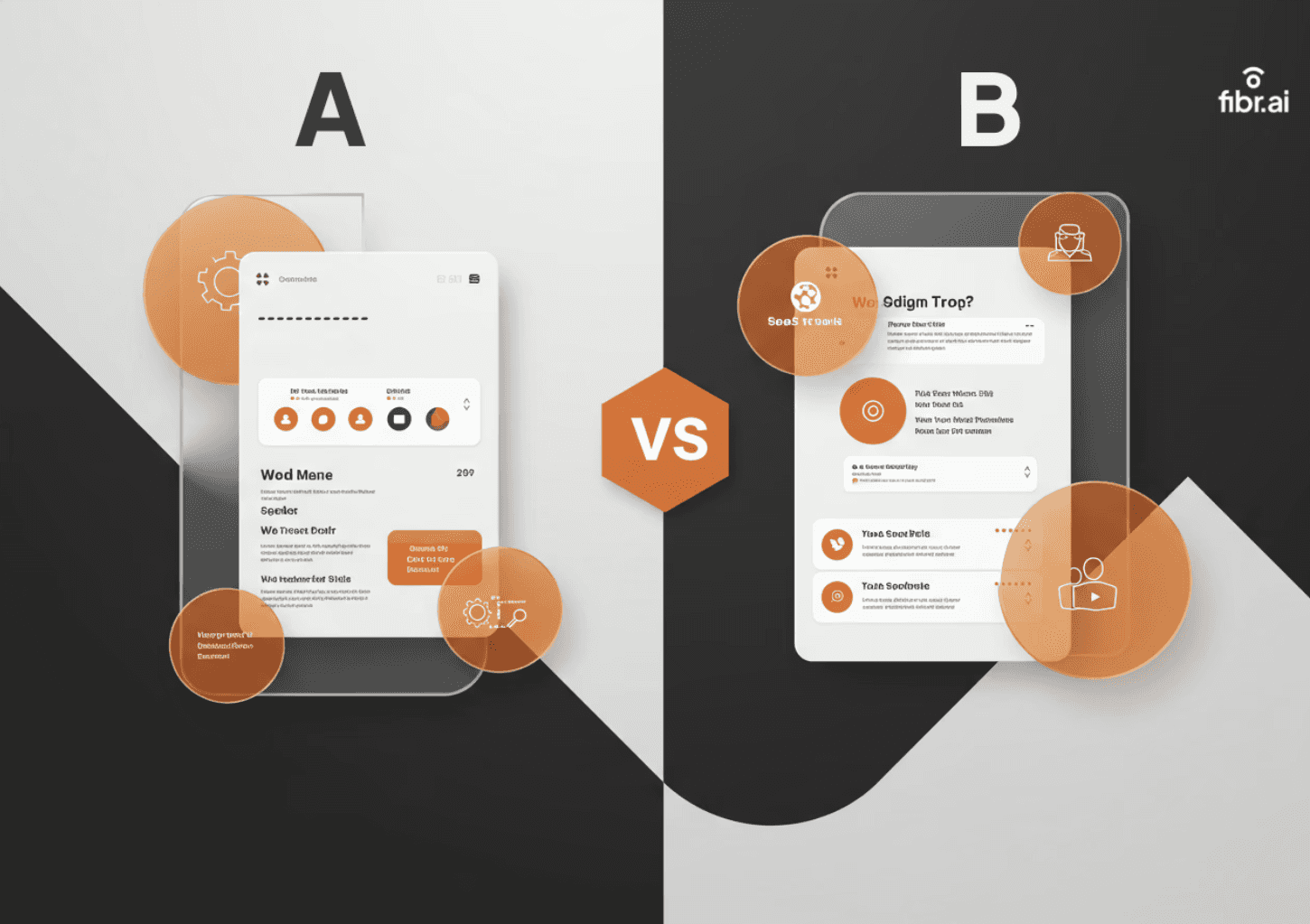
Discover how A/B testing for ads can help optimize your campaigns. Learn key elements to test, best practices for different platforms, and more.

Pritam Roy
What's one common strategy in every marketer's playbook? Running paid ads.
It is a quick and simple way to:
Get yourself in front of your target audience
Create demand for your product
Boost sales
Whether you're running Google ads or targeting platforms like Facebook, Instagram, or LinkedIn, clever advertising can help you connect with potential customers where they are.
But running ad campaigns goes beyond selecting a platform, creating a catchy headline, and setting a budget. You need to ensure they click with your audience and fulfill their purpose.
And the best way to do this is through A/B testing for ads.
In this comprehensive guide, we'll dive into everything you need to know about A/B testing for ads, including what it means, best practices, common misconceptions, and more.
Related Read: Google Ads A/B Testing: A Deep Understanding
Summary:
A/B testing for ads involves testing different variations of the elements to see what resonates the most with customers and encourages them to take the desired action.
The different elements to A/B test in ads include the headline, copy, CTA, visuals, and audience.
It's important to avoid common A/B testing mistakes, such as optimizing for the wrong audience, running experiments without a clear hypothesis, and changing traffic allocation mid-test.
To set up an effective A/B test for ads, start by defining your goals and creating a hypothesis. Then, create the variants, run the test, analyze the data, and finally implement the winning version.
What is A/B Testing for Ads?
A/B testing for ads is a marketing strategy that lets you compare two versions of an advertisement to see which performs the best. It lets you split your audience in two and show each group a different variation of the ad at the same time.
Say you're running a digital ad on Facebook showcasing a newly launched pair of sneakers. But you're confused if the ad should feature an image of the sneakers alone or of a person wearing them.
In this case, you can create two versions of this advertisement:
One with an image of just the sneakers
One with an image of a model wearing them
You can show both these versions to two different audience segments and track their performance to see which resonates with them the most. It is a sure-shot way of making practical, data-driven decisions without relying on guesswork. Moreover, AI-powered tools like Fibr AI's A/B testing agent Max help streamline this process by quickly analyzing performance data and identifying high-impact variations, so you don’t have to rely on guesswork.
Related Read: What is Facebook A/B Testing and Why Is It Important?
Common Misconceptions About A/B Testing in Ads
A/B testing your advertisements is a great way to ditch the guesswork and make decisions based on real data. But let's be honest - there's a lot of bad advice out there about how it works. From trying to test everything at once to expecting instant wins, here are some common A/B testing misconceptions you must be aware of to ensure you don't unknowingly sabotage your ad strategy:
1. Running A/B Tests for Ads Generates Instant Results
If only it were that easy! In reality, the A/B test isn't a magic wand that can wield results out of thin air. The results depend on data. And collecting good data takes time. Just because one variant gets a few clicks in the first 24 hours doesn't mean it's the winner. This trend can change over days or even weeks.
And so, you need to run the test for a longer duration to give it enough time to gain enough impressions and produce reliable data.
2. Once a Winning Variant, Always a Winning Variant
Had an ad that crushed it last quarter? Good for you! But that's no guarantee it'll continue working forever. Trends change. Customer preferences evolve. And even platform algorithms update. So why should you still rely on outdated results?
Think about it. You shared an irresistible holiday offer in December, and it was an instant hit among your audience. Would the same strategy work in, say, March? The key to staying relevant is to keep testing.
3. Testing Every Element, All at Once
Suppose you create two variants for a LinkedIn ad:
Version 1 has a shorter headline, a product image, and a bright-red CTA text.
Version 2 has a longer, benefit-driven headline, a user-generated image, and a QR code linked to the product page instead of a direct CTA.
Now, say the test results suggest Version 2 is the winner. How do you know which tweak made the difference? You don't.
Testing too many elements at once can cause confusion, which can lead to bad decision-making. Therefore, it's important to focus on one change at a time. Test your CTA. Then, test your image. Then, your headline. This will help you determine which version of these elements is driving the best results.
4. Any Time is a Good Time to Run A/B Tests on Ads
Timing is key when running A/B tests on ads. For example, testing during major holidays can skew results due to seasonal traffic. Similarly, if you're running a high-stakes ad campaign, you wouldn't want it to have an average performance for half your audience.
Remember, the results of your A/B tests will impact your meticulously crafted advertisement strategy. So, be strategic about when you test.
How A/B Testing Works for Ads?
When running ad campaigns, A/B testing helps you compare their performance, understand why one ad performs better than the other, and use this insight to keep improving. Here's how it works:
An A/B test for an ad starts by isolating a single variable. This can be the ad headline, image, or CTA.
Once you've narrowed down the element you want to test, you create two versions of it: one original, 'controlled' version and the other with the change you want to experiment with.
Then, you segment your audience into two, so each section sees one version of the ad.
The test should be run for a duration, ideally for around 10 days if you have 20,000 visitors per day, to get reliable results.
What Elements to A/B Test in Ads
With Max, you can run hundreds of experiments on various elements like:
1. Headline
Your headline must have a catchy hook to grab your audience's attention. It has the power to increase engagement even before users process the rest of your ad. You can experiment with different styles of headlines like:
Curiosity vs. Clarity: For example, 'The Secret to Doubling Your Sales' vs. 'Get 2x More Sales with This Strategy.'
Pain Points vs. Benefits: For example, 'Struggling to Find a Job?' vs. 'Land Your Dream Job in 30 Days.'
Question vs. Statement: For example, 'Tired of Searching for the Perfect Marketing Automation Tool?' vs. 'Save 30% on Marketing Automation Today.'
2. Copy
Once you grab the customer's attention with a strong headline, the next element at play is the copy or description. It needs to convince and convert your audience. But not every copy will work for every customer. You can experiment with different styles like:
Creating Urgency: For example, 'Last Chance! Prices Go Up Tonight!'
Hard Claims: For example, 'Proven to Reduce Costs by 30%.'
Conversational: For example, 'Are you tired of wasting money on bad software?'
3. CTA
Your CTA should tell people exactly what to do next. A weak or vague CTA can be ineffective, causing them to drop off. So, experiment with different options that align with your audience's mindset and nudge them to the next step. For example, your CTA could be:
Benefit Oriented: For example, 'Unlock Your Free Trial.'
Action-Oriented: For example, 'Sign Up for Free.'
Time-Sensitive: For example, 'Get 20% Off Today Only.'
4. Visuals
Visuals are the images or videos you include in your ad copy. They should be catchy enough to encourage users to stop the scroll and persuasive enough to make them want to click the ad. You can experiment with different visual styles, such as:
Static images
Videos and gifs
Bright/dark colors
Product images
User-generated images
5. Audience
Finally, when you're running A/B tests on ads, you can also experiment with your audience. After all, the people who see your ad matter just as much as what's in it. Experiment with different audience segments, demographics, behaviors, interests, etc.
How to Set Up an Effective A/B Test for Ads
Here's a step-by-step guide for how you can run A/B tests for ads the right way:
Step 1: Define Your Goals
Before you start testing, answer this question: What's the one thing you want to improve? More clicks? Higher conversions? Better brand identity?
Having a clear destination prevents you from wasting time on random experiments and detouring along the way. For example, if you want to optimize for clicks, you might want to focus on the headlines, images, and CTAs. Similarly, if you're optimizing for conversions, you might test different offers or landing page designs.
Step 2: Build a Hypothesis
Your hypothesis forms the base of your test. It is the statement that predicts the potential outcome of your experiment. A good hypothesis follows this structure: 'If I change X, then Y will improve because Z.'
For example, 'If I make my CTA button red instead of blue, my click-through rate will increase because red creates a stronger sense of urgency.' Avoid generating random hypotheses just for fun. Test elements that make sense based on audience behavior.
With Max, you can generate automatic, data-driven hypotheses based on your content, visuals, and goals.
Step 3: Create Variants
Once you've created a hypothesis, the next step is to create two versions of the ad to see which one works better. For example, if your hypothesis is about the CTA button color, Variant A might have a blue button, and Variant B will have a red one.
While you can run A/B tests on more than two variants, it is usually more complex, time-consuming, and requires way more data to get reliable results. So, select the number of variants accordingly.
Step 4: Run the Test
Start by splitting your audience evenly and randomly. Half will see Version A, and the other half will see Version B. Then, run the test for an adequate duration to get reliable results.
Avoid making any judgments based on a few hours' worth of data. Wait until you have statistical significance, meaning enough data to be confident in your findings.
Step 5: Analyze the Data
Once you've run the test long enough, it's time to analyze the results. Look at how many people engaged with your ad, how many clicks turned into sales, and if the results meet your initial goal.
For example, Say Variant B got more clicks than Variant A. But your goal was increasing conversions. If those extra clicks didn't lead to a sale, you might have attracted more curious people instead of buyers.
Step 6: Implement the Winning Version
Once you find a version that outperforms the rest, roll it out across your campaign. And once you're done, don't stop at that. Identify the next element you want to test to push the campaign's performance even further.
Best Practices for Running A/B Tests on Different Ad Platforms
A/B testing isn't a one-size-fits-all strategy. Whether you're targeting Facebook, Google, Instagram, or LinkedIn, each platform has its own requirements. But here are some practical A/B testing in ads best practices that'll help you get accurate, actionable insights, no matter which platform you select:
1. Test Only One Variable At a Time
It can be tempting to change everything at once - headlines, images, CTAs. It might also seem more efficient. After all, why run multiple tests when you can experiment with all elements in just one?
But this is a serious mistake. You see if you tweak multiple things at once, how would you know which change actually made the difference? Therefore, it's important to pick just one variable at a time. For example, if you're testing a new image, keep the copy and CTA the same. This will help you get clear, usable insights instead of just guessing.
2. Use a Large Enough Sample Size
Don't just declare a variant the winner if it gets more clicks after just 50 impressions. For A/B testing to be accurate and statistically valid, you need a big enough audience to make sure your results aren't just luck.
As a rule of thumb, wait for at least 1,000 impressions per variant before analyzing results. You can also use A/B test calculators to estimate the sample size you need for accurate results.
Related Read: A/B Testing Sample Size: A Definitive Guide for Beginners
3. Run the Test Long Enough
Stopping a test too early? You'll not get good enough data to make a clear judgment. But on the other hand, you can also not keep a test running forever.
The sweet spot is running it for at least a week or till you reach statistical significance (typically 95%). This ensures the results aren't just random, helping you make informed decisions.
4. Show the Ad to Similar Audiences
It's important to show your test variants to similar sets of audiences. Otherwise, it'll be more like testing different groups than testing the ad.
So, split your audience evenly and exclude past customers to get reliable results.
Analyzing and Interpreting A/B Test Results
Running an A/B test is only half the job done. The next, equally important step is to analyze and interpret the test results. Here's how you can do it:
Step 1: Look At the Big Picture
Before delving into the nitty-gritty, see if you have a clear winner. But we'll reiterate: make sure the test has run long enough and you have a substantial sample size. This will ensure your results are reliable and won't mislead your analysis. If you haven't met these two requirements yet, let the test run longer before making any decisions.
Step 2: Understand 'Uplift' and 'Probability to Be Best'
Before you dive into analysis, it's important to understand these metrics. Uplift tells you how a variation performed compared to your baseline (control). Although a higher uplift is better, you also need to consider statistical significance and consistency for a thorough analysis.
The Probability to Be Best, on the other hand, tells you how likely the winning version is to be the best choice in the long run. While most A/B testing platforms calculate this metric automatically, you can also use online Bayesian A/B testing calculators to estimate it.
Step 3: Analyze Secondary Metrics
Most marketers focus only on primary metrics, like CTR or conversions. Suppose your A/B test aims to increase clicks, and Variation B wins with a 20% higher CTR. But what if users clicked but bounced without engaging? Or the winning variation increased low-quality traffic?
That's where secondary metrics come in. These include your conversion rates, revenue per user, average order value, etc., and help you see the full picture before making any major decisions.
Step 4: Break It Down by Audience
A test that wins overall might not necessarily win for every audience segment. That's why you must also analyze performance based on other parameters like the traffic source, device type, new vs. returning users, etc. This will help you refine your approach and get the most value from your test.
Common Mistakes to Avoid in Ad A/B Testing
A/B testing is a powerful tool. But to get it right, you must avoid these common A/B testing ad mistakes:
1. Optimizing for the Wrong Audience
If you optimize your A/B tests for the wrong audience, you could end up with a winning ad that drives the wrong kind of traffic. For example, if you're selling high-end software, an ad that attracts free trial users who never upgrade isn't a win. So, segment your audience carefully and prioritize bottom-line metrics.
2. Not Having a Clear Hypothesis
Jumping into A/B testing without a solid hypothesis will only steer you away from success. You'll end up testing random elements without even knowing what you're looking for. So, leverage Fibr AI’s A/B testing agent, Max to create a strong hypothesis before you start testing.
3. Changing Traffic Allocation Mid-Test
Once your test is live, leave it alone! Tweaking traffic allocation during the test can throw off your results, leading to distorted data and unreliable conclusions.
Run A/B Tests At Scale with Fibr AI
So, that was our detailed guide on A/B testing for ads. But despite all the guidance, it can often be difficult to manage every aspect of the test while also managing other marketing campaigns. Fibr AI's A/B testing agent, Max, simplifies this.
It runs non-stop experiments, tests hypotheses, and refines your website around the clock so you can focus on more strategic tasks. Here's how it helps you:
Smart Hypothesis Generation: Max analyzes your website's content, visuals, and goals to create data-driven test ideas.
Always-On Testing: It runs continuous experiments to identify the best-performing variations without requiring any manual setup.
Data-Driven Optimization: It learns from every test and keeps refining your site for better conversions and engagement.
ROI-Focused: Every tweak and test aims to maximize revenue, not just improve surface-level metrics.
Let Max handle the testing so you can focus on growth.