identify your hypothesis: Based on data, you may hypothesize that a change will improve performance. For example, you might believe that changing the color of your CTA button will increase conversions.
Step 2: Design the experiment: Create two versions—Version A (the current design) and Version B (the proposed change). Ensure the change is measurable.
Step 3: Split testing: Randomly direct half of your traffic to Version A and the other half to Version B. This eliminates bias and ensures accurate results.
Step 4: Track and collect data: Use tools like Fibr AI, Google Analytics, Optimizely, or VWO to track the performance of both versions and gather insights.
Step 5: Analyze and take action: After gathering sufficient data, analyze the results to see if the change leads to an improvement. If it does, you can confidently roll out the change. If not, revise and retest.
Remember, A/B testing is not just about testing for the sake of it. It’s about making informed decisions that are based on real data for continuous improvement and better results.
When & How to Conduct Multivariate Testing for Web Pages
There are scenarios when A/B testing can’t help but multivariate testing does.
Here are scenarios when you will need to conduct multivariate testing and how to do it for each scenario:
1. When You Have Multiple Variables to Test
Multivariate testing is ideal when you have more than one variable you want to test simultaneously. For example, if you are looking to optimize multiple elements on a page, such as headlines, images, and button placements, MVT can help you determine which combination of these elements drives the best performance towards a specific goal, like increasing conversion rates.
Here’s how to conduct multivariate testing with multiple variables:
Step 1: Identify multiple variables: Start by selecting the elements you want to test. These could include a headline, a call-to-action (CTA) button, an image, or even the page layout.
Step 2: Create variants for each Element: Unlike A/B testing, where you create only two versions, multivariate testing requires you to create different combinations of changes for each variable. For example, if you test two headlines and two images, you'll have four possible combinations to test.
Step 3: Build the test pages: Each combination of the variables will form a unique page variant. For example:
Variant 1: Headline A + Image 1
Variant 2: Headline A + Image 2
Variant 3: Headline B + Image 1
Variant 4: Headline B + Image 2
Step 4: Split the traffic: Divide the incoming traffic equally among the different variants. For example, 25% of visitors see Variant 1, 25% see Variant 2, and so on.
Step 5: Analyze the results: Once the test has run long enough, analyze the performance of each combination based on your conversion goals (e.g., lead generation, click-through rates, purchases). The combination that performs best is your winner.
2. When You Want to Optimize a Specific Conversion Goal
The other scenario when multivariate testing is necessary is when optimizing a specific conversion goal:
If you're aiming to improve specific conversion goals on your web page, such as increasing the number of sign-ups, purchases, or clicks, multivariate testing is highly beneficial. It allows you to optimize several factors at once and measure how they contribute to your conversion goal.
Here are simple steps to optimize for a specific conversion goal with multivariate testing:
Step 1: Define your goal: Begin by clearly defining your conversion goal. This could be anything from increasing email sign-ups to improving the checkout completion rate.
Step 2: Choose the variables that influence the goal: Once you've defined your goal, identify the elements on your page that you believe affect the outcome. For example, if you want to increase purchases, you might test variations of product images, prices, CTA buttons, and product descriptions.
Step 3: Set up the variants: Create different versions of each element. With more variables, you'll need to create more combinations. For example, two buttons, two images, and two headlines could lead to 8 unique variants (2 x 2 x 2).
Step 4: Run the test and measure the impact: Track which combination of elements leads to the highest conversion rate for your goal. If your goal is to increase purchases, you might track how many visitors complete the purchase after interacting with each variant.
Step 5: Implement the best combination: After analyzing the results, use the combination that performed best for your conversion goal.
3. When you have enough traffic
As mentioned above, multivariate testing is suitable for high-traffic websites.
The test requires a significant amount of traffic to produce statistically valid results. If your website or campaign has low traffic, the results from an MVT may not be reliable due to a lack of data.
You should only consider running multivariate experiments when your site has enough visitors to ensure that each variation gets a sufficient number of views for accurate conclusions.
Follow these simple steps to run multi-variable testing on high traffic website:
Step 1: Check your traffic volume: Use analytics tools (like Google Analytics) to evaluate the amount of traffic your website receives. Multivariate testing works best with a steady stream of traffic, ideally thousands of visitors per month.
Step 2: Set up your experiment: Similar to the earlier steps, define your variables, create variants, and split your traffic evenly among them. Ensure that you have enough visitors in each group for the results to be statistically significant.
Step 3: Monitor the test duration: Keep the test running for an appropriate amount of time to gather enough data. The test should run long enough to ensure that your results are not skewed by daily fluctuations in traffic.
4. When You’ve Already Run A/B Tests
If you've already conducted A/B tests on individual elements (like testing different headlines, CTAs, or images) and you want to test combinations of those elements, multivariate testing takes it a step further.
After identifying which individual elements perform best, you can test various combinations to see how they work together in driving conversions.
Here is how to do multivariable testing:
Step 1: Analyze previous A/B test results: Look at the results from your A/B tests and identify which individual elements (e.g., headlines, images, buttons) performed the best.
Step 2: Combine the top elements: Now that you know which individual elements are effective, combine them to create different variations. For example, if Headline A performed best in A/B tests, and Image 2 performed best, combine them to form a variant.
Step 3: Set up multivariate testing: Create different combinations of these elements (headlines, images, and buttons) and split the traffic between them. Ensure you're testing multiple combinations at once.
Step 4: Measure the impact on conversions: Track how the different combinations of variables affect your conversion goal. This could be sales, clicks, form submissions, etc.
Pros & Cons Of AB Testing vs Pros & Cons Of Multivariate Testing
Both A/B testing and multivariate testing are essential tools for optimizing web pages, but they each have their own strengths and weaknesses. Understanding the pros and cons of A/B testing vs multivariate testing can help you decide which method to use depending on your specific goals.
Pros & Cons of A/B Testing
Pros of A/B testing:
Simplicity: A/B testing is straightforward to implement. It compares two variants of a page or element (such as a headline or CTA button) to determine which one performs better. This simplicity makes it ideal for beginners.
Clear results: Since only one variable is changed at a time, A/B testing provides clear, focused results, allowing you to directly measure the impact of specific changes on conversion rates.
Quick to set up: A/B tests can be set up quickly, especially when compared to multivariate experiments. You can start testing with minimal changes, which makes it easy to iterate rapidly on your webpage.
Statistical significance: With fewer variants, A/B testing requires less traffic to reach statistically valid results. If your site has moderate traffic, you can get reliable results with fewer visitors.
Cons of A/B testing:
Limited scope: A/B testing only allows you to test one variable at a time. If you want to test the combined effect of multiple variables (like headlines, images, and buttons), it can be inefficient.
Longer test duration: Because you're only testing one variable, it can take longer to reach conclusive results, especially if your site doesn't have high traffic.
Cannot Test Combinations: A/B testing doesn't allow you to assess how different combinations of elements work together, which can be a limitation when trying to understand the broader impact of changes.
May require multiple tests: To fully optimize a page, you might need to run several A/B tests for each element, which can become time-consuming and fragmented.
Pros & Cons of Multivariate Testing
Pros of multivariate testing:
Comprehensive testing: Multivariate testing allows you to test multiple variables at once, providing insights into how different combinations of elements affect your webpage's performance. This is ideal when you want to optimize multiple aspects of a page (e.g., headline, image, and CTA button) simultaneously.
Optimized conversion rates: By testing multiple combinations, multivariate testing can help identify the best combination of elements, which can lead to higher conversion rates and more effective optimization.
Efficient for large sites: For websites with high traffic, multivariate testing can quickly provide detailed insights. Testing multiple variations in one experiment can save time and effort compared to running several A/B tests.
Detailed data insights: Multivariate testing provides more granular insights, helping you understand how each element's combination influences user behavior. This is useful when aiming for highly specific optimization goals.
Cons of multivariate testing:
Requires high traffic: Multivariate testing needs substantial traffic to generate statistically valid results. Sites with low traffic may not see reliable outcomes due to insufficient data.
Complex setup: Multivariate tests are more complicated to set up than A/B tests. You'll need to create multiple variations for each variable, which can be resource-intensive and require careful planning.
Longer test duration: Since you're testing several combinations at once, it may take longer to gather enough data to determine a winner, especially if your website doesn't have high traffic.
AB Testing or Multivariate Testing: Which Is Better?
When comparing A/B testing vs multivariate testing, the decision largely depends on the complexity of the changes you wish to test and the amount of traffic your site receives.
A/B testing is simpler and works well when you want to test a single element, whereas multivariate testing is more appropriate when you want to test multiple variables simultaneously and understand their interactions.
In summary:
A/B Testing is best for testing individual elements like headlines, CTAs, or images.
Multivariate Testing is more suited for testing several elements at once, like different combinations of headlines, images, and CTAs.
Want to optimize your website efficiently to improve conversions with A/B testing?
Use Fibr AI.
The free A/B testing tool offers powerful features tailored for enhancing conversion rates and personalizing user experiences such as:
Advanced testing capabilities: Perform comprehensive tests with various configurations to optimize landing page performance and ad effectiveness.
AI-powered conversion optimization: Automatically analyze test results and provide actionable insights to improve conversion rates dynamically.
Bulk personalization: Customize up to 10 landing pages simultaneously in the basic setup or unlimited pages with advanced plans.
Integrated analytics: Combine Google Analytics, Google Tag Manager, and Fibr’s advanced insights for a thorough understanding of user behavior.
Book a personalized demo here or get started for free to see how Fibr AI can help you with your A/B testing project!