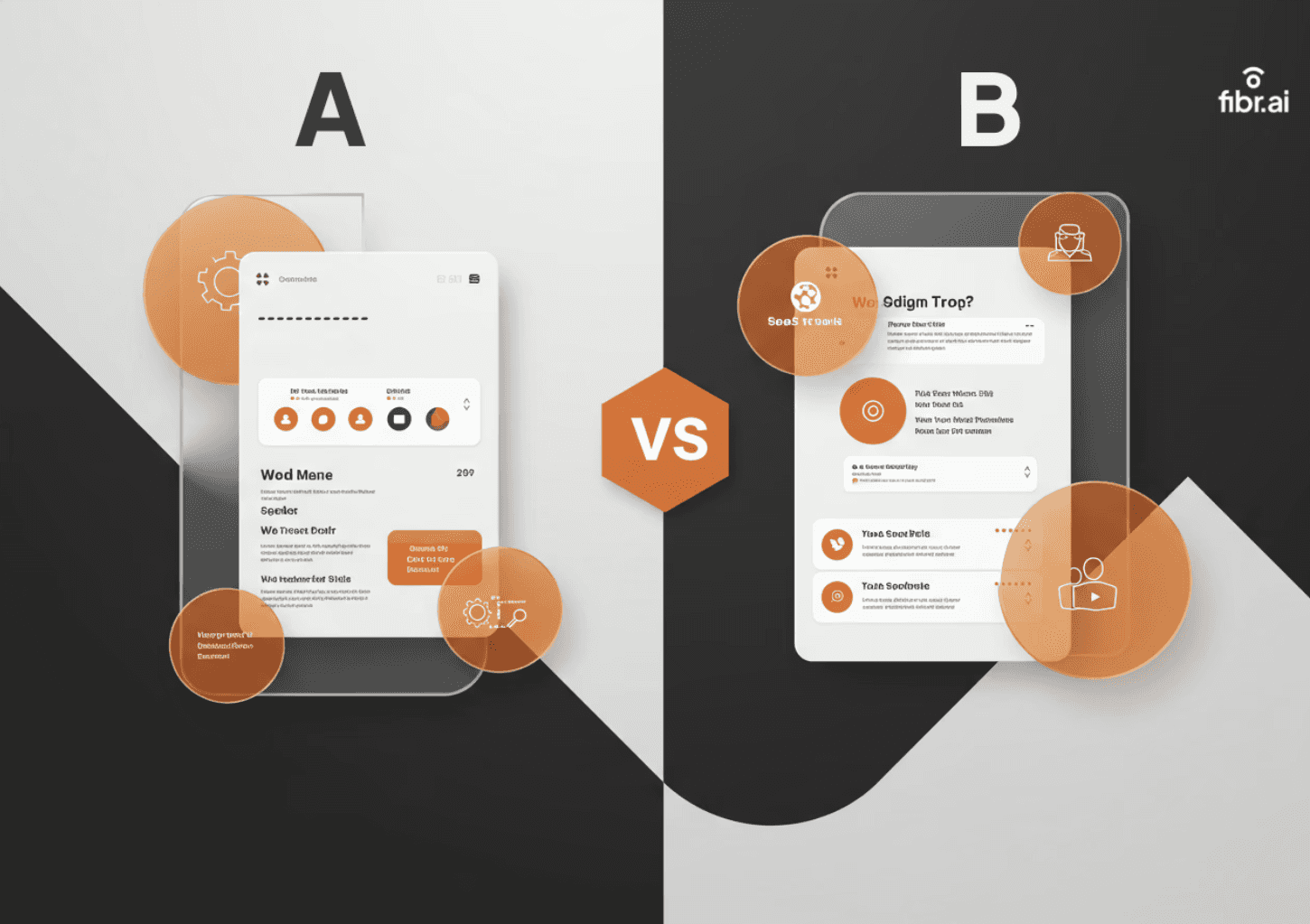
Unsure of why you’re A/B tests aren’t yielding the right results? Check out our list of 24 A/B testing mistakes to strike off possibilities one by one.

Ankur Goyal
As many as 77% of businesses today conduct A/B testing on their websites, with 60% performing it on their landing pages. Further, 58% of companies leverage it for Conversion Rate Optimization (CRO), and about 44% are dedicated enough to integrate A/B testing tools into their tech stack.
Related Read: CRO Strategy: How to Create an Effective Plan
This unique testing method that compares two versions of the same element (from whole landing pages to specific CTA buttons, color schemes, etc.) and is also known as split testing is a powerful technique to ensure you’re putting your best foot forward when it comes to wooing your customers.
That said, it is not free of its pitfalls—some of which can quite easily derail the entire exercise and bring your A/B testing efforts crashing down. Fortunately for you, we have you covered.
Here, we help you navigate this minefield by marking where the mines are buried and giving you a road map to get to the other sides. So, put on your safety suits, and let’s begin!
TL:DR:
Here’s a quick summary of what’s ahead:
Some of the most common A/B testing mistakes that you can make even before the test include testing the incorrect element, failing to align the test with business goals, running tests without a hypothesis, ignoring user segmentation, presuming users function in isolation, not involving your team in the tests, and focusing on incorrect metrics.
Further, A/B testing your landing page only, focusing on desktop traffic and ignoring mobile users, integrating mediocre testing tools, not attaining statistical significance, focusing too much on aesthetics, A/B testing elements that aren’t relevant, presuming testimonials always work, ignoring the role of external factors, prioritizing conversions over company personality, altering testing parameters mid-test, and not undertaking A/A tests are some mistakes to avoid during A/B tests.
Lastly, stay vigilant about post—A/B test mistakes such as not analyzing your results accurately, overestimating the impact of your changes, not recording your test learnings, skipping iterations, not staying vigilant about downstream impact, and marking an inconclusive test as a failed test are some common A/B testing mistakes that occur after the test.
Common A/B Testing Mistakes and How to Avoid Them
Common A/B testing mistakes before the test include testing the incorrect element, failing to align the test with business goals, running tests without a hypothesis, ignoring user segmentation, presuming users function in isolation, not involving your team in the tests, and focusing on incorrect metrics.
Some A/B testing mistakes during the test include A/B testing your landing page only, focusing on desktop traffic and ignoring mobile users, integrating mediocre testing tools, not attaining statistical significance, focusing too much on aesthetics, A/B testing elements that aren’t relevant, presuming testimonials always work, ignoring the role of external factors, prioritizing conversions over company personality, altering testing parameters mid-test, and not undertaking A/A tests.
Lastly, not analyzing your results accurately, overestimating the impact of your changes, not recording your test learnings, skipping iterations, not staying vigilant about downstream impact, and marking an inconclusive test as a failed test are some common A/B testing mistakes that occur after the test.
Common A/B Testing Mistakes Before the Test
Here are some A/B testing mistakes that can take place before the test and how to avoid them:
1.Testing the Incorrect Page or Element
A/B testing is a time and resource-intensive undertaking, which means that if you select the wrong landing page or element to test, you’re making a costly mistake. You see, if you choose a landing page that receives low traffic for A/B testing, pondering and testing whether changing a CTA button’s design, placement, or color will improve its performance won’t yield any jaw-dropping results.
In other words, you would have spent testing a page that was, anyway, not positioned to bring you the results you were hoping to achieve with A/B testing.
How to Avoid It: Do your ground research and identify high-impact pages or elements. Collect data on what nudges users to take action, whether it's lead generation forms, the checkout process, or a specific offering’s page. Use this to help select what gets tested. Because at the end of the day, if your page already gets negligible traffic or doesn’t really have a role in conversions, chances are it's not going to yield much after testing.
2.Failing to Let Business Goals Lead the Way
Running A/B tests without keeping a larger business goal in mind is like courting the devil. After all, if your tests are trying to improve a small element without taking revenue, customer retention, or customer acquisition into account, you’re working in isolation—the results of which will fall flat once you get to the real world.
Let’s take an example for better clarity—let’s say your business goal is to drive conversions. In such a case, testing the placement of your visual elements on the page might improve engagement, but it will have little effect on the conversion rate (as compared to, say, a CTA button or testimonials). Without a strategy and a goal in mind, you could be testing elements that perform in a vacuum but not on the ground.
How to Avoid It: Ascertain whether the test addresses a business challenge, whether enhancing it will have measurable gains, and the like to ensure your test connects with your overall business goal.
3.Undertaking Testing Sans Hypothesis
A hypothesis is a prediction about how a tweak to a specific element or landing page will impact its performance. It effectively addresses what you’re trying to enhance, why it would enhance, or how you’ll measure the said enhancement.
If you run tests without a hypothesis, you are just throwing things at a wall and looking for what sticks.
How to Avoid It: Ascertain the issue with what you’re testing and the opportunity for improvement. Now, leverage data from your reports, heatmaps, user feedback, etc. to create a hypothesis. Your hypothesis should read something like—"We believe that tweaking the X variable to the Y color scheme will improve the conversion rate.” Further, consider Fibr’s Experimentation Expert—Max, for the task. Max recognized areas that needed improvement and created automatic hypotheses like “Adding an image can help improve engagement.”
4.Forgetting User Segmentation Plays a Role
One of the most common A/B testing mistakes is presuming all your users behave the same way. After all, a new visitor won’t behave the way a repeat customer will. Similarly, in the case of an email campaign, a Gen X customer might find customer testimonials more convincing, while a Gen Z customer might first explore your social media presence.
How to Avoid It: Segment your audience based on shared characteristics, such as demographics, location, user preferences, and device type. Doing this will help you learn how different user segments behave with the tweaked variable and make the correct decisions for every segment.
5.Presuming Users Function in Isolation
A/B testing typically functions with the assumption that users aren’t talking to each other. But you know it as well as we do: users do interact with each other, sharing their experience, making recommendations, etc., which influences their behavior. And ignoring this fact can lead to misleading test results.
How to Avoid It: Consider leveraging network A/B testing to factor in group interactions. First, separate the test groups (create different communication channels or environments) and use tools to help measure group interactions (this will help you tweak your approach). Alternatively, if you can’t separate your users, take social influence into account when analyzing your results. You can also monitor social platforms to understand where your test is being discussed to learn users are influencing one another.
6.Running Tests Without Your Team
Failing to involve employees across departments and verticals means willfully turning away from ideas and suggestions that could help you drive the impact of your results. Further, your test could impact other marketing areas and sales activities. If employees managing these areas aren’t in the know of the tests you’re conducting, it could lead to confusion and issues that they’re not prepared for.
How to Avoid It: Prioritize cross-team collaboration by engaging employees across product development, design, content marketing, SEM, etc. Further, it helps them understand the A/B testing process and encourages participation.
7.Focusing On the Incorrect Metrics
The next A/B testing mistake is to focus on the wrong metrics, which can lead to misleading test results. Let’s say you’re testing the layout of your checkout page. In such a case, focus on the number of successful orders rather than page viewer density.
How to Avoid It: Ascertain a specific metric that directly evaluates the result you want. Now, choose other metrics that support this metric. After this, all you have to do is validate that these metrics reflect true customer behavior and not surface-level engagement.
Common A/B Testing Mistakes During the Test
Here are some A/B testing mistakes that can take place during the test and how to avoid them:
8.A/B Testing Your Landing Page ONLY
Don’t limit yourself to landing pages when A/B testing. Going full steam ahead when optimizing them but not analyzing and improving what comes next is a pointless exercise that doesn’t really yield any long-term benefits. After all, if users are engaging with your landing page but not really making any purchases, it's all for nothing.
How to Avoid It: Take a step back and evaluate your entire customer experience. Evaluate sign-up forms, cart pages, order tracking pages, etc., and work to improve the entire customer journey.
9.Focusing on Desktop Traffic and Ignoring Mobile Users
63.31% of all internet traffic comes from mobile phones, while only 36.69% comes from desktops. In other words, you’re losing a chunk of your visitors if you’re optimizing landing pages and elements only for desktops.
Your desktop design is not going to work for mobile phones considering the difference in screen size and actions (taps instead of mouse clicks and swipes rather than scrolls).
How to Avoid It: Segment your users based on device type and run A/B tests separately. Make sure your final design works for mobile users, and check if iterations work across devices.
10.Integrating Mediocre Testing Tools
If you use poor A/B testing tools, you can’t hope for stellar insights or results. After all, would you rely on a calculator that gives wrong answers? Well, the same goes for A/B testing tools.
How to Avoid It: Integrate Fibr’s Experimentation Expert—Max. This AI-powered tool runs reliable A/B tests 24x7 to help you optimize every last aspect of your landing page without the usual headaches and second-guessing. Not only that, but it generates seamless hypotheses and makes data-backed optimizations to ensure long-term success.
11.Not Attaining Statistical Significance
Statistical significance refers to the likelihood that variations between two versions aren’t random but, in fact, genuine and error-free. Let’s say you run an A/B test and find that one version of the element is performing 20% better after a week and take that as an indicator of discontinuing the test. That’s where the A/B testing mistake takes place—shutting the test down before it has gathered enough data to make an educated conclusion.
How to Avoid It: Implement a statistical significance of 95% or more. Further, ensure you work with a large enough sample size before completing the test. After all, a test with 50 users isn’t enough to help you recognize reliable trends.
12.Focusing Too Much on Aesthetics
Yes, aesthetics matter, but they’re not the only thing that matters. It's easy to fall down the rabbit hole of making your elements and pages visually appealing, but that’s just half of the story. The other half is value. You see, a beautiful landing page can do only so much before a user realizes they’re not gaining anything of true value.
How to Avoid It: Keep the focus on the value and the copy—make it compelling, strong, and impactful. Then, create visual elements to support it. Prioritize functionality over aesthetics as well. For instance, if your visual elements are heavy and slow down the landing page, you need to lose them.
13.A/B Testing Elements That Aren’t Relevant
Believe it or not, tweaking the color scheme of your image’s border might not affect your conversions. So, spending time A/B testing that—wasted! This is because not every aspect of your page requires testing; sometimes, the element is just not relevant to user behavior. And if you go optimizing the wrong element, you’re risking putting hours into something that won’t really give you anything in return.
How to Avoid It: Identify elements that influence behavior (CTAs, pricing sheets, headlines, images, etc.) and then ask yourself—“how much will it affect my overall business goal?” if your answer is not “significantly” or better yet “a bucket load,” it's not the one.
14.Presuming Testimonials Always Work
71% of customers trust businesses more after reading positive customer testimonials. Yes, customer reviews are powerful and can nudge new customers to a purchase. But they don’t guarantee it. And so another common A/B testing mistake is to presume adding testimonials to your page will automatically boost engagement and conversions. You see, even elements as successful as testimonials must be tested.
How to Avoid It: Don’t show favoritism to testimonials. Test them like you would other elements. Try different layouts, placements, and styles to understand what works best for your customers.
15.Ignoring the Role of External Factors
External factors such as weather, the festive season, public advisories, or even viral social media posts can sometimes affect your test results. For instance, let’s say you’re testing CTA buttons and notice a jump in conversions. You get excited but then realize your sales of umbrellas have increased because the weather forecast predicts heavy showers in the next few days.
How to Avoid It: Keep external factors in mind when testing, and if possible, run the test after the specific external factor nullifies to gain a clearer picture.
16.Prioritizing Conversions Over Company Personality
In the quest for higher conversions, you can sometimes lose track of your business’s unique personality. You see, being too focused on a business goal can make you disregard what makes your business stand out, leading to an inconsistent customer journey and confused users.
How to Avoid It: Balance optimization for the business goal with your business’s unique voice and personality. If a specific iteration works but contradicts your business’s tone, it's not the one for your landing page, no matter how successful it is.
17.Altering Testing Parameters Mid-test
You might be tempted to make adjustments to your test parameters while running it, but that’s a one-way ticket to unreliable test results. After all, think about it, if the test doesn’t say constant, how can the variables be tested equally every step of the way?
How to Avoid It: There’s only one way: define your test parameters and don’t divert from them. If you must, start a new A/B test.
18.Not Undertaking A/A Tests
You’d be surprised to know that you need to test your A/B testing tool before you allow it to test your variables. How do you do it? With A/A tests. This method tests two identical versions of the element to confirm the tool is working optimally.
How to Avoid It: Integrate Fibr’s Experimentation Expert—Max, and say goodbye to ever having to second-guess your AB testing results again. By creating suitable hypotheses to ensure your test has a guiding light and ensuring tests get conducted continuously, Max effectively ensures the integrity of your A/B test results.
Common A/B Testing Mistakes After the Test
Here are some A/B testing mistakes that can take place after the test and how to avoid them:
19.Failing to Analyze Your Results Correctly
Misunderstanding your A/B test results opens the door to inaccurate takeaways, errored conclusions, and, most importantly—unoptimized decision-making. This is especially possible when data is not analyzed correctly.
How to Avoid It: Leverage clear data analysis methods and consider seeking expert advice to navigate your way around your conclusions. You can also use charts, graphs, and other visual data representations to digest data more easily.
20.Exaggerating the Impact of changes
The next A/B testing mistake is to overestimate how impactful changes will be. This can cause you to set unrealistic expectations and cause disappointments. While it is a slippery slope to assume a favorable test result will be the key to your business goal’s metaphorical lock, you must take care not to fall victim. The impact of changes driven by positive test results is often modest rather than earth-shattering.
How to Avoid It: Study your A/B test results thoroughly and set realistic expectations. Further, be sure to iterate it multiple times before going full steam ahead and scaling efforts. Lastly, monitor and measure results to ensure you’re running the best version of the element.
21.Not Recording your Test Learnings
Not noting down your A/B tests can mean running in circles. You keep repeating the same mistakes, and you keep missing out on the same opportunities. After all, without meticulous records, you can’t possibly track what you tested, why you abandoned a specific approach, or why you doubled down on another.
How to Avoid It: Create and maintain a testing record. Note down every hypothesis, insight, result, and learning, including the subsequent steps you took after a test. This will soon evolve into a resource that can help you conduct quicker optimizations.
22.Skipping Test Iterations
Yes, A/B tests are time-consuming. No, unfortunately, one is not enough. Once you find a top-performing version, you must run it through A/B testing again in order to fully optimize it. You see, without an iteration, you risk adopting a version of the element that still has more potential for performance.
How to Avoid It: Allow for follow-up tests after initial results to get an opportunity to further improve the element being tested. Take an iterative angle to your A/B testing initiatives and keep testing versions until you find no room for improvement.
23.Not Staying Vigilant About Downstream Impact
Tweaks and iterations that improve one metric can do the opposite for another. Always consider the overall impact an iteration will have on your page’s performance before implementing it.
How to Avoid It: Track the downstream effects of your A/B test results. Double-check that implementing the A/B test results’ recommendation doesn’t compromise one area for improvement in another.
24.Marking an Inconclusive Test as a ‘Failed’ Test
‘Inconclusive’ is not the same as ‘failed.’ Interchanging them as if they’re the same is the last A/B testing mistake on our list. You see, when conducting A/B tests, you’ll get two kinds of results—impactful and inconclusive. But that doesn’t mean ‘inconclusive’ must head for the dustbin.
How to Avoid it: Change your perspective on ‘inconclusive’ test results. Think of them as indicators or what you should avoid. If nothing else, they’ll help you get to your best element version through omission. Use them to understand factors that don’t have much of a bearing on your business goals.
Conclusion
And that culminates our list of the top 30 A/B testing mistakes to avoid (and how) in 2025. However, you know it as well as we do, sidestepping these very easy mistakes is no easy task. What you need is a handy tool that automates the exercise of optimizing your landing pages and websites with a click of a button.
This is what you find with Fibr. Powered by multiple advanced AI agents that automatically manage experimentation, personalization, and performance optimization, this tool is a game-changer for your CRO efforts.
In particular, it's Experimentation Expert—Max. Running 24x7 and an addict for optimization, this Fibr offering does everything from hypothesis generation and ensuring your website is always improving to furnishing data-driven results that drive your overall ROI.
Make smarter decisions faster with Fibr’s expert—Max. Book a demo today!