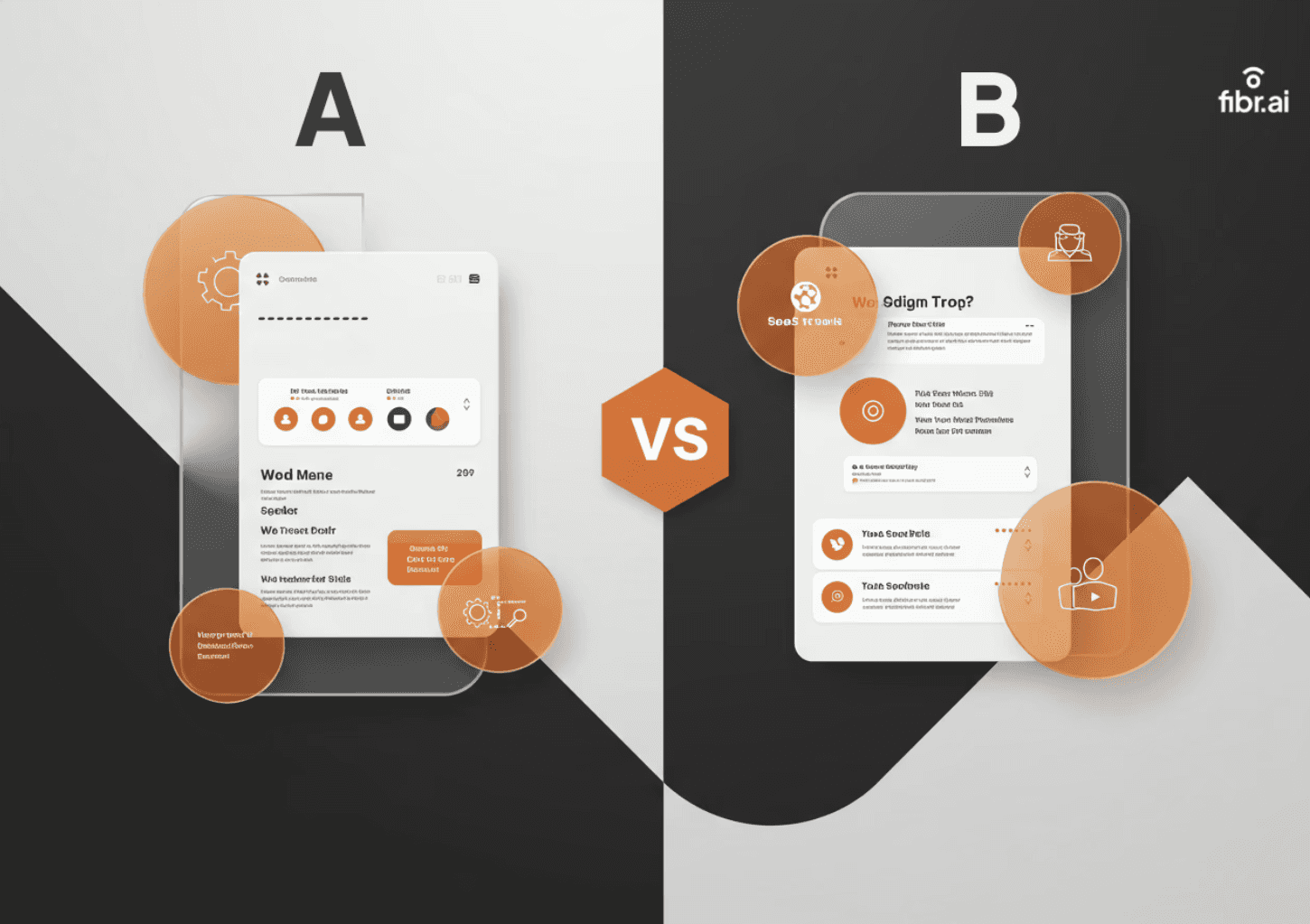

Ankur Goyal
TLDR:
In SaaS, one faulty UI change or pricing redo is enough to tank conversions and retention.
A/B testing fixes that. It helps you experiment with real user data so that you can make meaningful changes to your product.
Define clear goals, pick the right metrics, and make sure you have enough traffic for reliable results.
Bad tests equals bad decisions. Avoid layman mistakes like stopping tests too early, testing too many changes at once, and ignoring retention impact.
Track the right numbers. Focus on trial-to-paid conversion, churn, LTV, and feature adoption,not just clicks.
Platforms like Fibr automate testing, generate high-converting variations, and analyze results so you don’t have to.
SaaS growth is a walk on nails—unless you’re A/B testing.
For SaaS companies, every click, signup, and retention metric matters. The smallest changes have huge consequences. A confusing onboarding flow? Users churn before they even see the product’s value. A new pricing model? It might attract bargain hunters but drive away high-value customers.
A/B testing is the answer to all of that.
In this guide, we’ll break down everything you need to know about SaaS A/B testing. Let’s begin with the basics…
A/B testing (aka split testing) is the practice of comparing two versions of a product feature, UI element, email, or pricing page to see which one performs better.
You randomly divide your users into two groups, expose them to different versions, and let data decide the winner.
Let’s say you run a SaaS tool for project management. Your sign-up rate is… just bad. You suspect that your Start your free trial button isn’t exactly the ‘Click me!’ type.
So, you A/B test two versions
Version A (control): The existing dull button.
Version B (variant): A bold, orange button with the text “Take control of your projects now!”
You roll out the test to 50,000 visitors and track the conversion rate. A week later, the results are in: Version B boosted sign-ups by 27%. So, you roll out version B across your site.
That’s how A/B tests work.
SaaS giants like Dropbox and Google run hundreds of tests yearly. They are constantly changing up onboarding flows, email subject lines and even pricing models.
Interestingly, 70% of A/B tests fail. This means that the new version isn’t always better. But when it does, the payoff can be massive.
Why is A/B Testing Important for SaaS Companies?
A/B testing is a fundamental pillar of growth in SaaS. The reason? SaaS businesses operate in an environment where user behavior, engagement, and retention directly impact revenue.
Unlike traditional businesses, where a sale is a one-time event, SaaS companies rely on ongoing customer interactions. Even tiny optimizations in onboarding, pricing, or even a CTA button have a big influence on conversion rates, churn, and lifetime value (LTV).
Here’s why A/B testing is non-negotiable for SaaS companies:
1.A/B testing gets rid of assumptions and bias
Product teams, marketers, and executives all have opinions about what works best. But opinions don’t pay the bills—data does. A/B testing gives you definitive answers by replacing subjective decision-making with quantifiable evidence.
A pricing page redesign might “feel” more intuitive to your team, but will it actually drive more upgrades? Without testing, you’re making a change based on intuition, not data.
2.It increases conversion rates at every stage
From free trials to paid upgrades, conversion rates define SaaS success.
If 1,000 visitors land on your pricing page and only 20 converts, that’s a 2% conversion rate. But what if a simple headline tweak could raise that to 3%? That’s a 50% increase in sign-ups, without spending a cent on extra traffic.
A/B testing helps optimize every step of the funnel:
Sign-up forms (shorter forms vs. longer forms)
Onboarding sequences (interactive tutorials vs. video walkthroughs)
Feature adoption strategies (pop-ups vs. email nudges)
Pricing models (monthly vs. annual emphasis)
Even a small lift in conversion rates compounds over time and leads to higher revenue and lower acquisition costs.
3.It reduces churn and improves retention
Getting users to sign up is just step one. Keeping them engaged is where the real challenge lies. A/B testing identifies friction points that cause users to drop off.
For example, if your data shows that 40% of trial users never return after day one, that’s a red flag. You could A/B test different onboarding flows:
Version A: A product tour with an interactive checklist.
Version B: A more personalized approach with in-app prompts and email follow-ups.
By tracking retention metrics, you can then determine which experience keeps users engaged longer. And in SaaS, higher retention equals higher LTV; meaning fewer resources wasted on constantly replacing lost users.
4.It optimizes pricing and monetization
Pricing is one of the most critical (and difficult) aspects of SaaS growth. Charge too little, and you leave money on the table. Charge too much, and you scare away potential customers.
A/B testing lets you experiment with different pricing models, feature bundles, and discount strategies. It also validates whether users are willing to pay more for premium features or whether a freemium-to-paid conversion strategy is more effective.
5.It maximizes marketing ROI
Marketers in SaaS often work with limited budgets. Instead of pouring money into more traffic, A/B testing maximizes existing traffic.
Testing variations of landing pages, email subject lines and ad creatives ensures that every marketing dollar is spent efficiently.
How to Set Up an A/B Test for SaaS
A/B testing sounds simple: show two versions, see which one wins, and profit.
But IRL, a poorly executed test means misleading results, wasted time, and bad decisions.
To get it right, you need a structured approach. Here’s how to set up an A/B test for your SaaS product.
1. Define your goal (What are you testing?)
Every A/B test should start with a clear objective. Are you trying to increase sign-ups, reduce churn, boost feature adoption, or optimize pricing? Without a goal, you won’t know whether your test was a success.
Potentials goals look like
Improving the conversion rate of your free trial sign-up page.
Increasing engagement with a newly launched feature.
Reducing drop-offs in the onboarding flow.
Pro tip: Avoid testing multiple things at once. If you change both the CTA and the headline, you won’t know which one actually caused the impact.
2.Pick a single variable to test
An A/B test compares two versions of a single element, so choose wisely. Some high-impact variables in SaaS include:
CTA buttons
Headlines and messaging
Pricing page layout
Onboarding flow
Email subject lines
Let’s say your trial-to-paid conversion rate is low. Instead of redesigning the entire onboarding process, you could A/B test a welcome email variation: one with a video tutorial and one without, to see if it improves engagement.
3.Split your audience randomly
Once you’ve chosen what to test, you need to split your users into two groups:
Group A (control): Sees the original version.
Group B (variant): Sees the modified version.
For accurate results, these groups must be randomly assigned and statistically significant ( large enough to detect a meaningful difference). A sample size calculator will tell you how many users you need.
The rule of thumb is that if you’re running a small test with only 500 users, the results may not be reliable. More users equals trustworthy data.
4.Run the test for a meaningful duration
Stopping a test too early (or running it too long) will definitely lead to skewed data. A/B tests should run long enough to capture different user behaviors but not so long that external factors (like seasonality) interfere.
For high-traffic pages, one to two weeks is usually enough. For low-traffic SaaS features, you may need a month or more to get reliable results.
Pro tip: Don’t check results too frequently. Statistical fluctuations sometimes open up premature conclusions.
5.Analyze results and look for statistical significance
Once your test has run its course, analyze the data. Did the variant outperform the control? By how much? Look beyond surface-level metrics. Consider retention impact, engagement depth, and revenue per user.
The conversion rate, bounce rate, and time spent of page are the most important metrics you need to pay attention to.
Use tools like Fibr, Optimizely, or VWO to crunch the numbers. If the improvement is statistically significant (usually 95% confidence or higher), you can confidently roll out the winning version.
6.Iterate and keep testing
A/B testing isn’t a one-time thing. Even if a test fails, you’ve still learned something valuable. The best SaaS companies are always optimizing: testing pricing, onboarding, UX tweaks, and everything in between.
Best Practices for SaaS A/B Testing
Now that you know how its done, follow these best practices to produce accurate, actionable, and scalable SaaS A/B tests.
1.Track downstream metrics, not just surface-level conversions
A/B tests often focus on short-term wins like a higher sign-up rate or a lower bounce rate. But in SaaS, the real impact is often downstream (post-sign-up behavior).
For example, let’s say you test a new onboarding flow that increases trial sign-ups by 15%. Sounds great, right? But what if it also increases churn after 30 days because users weren’t properly educated on the product?
How to do it right
Track metrics beyond the test window (like retention at 14, 30, or 90 days).
Keep an eye on feature adoption instead of just sign-ups. Dd users actually engage with the product after the change?
Use cohort analysis to see how different user groups behave over time.
If you’re only looking at top-of-funnel improvements, you will end up optimizing for vanity metrics rather than long-term growth.
2.Ensure statistical power (don’t call the test too early)
One of the most common SaaS A/B testing mistakes is stopping a test too soon. Just because a variation seems to be “winning” after three days doesn’t mean it actually is.
Why?
Early fluctuations are normal. Your first few hundred users might not represent the broader user base.
You need statistical power (aka a large enough sample size) to make reliable conclusions.
Use a sample size calculator before launching a test. Tools like Evan Miller’s calculator can help. And like we already said, avoid peeking at results too early. Wait until the test reaches at least 95% statistical confidence before making a decision.
If your traffic is too low to reach significance in a reasonable time, you can try running Sequential Testing (which allows for early stopping based on Bayesian analysis).
Half-baked conclusions lead to bad product decisions. Let the data mature.
3.Test against the right user segments
All SaaS users don’t behave the same way. If you test all users at once, you are diluting your insights. Instead, segment your audience based on behavior, plan type, or user intent.
Bad segmentation looks like
Running a test on both free and paid users, even though paid users have completely different motivations.
Testing onboarding changes on returning users instead of new users (skewing the results).
You need to target users who will actually experience the change. If testing a pricing page tweak, only include new visitors in the test, not users who already signed up.
Exclude irrelevant traffic. If your SaaS is global, time zone and cultural factors might skew results (like pricing sensitivity in different regions). Along with that, use behavioral segmentation. Compare results between power users vs. first-time visitors to see how different groups respond.
Without proper segmentation, you might think a test "failed" when it actually worked for a specific, valuable audience segment.
4.Beware of the “novelty effect” in UX changes
Whenever you launch a new design, people are likely to engage with it more simply because it’s new, not because it’s better. This is called the novelty effect, and it misleads A/B test results.
If you redesign your dashboard UI, users might initially click around more. But that doesn’t mean they’re more productive. After the initial excitement wears off, engagement might drop back to previous levels.
To avoid the novelty effect
Run tests for at least 2 to 4 weeks to let the novelty effect wear off.
If possible, run a holdout group: keep a small subset of users on the old version for longer and compare long-term behavior.
Look at deep engagement metrics like time spent per session, repeat usage, and task completion rates.
If a test shows a short-term spike but long-term decay, you’re probably seeing the novelty effect in action.
5.Test at the right stage of the SaaS lifecycle
Not all SaaS companies should blindly test the same things. The type of A/B tests you should run depends on where your SaaS business is in its growth cycle.
If you’re an early-stage SaaS (pre-PMF), focus on high-impact tests like onboarding and pricing. Don’t obsess over micro-optimizations like button color. Bigger strategic decisions matter more.
For growth-stage SaaS, optimize expansion and monetization. Test upsell prompts, feature bundling, and pricing tiers. A/B test referral programs and viral loops to scale user acquisition.
Mature SaaS are free to test retention drivers (like proactive churn prevention, and re-engagement campaigns. These also benefit from experimenting with advanced segmentation, like customizing onboarding flows for enterprise vs. SMB customers.
Running A/B tests on the wrong things at the wrong time leads to wasted effort. Align testing with your SaaS growth stage.
What Not to Do While A/B Testing for SaaS?
We like to think of A/B testing as a game of chess—one wrong move, and all your efforts hit a wall. Most guides will tell you generic mistakes like ‘not testing long enough’, or ‘not defining goals’, but let’s go beyond that.
Here’s what not to do
Do not mix new and returning users in onboarding tests
Most onboarding A/B tests focus on improving trial conversions, but the problem is that new users and returning users behave very differently. Returning users already have some product familiarity, while new users are seeing it for the first time.
If you run an onboarding experiment and include returning users who have already seen parts of your product, your data will be completely skewed. A new onboarding flow might “fail” simply because returning users find it redundant—when it might work great for brand-new users.
To avoid this trap, segment new users separately and run the test only on them. Use a unique experiment identifier in your database to prevent old users from being bucketed into the test.
If you mix user cohorts, you’ll end up killing experiments that could have improved first-time user activation.
Do not ignore user intent on pricing page tests
When testing pricing page changes, many SaaS teams just track clicks on the ‘Start trialt or ‘Request demo’ button. Big mistake. The real question isn’t whether a user clicks, it’s whether they convert into a paying customer later.
Some users just browse pricing pages to compare options. If your test only tracks button clicks, you might celebrate a win that doesn’t translate into real revenue.
Instead of just measuring clicks, track downstream metrics like trial-to-paid conversion rate, the average revenue per user (ARPU), and churn rate after 30-90 days. Run a cohort analysis too, to see if the users who clicked converted at a higher rate.
Do not overlap experiments that pollute each other
Many SaaS teams run multiple A/B tests at once: onboarding, pricing, email sequences, in-app nudges. But if these tests overlap with the same users, your results become a mess.
How? Because if a user is exposed to two or more experiments at the same time, you won’t know which one influenced their behavior.
Let’s say you test a new signup flow while also testing a homepage redesign. If sign-ups increase, was it the homepage, or was it the onboarding change?
That’s why you need to tag test participants in your database and ensure they’re not bucketed into multiple overlapping tests. To help with that, use mutually exclusive experiment groups (if someone is in Test A, they can’t be in Test B).
If your A/B tests are stepping on each other’s toes, you’ll never get clean data.
Do not test too many variations at once (without enough traffic)
Running an A/B/C/D test (four variations plus a control) might sound like a great way to speed up optimization. But unless you have high traffic volumes, you’re setting yourself up for useless noise, instead of anything meaningful.
Each additional variation means your traffic is split even further. If you don’t have tens of thousands of users, each group will be too small to draw reliable conclusions. The more variations you add, the longer the test needs to run to reach statistical significance.
If you have low traffic, stick to simple A/B tests (Control vs. Variation A). Use Bayesian statistics if you absolutely need to run multivariate tests with low traffic.
And if you must test multiple ideas, use sequential testing: run one test, get results, and then test the next idea separately.
Do not ignore experiment fatigue
SaaS power users, especially in B2B, interact with your product daily. If you’re always changing things with back-to-back A/B tests, these users notice the changes and will behave differently just because they’re aware they’re being tested.
Frequent UI changes is bound to frustrate users and lead to lower engagement; not because the variation is bad, but because users are tired of constant changes.
Limit major UI/UX tests to once per quarter for frequent users. It's a good idea to use incremental rollouts instead of abrupt A/B tests for major feature changes. Moreover, rotate test participants so that the same users aren’t in experiments all the time.
The Metrics You Need to Track for SaaS A/B Testing
In SaaS A/B testing, you can’t just rely on stuff like CTRs and sign-ups. You need quantifiable, statistically sound data to make decisions that impact growth, retention, and revenue. And that means some daunting-looking formulas. Here we go
The core business metrics (Revenue impact and long-term growth)
These are the metrics that decide if you’re heading towards real business value, or just wasting time.
Customer Lifetime Value (CLTV or LTV)
CLTV estimates the total revenue a customer generates during their time with your product. If an A/B test increases sign-ups but lowers CLTV, its a sign that you’re acquiring low-quality users who churn quickly.
Formula:
CLTV = ARPU ÷ Churn rate
Where
ARPU = Average revenue per user
Churn Rate = Percentage of customers lost per period
If CLTV drops in the variation group, your test might be attracting the wrong audience (like discount hunters who never convert to high-value customers).
Trial-to-paid conversion rate (TTPCR)
This is a critical SaaS metric, especially for freemium and trial-based models.
Formula:
TTPCR = (Number of paid conversions ÷ Number of trial signups) x 100
A low TTPCR signals that you might be attracting unqualified users who never intended to buy.
Monthly recurring revenue (MRR) and Average revenue per user (ARPU)
MRR represents your total predictable revenue per month.
Formula for MRR:

ARPU tells you how much revenue each user generates, on average.
Formula for ARPU:
ARPU = MRR ÷ Total active users
If a pricing test shifts more users to lower-priced plans, your sign-ups might increase, but MRR and ARPU could drop; a potential failure.
Retention and churn metrics
Customer retention rate (CRR)
A high sign-up rate means nothing if users churn. CRR tells you how many customers stick around over time.
Formula:
If, for instance, an onboarding flow improves trial-to-paid conversion but drops CRR, you might be onboarding people who don’t need the product.
Churn rate
This measures how quickly customers leave.
Formula:
Churn rate = (Customers lost in period ÷ Customers at the start of the period) x 100
Time-to-value (TTV)
TTV measures how quickly a new user experiences your product’s core benefit.
Formula:
TTV = Median time taken for users to reach activation event
A successful test should reduce TTV, so that users realize value faster.
User behavior and engagement metrics (To Decode Why Users Convert or Churn)
Feature adoption rate
Measures how many users engage with a new feature.
Formula:
Feature adoption rate = (Users who used feature ÷ Total users exposed to feature) x 100
Click-through rate (CTR) and Click-to-conversion rate (CTCR)
CTR alone doesn’t tell much; you need to track if clicks lead to meaningful actions.
Formula for CTR:
CTR = (Clicks ÷ Impressions) x100
Formula for CTCR (More Important!):
CTCR = (Conversions ÷ Clicks) x 100
If CTR goes up but CTCR drops, you’ve likely created misleading UI changes that encourage accidental clicks.
Statistical confidence (to avoid false positives)
Sample size calculation
Before running an A/B test, estimate the minimum sample size required for reliable results.
The approximate formula goes like this
Statistical significance (p-value and confidence level)
Statistical significance tells you whether the observed differences are real or due to chance.
p-value < 0.05 = There’s less than a 5% probability that the results occurred by random chance.
Confidence Interval (CI): If a test’s 95% CI doesn’t overlap with the baseline, the change is significant.
Best SaaS A/B Testing Tools
Now, let’s talk about the tools of the trade. For something as critical to your SaaS operations as A/B testing, you’d also need an equally capable tool.
We have some good recommendations.
1.Fibr

Via Fibr
Fibr is the only tool you’ll ever need for A/B testing, be it for SaaS, your personal site, e-commerce, or something else. Why? Well, there are plenty of reasons why.
Fibr is designed to maximize your SaaS website’s potential with unrestricted experimentation. You are free to create, run and analyze unlimited campaigns across any pages, without worrying about session limits.
AI is the heart of Fibr’s platform. Surely enough, it carries over to A/B testing. Along with the intuitive WYSIWYG editor, you also get AI-powered suggestions for copy variations to improve your experiments.
Oh, and the AI also generates multiple high-converting variations for your webpage, saving you hours of tedious work.
We haven’t even talked about its best feature—MAX.
MAX is your AI-powered experimentation partner. He can find hidden patterns in historical data, user behavior, and trends to build data-driven hypotheses.
MAX is also adept at automation. He sets up experiments, configures elements, and analyzes results, basically automating the entire process.
Fibr is your best bet for smart, adaptive, data-driven A/B testing at wallet-friendly prices.
Best features
Run AI-powered A/B tests that easily target experiments to specific audience segments.
Create landing pages in bulk and generate multiple variants simultaneously.
Automate the entire testing process with the dedicated experimentation agent MAX.
Generate multiple high-converting suggestions to enhance your website's performance instantly.
Integrate easily with Google Analytics 4 to track campaign results, attributed revenue, and experiments alongside all your visitor data.
Pricing
Starter plan: $239/month for up to 50,000 visitor sessions
Starter plan: $479/month for up to 2,00,000 visitor sessions
Enterprise plans available on demand
2.Optimizely

Via Optimizely
Optimizely is a well-known A/B testing and experimentation platform made primarily for enterprises. It enables SaaS companies to test and personalize digital experiences at scale.
With Optimizely, you can run server-side and client-side experiments, ensuring you optimize not just your marketing pages but also your app’s core functionalities. Its Feature Experimentation tool lets you test features before full rollouts, reducing the risk of deploying changes that might negatively impact user experience.
The platform also offers statistical rigor through multi-armed bandit testing, which dynamically allocates traffic to better-performing variants in real-time.
Best features
Benefit from full-stack experimentation across web, mobile, and server-side applications.
Get advanced statistical models for reliable experiment conclusions.
Use the multi-armed bandit testing for real-time traffic optimization.
Robust API integrations with data warehouses and analytics tools.
Enable Feature flagging for controlled rollouts and gradual deployments.
Pricing
Custom pricing
3.VWO

Via VWO
Next up on our list is the crowd-pleasing VWO: a comprehensive experimentation and conversion optimization platform for both small businesses and large enterprises. VWO brings visual and code-based testing for A/B, split, and multivariate experiments.
VWO’s SmartStats (Bayesian-powered statistics engine) is a boon for faster and more reliable results by reducing the chances of false positives. It also includes session replays, heatmaps, and funnel analysis to help you understand user behavior before setting up experiments.
Best features
Run no-code A/B tests with the WYSIWYG editor.
Accurately interpret test results with the SmartStats Bayesian engine.
Track user behavior with heatmaps, session recordings, and funnel analysis.
Deliver targeted experiences based on user segments with the Personalization engine.
Get smooth integrations with analytics and marketing tools.
Pricing
Free plan available
Growth plan: $275/month billed annually
Pro plan: $633/month billed annually
Enterprise plan: $1,107/month billed annually
4.Convert

Via Convert
Convert is a developer-friendly A/B testing platform with a strong focus on full-stack experimentation. It provides advanced targeting and segmentation options, which makes it a great fit for SaaS companies that need precise audience testing.
With flicker-free testing and a lightweight script, Convert ensures that page load speeds remain fast—an important factor for SEO and user experience. It also supports server-side experiments, allowing teams to test deeper application logic beyond UI changes.
You also get to build better products with feature flags and rollouts
Best features
Get privacy-first A/B testing with GDPR and CCPA compliance.
Advanced audience segmentation for precise targeting.
Get full API access for custom integrations and automation.
Enable flicker-free experimentation for a better user experience.
Use server-side testing to experiment with backend features.
Pricing
Pro plan: $499/month billed annually
Growth plan: $299/month billed monthly
Enterprise plan: Request for pricing
5.AB Tasty

Via AB Tasty
Last, we have AB Tasty: an AI-powered experimentation and personalization platform built for SaaS, e-commerce, and media businesses. AB Tasty allows teams to create A/B tests, multivariate experiments, and feature rollouts without heavy engineering involvement.
One of its best features is predictive testing, which uses AI to forecast experiment results before they are completed. AB Tasty also includes a server-side testing suite, which is great for SaaS teams that need to test product features at scale.
Best features
Enable AI-driven predictive testing to anticipate outcomes before full test completion.
Feature flagging and rollouts for controlled deployments.
Personalize user experiences dynamically with the Personalization engine.
Integrate with analytics, CRM, and CDP tools.
Get mobile app experimentation for cross-platform consistency.
Pricing
Custom pricing
Test, Learn, and Climb Your Way to the Top
In SaaS, every decision, big or small, affects growth, retention, and revenue. A/B testing is your best bet against the evil of being in the dark. Just remember to test properly, track the right metrics, and let real user data guide your product evolution.
If you’re looking for a smart, AI-powered way to streamline your experiments, Fibr is your answer. With unlimited testing, AI-driven insights, and full automation, it takes the hassle out of experimentation so you can focus on what matters: building a product that wins.
Book a demo today.