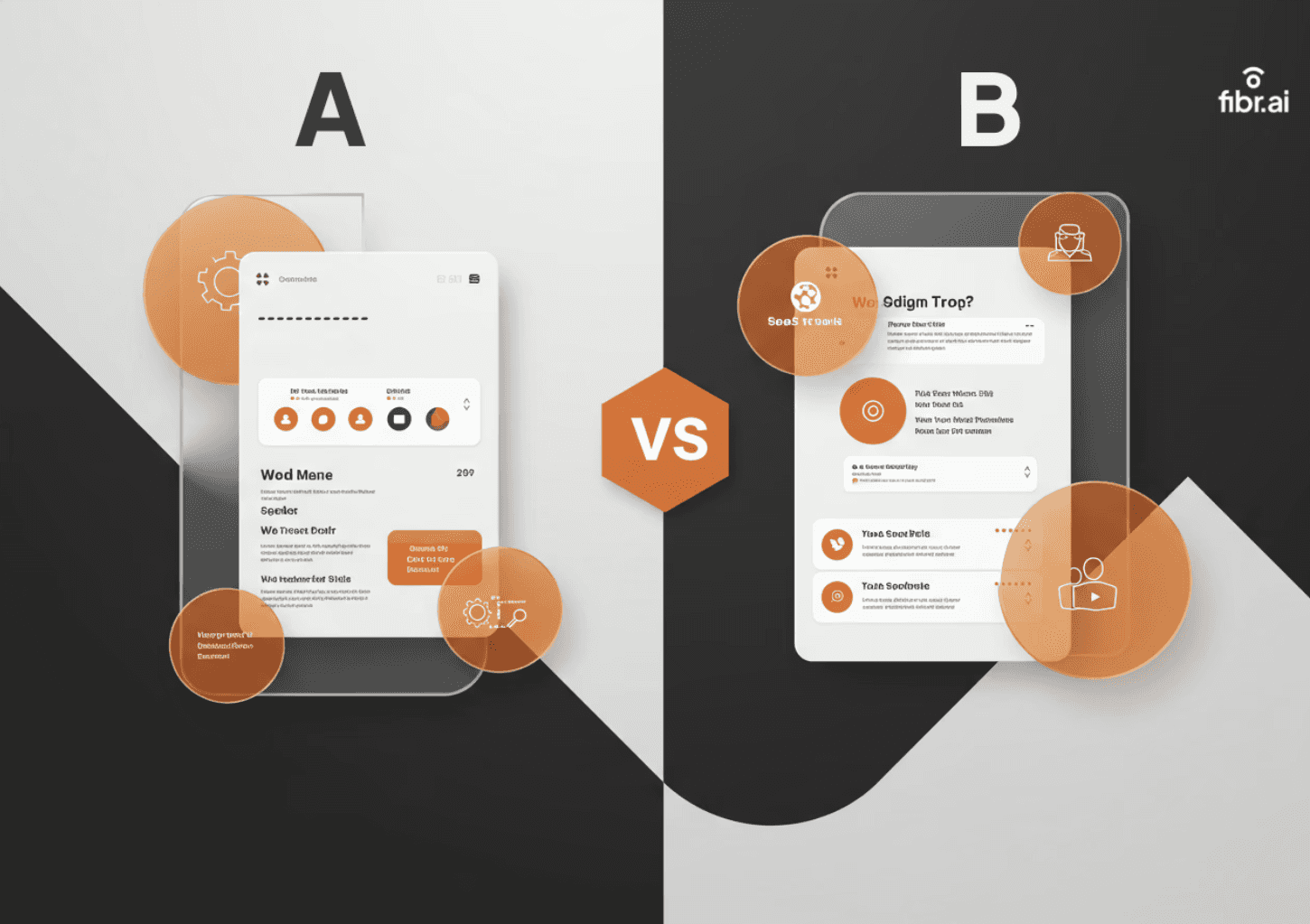

Pritam Roy
Imagine you’ve introduced new products or features and want to create the perfect landing page for them. You have sorted out all the elements, from the navigation flow and CTA buttons to the color scheme and typography.
Now, you design a page that you think will suit your brand. But one important consideration is amiss: how will you ensure your audience likes it enough to engage?
You need real first-hand data to answer this, not assumptions — and that’s where A/A testing and A/B testing come in handy.
First, you run two different variations to see which one gets a better response. But how do you know it’s actually going to work for most (if not all) of your customer segments? You will have to compare two identical versions of the same webpage in two splits of audience through A/A testing.
Songs overwhelming? We have your back.
Below, we’ll dive deep into A/A testing vs A/B testing, when and why they are important, and how you can use them both:
What is A/B Testing?
A/B testing, also called split testing, is a quantitative research method where you test two or more variations of a design or digital asset and see which one gets a more positive response from the audience.

You show version A (the control) and version B (the variant) to two random audience segments simultaneously. Then you track KPIs like click-through rate, bounce rate, conversion, and more and see which variation performed better.
A/B tests are a popular method is assessing the relevance and appeal of:
Webpage layouts
CTA buttons and placements
Headlines
Web Copy
Marketing emails
Pricing and offers
Purpose of A/B Testing
Here is why it should be a priority:

1.Making data-driven decisions
According to studies, companies that make data-backed decisions drive 5% more productivity and 6% more profit than their competitors.
A/B testing lets you do that for your web page designs as well. You are just not adding elements and hoping your audience will interact. Instead, split testing offers measurable evidence of what your audience will actually like.
2.Reducing uncertainty
Want to redesign a webpage to improve user experience or create new landing pages for new features? It’s a big undertaking. One mistake and all your efforts and money go down the drain. Plus, irrelevant changes may just end up hindering user experience, causing churn and lost opportunities.
A/B testing allows you to test out small changes among the audience and see how they are receiving it. You can make improvements on a smaller scale, gauge the audience’s reaction, and then finalize all elements of the webpage. This eliminates uncertainty, ensuring the new user experience and interface resonates with the users before you finalize it.
3.Understanding your audience
The audience has constant exposure to new trends and products. So, their preferences change rapidly. For any business to stay competitive, adapting to these fast changes is crucial. Otherwise, your audience won’t hesitate to jump ship with your competitor.
In fact, a PwC report revealed that 59% of customers will change brands after several bad experiences, while 17% will do so after just one bad experience. That’s not all. One in three customers are willing to walk away from brands they love because of one bad experience!
As you can see, keeping up with your audience’s preferences, needs, and challenges is non-negotiable for long-term success — and A/B testing gives you that path.
Running these tests regularly keeps you updated with the customer’s evolving likes, dislikes, behaviours, and needs. Since you get data on how users interact with different versions of products or webpages, you understand them better and apply this knowledge in personalizing their experience.
4.Improving performance metrics
A/B testing data lets you lay down a tangible roadmap to achieving targeted business goals. You get to test different versions of your digital assets and choose the one that drives the best results. This way, when you launch the winning variation officially, you have better chances of improving metrics such as more click-throughs, lower bounces, and boosted conversion.
Say you run an online store and test two versions of your product page. One has customer reviews (Version B), and one doesn’t (Version A). A/B testing showed that Version B drove a 20% increase in conversions. So, when you implement it site-wide, you can safely assume that overall sales and other performance KPIs will increase.
Common Elements Tested in A/B Testing
Confused about which aspects to compare through A/B testing? Here are the most common ones to get you started:
1.Headline
You have only a few seconds to grab a visitor’s attention, and you must make it count with a compelling headline. It has to communicate how your brand can help them. But is it resonating with their needs?
You can create 15 to 20 different versions of your web page headline copy and run A/B tests. Shortlist 3 to 4 best-performing ones. Then you start ensuring whether your headline’s aesthetics are right by including split variations like:
Color: Combination of contrasting colors against the background to test visibility
Text size: Large fonts vs medium fonts
2.CTA
The right headlines impressed your visitors and made them explore more. Now, a persuasive CTA button can turn a casual visitor into an interested lead. That’s why, you must be sure that your call-to-actions can actually convince the audience to convert, whether they are on your webpage, ads, or emails. Here is what you want to test in your CTAs:
The copy on the button
Button placement
Visibility against the background
3.Visuals and texts
For some users, having too many videos on the webpage may feel overwhelming. Some may look for more text-based explanations of what your product does so that they can skim through quickly. Run A/B tests to understand what works for your target audience:
Product demo on home page vs product demo on landing pages of every feature
Image placements
Variations of text copies
4.Pricing
How you frame your pricing is a big deciding factor in whether someone will opt for your product or not. A/B testing will get you the data on what pricing structure fits your customer demographic. Here are some splits you can try:
Monthly or annual subscription
Discount or money-back guarantee
Listing all associated features of a plan or only highlighting the primary ones
Per user or a set number of user structure
5.Color scheme and typography
Colors influence emotions and actions. They also play a big part in making your brand memorable. For readability and engagement, you must also choose the font style and size carefully as well. For that, here are some elements you must A/B test:
Light vs dark themes to test ease of navigation
Line spacing and letter spacing to test readability
Font type and size to see which improves brand recognition
Combinations of background and font color to see which keeps the visitor engaged for longer
To analyze so many elements accurately, you need a reliable A/B testing tool that lets you collect and analyze split test data and implement them properly — that’s where Fibr AI can help you.

Our platform lets you conduct A/B testing effortlessly. Fibr AI comes with an AI experimentation engine by the name MAX - creates hypotheses and continuously conducts experiments to maximize conversions.
Now, let's talk about the ideal times to run A/B tests:
1.Before major redesigns
Planning major redesigns? Run split tests to ensure you are adding the right elements before changing all your web pages or app experience. This will help you avoid performance drops. Make sure you test all elements, from navigation to test sizes.
2.Before launching new features
You may add a really innovative feature to your product and still see performance metrics going down. Why? The audience may have found the feature irrelevant or a hindrance to their experience.
So, before the official launch, run A/B tests on feature placement, descriptions, functions, and marketing campaigns to gauge effectiveness.
3.Seasonal campaigns or events
You can expect a significant rise in traffic during seasonal campaigns. Run A/B tests beforehand to ensure your web pages are optimized enough to handle sudden traffic surges.
Events are excellent tools to create short windows of high engagement and build credibility along the way. A/B testing and fine-tuning your event page ensures visitors will have a smooth registration process, maximizing participation.
4.Before launching an email campaign
Nobody wants their emails to end up in spam folders. Plus, you need the recipient to open the email and click on the CTA button.
So, before launching any email campaigns, test elements like subject lines, visuals, email copies, and CTAs to ensure your approach is effective.
5.Before increasing the marketing budget
Got some really good results from your recent marketing campaigns? You may feel a little too excited to scale it in the hopes of more profits.
But the smart thing to do here is to split-test your newer ad copies and creative campaigns on a smaller budget. If you get a good response, then you can allot more funds into those campaigns.
Pro Tip: Make it a routine to run A/B tests during high-traffic windows. You will get an extensive audience base to test the variations and get more detailed and conclusive insights.
Handling so many A/B tests, that too regularly, sounds intense, doesn’t it? With Fibr.AI, it doesn’t have to be.

Our AI Agent Max will continuously scan your web pages and find hotspots where your audience is engaging the most. It analyzes user behavior, detects interaction patterns, and refines tests in real-time. So, no need to worry about setting up individual A/B tests!
Key metrics to evaluate in A/B Testing
Here are the CRO metrics you should be measuring through split tests:
Conversion rate: Usually the primary goal of most A/B tests, this metric will tell you which variant encourages the most number of desired actions from visitors.
Click-through rate: This metric quantifies how effective the changes in headlines, buttons, or design elements will be. Higher CTR shows that the variant is persuasive enough to drive clicks.
Bounce rate: A lower bounce rate shows that users are engaging more because the test variant is keeping them interested.
Revenue per visitor/ Average order value: This KPI helps you go beyond the conversion rate and understand whether the changes in web design and copy led to higher spending.
Average Session Duration: A higher session duration shows that visitors are finding your content and product pages valuable. This KPI is particularly useful when you are testing layout changes, content restructuring, or navigation tweaks.
Pages per Session: If a visitor views more pages for a particular variant, it shows that they can navigate your site easily and are interested enough to explore more.
Time on Page: If one variant lowers your general time on the page, it could mean that its content isn’t relevant enough for the visitors. It could also indicate that the design had too many friction points for them to engage properly.

Don’t want to spend time on never-ending calculations? Fibr.ai’s experimentation agent Max will suggest your optimization hypothesis automatically. It runs tests in the background so that you always get the most accurate suggestions.
What is A/A Testing?
A/A testing is a data reliability assurance process where you split your traffic into two parts and run each through identical experiences.
You will be restructuring your web pages according to A/B testing data. It only makes sense to double-check the accuracy, right? How do you do that? Through A/A testing.
The A/B test showed you the variant that drove better results. A/A test lets you determine whether the results are reliable by testing visitor responses on two same experiences.
Suppose the A variant in your split test drove more conversions. Your goal here is to determine if there is any difference in metric improvements between the identical experiences.
Purpose of A/A Testing
A/A testing may feel redundant at first. But it actually serves a crucial purpose in making data-driven changes in your user experiences, like:
Creating a baseline
A/A tests let you set up a baseline conversion rate. It detects conversion metrics through two identical versions of an element. This shows you the benchmark you can use to measure results from your future A/B tests.
Validating A/B testing
Did the winning variant really impact the rise in conversion metrics? Or did natural variance cause the detected fluctuations? A/A tests let you clear such doubts. It confirms that your testing tool, data collection methods, and analysis processes are working properly.
Identifying Technical Issues
It can reveal potential problems with your testing platform, randomization algorithms, and data tracking. These can otherwise lead to inaccurate A/B test results. A/A tests can help also identify potential inherent biases in your testing methodology that might mess up your results.
Common Elements Tested in A/A Testing
You can run A/A tests in these elements:
Randomization logic
You can use A/A testing to see if the winning variant performs the same with random user groups without any patterns or bias. If the random assignment fails, it shows that your A/B testing method data isn’t accurate enough.
Page load time and performance
A/A tests ensure both traffic groups experience similar page load speeds. This detects any possible performance inconsistencies affecting user experience.
When to Run A/A Testing
A/A tests only generate the right results when you run them at the right times. Here are some instances when A/A tests are a must:
Before investing in new A/B testing tools
Thinking about investing in a new A/B testing tool? Get the trial version first and then run the A/A test on the results. It verifies whether the tool is working properly and is collecting accurate data. That way, you won’t get stuck with an unreliable A/B testing tool.
After A/B testing setup changes
Validating A/B testing results for each element is a good practice to eliminate the possibility of data inaccuracy. So, once you get your winning variant, run it through A/A testing to be sure of it’s effectiveness.
If you notice data inconsistencies
In case you notice big inconsistencies in your A/B testing results and analytics data, it can be a hint to performance issues in your A/B testing system. A quick A/A test can help you identify and resolve them promptly.
Setting sample size
Running A/A tests before your A/B tests can help you understand the appropriate sample size needed for statistical significance in A/B testing results. So, make sure you create a baseline through A/A tests.
As a semi-regular routine
We suggest running A/A tests semi-regularly as a routine. It will ensure your testing tools are still functioning accurately.
Key metrics to evaluate in A/A Testing
Now, let’s talk numbers. Here are the KPIs you should be measuring with A/A tests:
Traffic distribution metrics: Messing this metric identifies if one variant is getting more traffic. You can detect imbalances in sample sizes.
Conversion rate: Significant differences between two identical experiences highlight issues in tracking setup and randomization flaws.
Engagement metrics: Differences in engagement metrics identify possible anomalies in on-page experiences, content delivery, or any other technical issues
Event consistency: This metric helps detect missing, double-counted, or delayed event
Page Load Time: Measuring this KPI ensures users are experiencing the same loading speed for the winning variant.
How A/A and A/B Testing Work Together
Both A/A and A/B tests have their benefits and drawbacks. But when you use one to validate the other’s results, it maintains data integrity, removing guesswork from the picture. Result? You create landing pages, launch features, and run campaigns that actually convert and retain users.
Choosing an AI-powered testing platform that lets you apply changes directly can help you get there — and that’s what Fibr.ai offers. With AI agents, you can run 100x more experiments 10x faster with no outside help.

For example, our experimentation agent, Max will scan your web pages and generate testing ideas in seconds. You can select the ones you like and automate tests directly from your dashboard. Our system will create variations in bulk and show results you can actually rely on.
So, what are you waiting for? Sign up with Fibr.ai, tap into the power of artificial intelligence, and run A/B tests with no fuss!
FAQs
1.What is A/A test vs A/B test?
A/A testing involves showing two identical versions of an element to check if both variations are getting the same response from the audience. It validates whether A/B testing and data tracking setup are accurate.
A/B testing, on the other hand, compares two different variations (variant A and variant B) against engagement and conversion KPIs.
2.Why is A/A Testing important before running an A/B Test?
A/A testing is important before running A/B tests because the former validates whether the testing setup is running accurately. It identifies potential gaps in your testing methodology so that you can address them and ensure correct A/B test results.
3.How long should an A/A Test run before an A/B Test?
You should run an A/A test long enough to collect a statistically significant sample size. Typically, companies run them for 1 to 2 weeks, depending on their website website traffic.
4.What are the limitations of AA Testing?
Some limitations of A/A testing are:
It doesn't evaluate new ideas or improvements but only validates the testing setup and data accuracy.
The process can be cost and time intensive