A/B Testing

Pritam Roy
Are you wasting time on A/B tests that don’t deliver accurate, reliable results?
If so, you’re not alone. Every marketer knows the frustration: hours spent testing with no clear results to guide better decisions.
Fact: A/B testing can unlock your website’s potential by identifying what works best for your audience.
But here’s the catch—without the right A/B testing sample size, your results could be misleading, and cost you time, money, and opportunities.
In this guide, we’ll walk you through the entire process of calculating your A/B testing sample size.
Specifically, we’ll explore the following areas:
What is an A/B testing sample size?
Why is sample size important in A/B testing?
How to determine the right sample size for A/B testing
Steps for calculating AB testing sample size
Common challenges in A/B testing sample size 600
Best practices for managing sample size in A/B testing 700
Let’s dive in and ensure your next A/B test delivers accurate results.
What is an A/B Testing Sample Size?
An A/B testing sample size is the number of users or sessions required to collect accurate data when testing website elements. It determines how many visitors need to participate in your test to ensure reliable results.
Calculating the correct sample size is critical because a test with too few participants may yield inconclusive or misleading results, while an overly large sample can waste A/B testing resources.
To find the right sample size for A/B testing, you must consider factors like the expected conversion rate, the minimum detectable effect (difference between variants), and the desired statistical significance level.
A well-calculated A/B testing sample size ensures your conversion rate optimization efforts are based on valid insights which improves user experience and boosts performance.
Why Is Sample Size Important in A/B Testing?
Sample size determines the reliability and accuracy of your A/B test results. It determines the amount of data needed to draw valid conclusions and minimizes the risk of making incorrect decisions.
A sample that’s too small increases the risk of false negatives (failing to detect a true difference) or false positives (detecting a difference when none exists). Conversely, using an unnecessarily large sample wastes time and resources. Here's why AB testing sample size matters:
1. Statistical Reliability
A sufficient sample size plays a crucial role in ensuring statistical reliability in A/B testing. It minimizes the influence of random chance on the test results, allowing you to draw valid conclusions about which website element performs better.
Tests with inadequate sample sizes risk producing skewed data, as minor variations in behavior may not represent your broader audience. This lack of reliability could lead to decisions that fail to address the actual preferences and behaviors of your users.
2. Contributes to a Higher Confidence Level
A well-calculated sample size is directly linked to achieving a higher confidence level in your A/B testing results. Confidence levels indicate the likelihood that the observed differences between variations are real and not due to random chance.
On the other hand, insufficient data undermines confidence, leaving you uncertain about whether the variation you choose will deliver consistent performance. Ensuring an appropriate sample size for A/B testing enhances the credibility of your test results and instills greater trust in your optimization decisions.
3. Encourages Accurate Decision Making
Accurate data enables accurate decisions. When conducting A/B tests for website elements, the sample size determines the quality of the insights you gather. Insufficient sample sizes can lead to misleading conclusions.
For instance, A/B testers can implement a design change based on results that do not reflect the behavior of your target audience.
On the other hand, an adequate sample size ensures that decisions are informed, data-driven, and more likely to yield positive outcomes.
4. Reduces Sampling Error
Sampling error is a common challenge in A/B testing and occurs when the sample does not accurately reflect the entire population. Larger sample sizes help reduce this error, which ensures that the test results are more representative of the actual audience.
With fewer sampling errors, you can trust that your insights and subsequent decisions align with user behavior across your website which improves the effectiveness of your optimizations.
5. Resource Optimization
Resource optimization is a key consideration when determining sample size for A/B testing. Running tests with an adequate sample size prevents unnecessary expenditure of time and resources on inconclusive or misleading experiments.
While large sample sizes require more traffic or time, they guarantee that the resources invested yield actionable insights. This balance prevents wasted efforts while maximizing the impact of your testing initiatives.
6. Sample Size Directly Affects the A/B Test Power
Sample size influences A/B test power, or the ability of your test to detect true differences between variations. Low-powered tests resulting from insufficient sample sizes might fail to identify meaningful differences between website elements which can lead to missed opportunities for optimization.
A sufficiently large sample size increases test power. This ensures that your A/B test is sensitive enough to detect even small but significant differences in performance.
7. Improved Accuracy in Metrics
The accuracy of metrics like conversion rate, click-through rate, or bounce rate relies on an appropriate sample size. Larger sample sizes provide more precise measurements, giving you a clearer understanding of how each variation impacts user behavior. This improved accuracy is essential for making informed changes to your website elements.
Conversely, small sample sizes can lead to variability and uncertainty in these landing page metrics, potentially leading to false positives or negatives.
8. Helps in Detecting Meaningful Differences
Detecting meaningful differences between variations is one of the primary goals of A/B testing. With an adequate sample size, even subtle changes in performance metrics become detectable.
For example, a slight increase in the click-through rate on a call-to-action button might significantly impact overall conversions. Small sample sizes often fail to highlight such differences, leading to decisions that overlook valuable optimization opportunities.
9. Reducing Variability
User behavior naturally varies and is influenced by factors such as demographics, preferences, and external conditions. Larger sample sizes reduce the impact of this variability and provide a more stable and reliable dataset.
Outliers, such as a small group of users exhibiting different behavior, have less influence on the overall results when the sample size is sufficient. This stability allows you to make decisions based on consistent patterns rather than anomalies.
10. Achieving Statistical Significance
Statistical significance determines whether the observed differences in an A/B test are likely to be real and not due to random chance. To Achieve statistical significance, you need a sufficient number of data points to ensure that the test results are robust.
Inadequate sample sizes often lead to inconclusive tests which can leave you uncertain about which variation to implement. You need to ensure a proper sample size to enhance the likelihood of achieving statistical significance for actionable conclusions.
Overall, sample size is a foundational element of A/B testing website elements. It ensures statistical reliability, reduces errors, and contributes to informed decision-making.
A well-calculated sample size enhances test power, improves metric accuracy, and helps detect meaningful differences between variations. By reducing variability and achieving statistical significance, adequate sample sizes lead to actionable insights that drive website performance improvements.
How To Determine The Right Sample Size For A/B Testing
A/B testing is a powerful tool for optimizing website elements, but its success depends heavily on determining the correct sample size. Determining the right sample size for A/B testing website elements is crucial for drawing actionable insights.
Getting this number wrong can lead to unreliable results, wasted resources, or missed opportunities. Here are some tips to help you get it right:
1. Understand Your Current Metrics
Before diving into calculations, start by analyzing your existing website data. Identify key performance indicators (KPIs) such as:
Conversion rate: The percentage of visitors completing the desired action (e.g., signing up, making a purchase).
Click-through rate (CTR): The percentage of users clicking a particular element, like a call-to-action (CTA) button.
Bounce rate: The percentage of visitors leaving the site without taking action.
These metrics establish a baseline, which is essential for estimating the expected performance of your test variants. For example, if your current conversion rate is 5%, you’ll use this value to calculate the sample size required to detect changes.
2. Define Your Goals and Hypotheses
Clarity on your objectives is essential. What are you testing? Are you trying to increase button clicks, reduce bounce rates, or improve sign-ups? Defining these goals allows you to focus on metrics that matter.
Additionally, set a minimum detectable effect (MDE)—the smallest change in performance that you consider significant. For instance, if you expect a 5% increase in conversions, your MDE is 5%. Smaller MDEs require larger sample sizes to detect the difference.
3. Choose a Desired Confidence Level
Statistical confidence represents the probability that your results are not due to random chance. Most A/B tests use a 95% confidence level, meaning there’s only a 5% chance of a false positive (Type I error).
Increasing the confidence level to 99% reduces the chance of errors but also requires a larger sample size. Balance confidence levels and resource constraints for effective A/B testing.
4. Calculate the Statistical Power
Statistical power is the likelihood of detecting a true effect when it exists. A power level of 80% is commonly used, meaning there’s a 20% chance of a false negative (Type II error).
Higher power increases the reliability of your test results but requires more participants.
When testing website elements like headlines, images, or CTAs, prioritize reaching sufficient power to ensure meaningful results.
5. Use Online Sample Size Calculators
Manually calculating sample size can be complex, as it involves statistical formulas for confidence levels, power, and MDE. You can use online calculators to simplify the process.
You can then input the following into the calculator:
Baseline conversion rate (e.g., 5%).
Minimum detectable effect (e.g., 2%).
Desired confidence level (e.g., 95%).
Statistical power (e.g., 80%).
The tool will provide the exact sample size needed for each variant.
6. Account for Variability
Real-world data varies due to random noise or external factors. Ensure your audience segments are representative of your overall traffic.
Here is how to do it:
Random assignment: Use software to randomly assign users to test groups to minimize biases.
Seasonal variations: Run tests during periods that reflect typical user behavior, avoiding major events or holidays unless relevant.
When you account for variability, you can reduce the risk of skewed results.
7. Adjust for Traffic Splits
Most A/B tests divide traffic equally between variations (50/50). However, some scenarios require unequal splits, such as allocating only 30% of traffic to the new variation for risk mitigation. In such cases, the smaller group needs a larger sample size to achieve statistical validity. Adjust your calculations accordingly.
8. Consider the Testing Duration
Sample size directly affects how long your test will run. A/B tests should capture enough data to account for natural fluctuations in traffic such as:
Day-to-day variability: Visitor behavior can differ between weekdays and weekends.
Time-on-site patterns: Certain elements (e.g., forms) may perform differently at various times of the day.
A good rule of thumb is to run tests for at least two full business cycles (e.g., 2 weeks) to ensure comprehensive data.
9. Monitor External Influences
External factors can distort your test results. For example:
Marketing campaigns: Launching a promotion or paid ad campaign during your test can artificially inflate traffic and conversions.
Seasonal trends: Black Friday or holiday shopping can temporarily change user behavior.
Plan your test timing carefully to avoid confounding variables.
Steps for Calculating AB Testing Sample Size
Calculating the right AB testing sample size is critical for obtaining reliable, actionable insights. Without proper sample size, your A/B testing efforts may lead to inaccurate conclusions, wasting time and resources.
In this section, we’ll explore the detailed steps to calculate the ideal A/B testing sample size for website elements while incorporating key statistical concepts such as power analysis AB testing and AB test power to ensure precision and reliability.
Let’s dive in.
Step 1: Define Your Baseline Conversion Rate
The first step to calculate AB testing sample size is to define your baseline conversion rate.
The baseline conversion rate represents the current performance of your website element and serves as the starting point for calculating the sample size for A/B testing. It acts as a benchmark to evaluate whether your variation achieves significant improvement over the control.
Here’s How to Determine It:
Analyze historical data: Use analytics tools like Google Analytics, Mixpanel, or internal tracking systems to assess the performance of the element under normal conditions. For example, if you’re testing a landing page’s headline, the baseline conversion rate could be the percentage of visitors who fill out the lead capture form.
Determine the baseline rate: Example: If your website gets 10,000 visitors per month, and 500 converts by completing a purchase, the baseline conversion rate is 50010,000×100=5%\frac{500}{10,000} \times 100 = 5\%10,000500×100=5%.
Defining the baseline conversion rate directly influences the required sample size. Tests with lower conversion rates typically need a larger A/B testing sample size because detecting subtle changes is statistically more challenging.
Step 2: Set Your Minimum Detectable Effect (MDE)
Next, set your MDE. This is the smallest performance improvement you deem meaningful. It establishes the threshold for determining whether the variation’s performance is worth acting upon.
Here is How to Set MDE:
Tie it to business goals: For instance, if increasing conversions by 1% significantly boost revenue, you can set your MDE to 1%.
Balance precision and practicality: Smaller MDEs require larger sample sizes, potentially prolonging the test duration. Choose an achievable MDE based on your traffic and resources.
Example: If your baseline conversion rate is 5% and you aim to detect an increase to 6%, your MDE is 6%−5%=1%6\% - 5\% = 1\%6%−5%=1%.
Setting a clear MDE ensures your AB testing sample size aligns with the level of precision needed for meaningful insights to prevent wasted resources on trivial differences.
Step 3: Select Your Confidence Level
Once you set the MDE, the next step for calculating your AB testing sample size is to select your confidence level.
The confidence level measures the probability that your test results are not due to random chance. A standard choice is 95%, meaning there’s only a 5% likelihood of observing a false positive result (Type I error).
Some common confidence levels include:
90%: Faster results but higher risk of incorrect conclusions.
95%: A balanced approach, suitable for most tests.
99%: Offers greater certainty but requires a significantly larger sample size.
For website elements with high business impact, such as pricing pages or checkout processes, prioritize higher confidence levels to reduce risks.
Step 4: Determine Statistical Power
Statistical power measures the likelihood of detecting a true effect if one exists. A commonly used power level is 80%, meaning there’s a 20% chance of a false negative (Type II error). Power is an integral part of power analysis AB testing, as it ensures the test is sensitive enough to identify meaningful changes.
Here is How to Choose Power Levels:
80%: Standard for most A/B tests—offers a good balance of reliability and feasibility.
90%: Reduces the risk of missing true effects but increases the required sample size for A/B testing.
Incorporating AB test power into your calculations ensures that your test is well-equipped to detect meaningful changes, preventing missed optimization opportunities.
Step 5: Use a Sample Size Calculator
Calculating the AB testing sample size manually can be time-consuming and hectic. It involves complex statistical formulas that require some expert skills. The good news? You can use an online sample size calculator like Optimizely to simplify the process by providing accurate estimates based on your input.
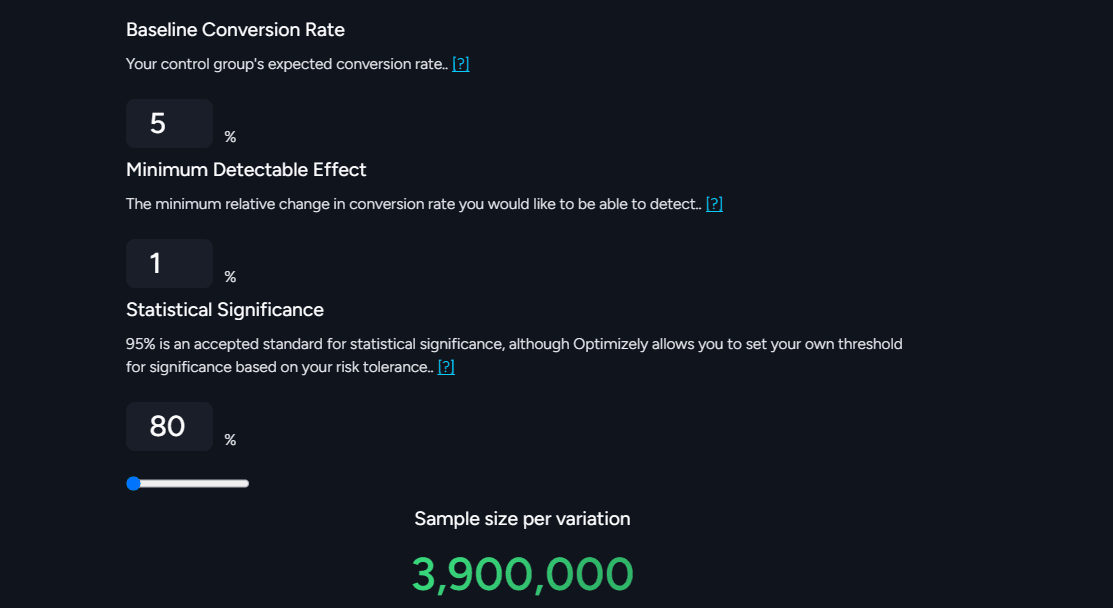
Here are the Key Inputs to use:
Baseline conversion rate (Step 1).
Minimum detectable effect (Step 2).
Statistical significance (Step 4).
Example: Suppose your baseline conversion rate is 5%, minimum detectable effect(MDE) is 1% and statistical power is 80%, your AB testing sample size is 3.900,000.
Step 6: Adjust for Traffic Allocation
While most A/B tests split traffic evenly between the control and variation groups (50/50), some scenarios may require uneven splits, such as 70% control and 30% variation. So the next step in your AB testing sample size calculation process is to adjust traffic splits.
Here is How to Adjust:
Use tools like Fibr AI to allocate traffic proportions automatically.
Ensure the smaller group has enough participants to maintain statistical validity.
Traffic allocation impacts your test’s duration and reliability. Adjust for unequal splits to ensure you maintain accurate results, even with disproportionate traffic distribution.
Step 7: Account for Variability
User behavior is rarely consistent, and variability in traffic sources, devices, or external factors can affect test outcomes. High variability demands a larger sample size to detect meaningful differences. To calculate the A/B testing sample size, account for variability.
Here’s How to Manage Variability
Segment your audience: Ensure test participants are representative of your target audience.
Avoid seasonal bias: Run tests during typical traffic periods to avoid skewed results caused by holidays or marketing campaigns.
Accounting for variability minimizes data noise which helps to deliver accurate and actionable A/B test results.
Step 8: Validate Your Assumptions
Before launching your A/B test, validate all assumptions to ensure the calculated AB testing sample size is accurate and the test design is feasible.
Follow these steps to validate your assumptions:
Verify that your baseline conversion rate reflects current performance.
Confirm your test will reach the required sample size within a reasonable timeframe.
Assess potential external influences, such as ad campaigns, that could distort results.
Step 9: Monitor the Test
Even after starting the test, ongoing monitoring is crucial. Regularly check traffic distribution and conversion metrics to ensure the test progresses as planned. Avoid stopping the test prematurely, as doing so can lead to misleading results.
Common Challenges in A/B Testing Sample Size
While A/B testing is a vital part of optimizing website performance, it comes with its own set of challenges, especially when determining the appropriate sample size. An incorrect sample size can lead to invalid results, waste resources, and delay the decision-making process.
In this section, we will explore common challenges you’re likely to face when determining the right sample size for A/B testing, and how these challenges can affect the accuracy and efficiency of website optimizations.
1. Miscalculating the Required Sample Size
One of the most fundamental challenges in A/B testing is miscalculating the required sample size. The AB testing sample size determines how many visitors need to be included in each variation to ensure statistical validity.
If the sample size is too small, the results might not be reliable, and any detected differences may be due to chance rather than actual performance differences.
Conversely, if the sample size is too large, it can lead to unnecessary resource allocation, making the test more time-consuming and expensive.
For accurate results, you should calculate the sample size for AB testing based on the following factors:
Baseline conversion rate: The current rate of success for the website element being tested.
Minimum detectable effect (MDE): The smallest change you want to detect (e.g., a 5% improvement).
Power of the test: The likelihood that the test will detect a true effect when one exists (typically set at 80% or higher).
By considering these factors, A/B testers can calculate a more accurate sample size that will provide valid results without wasting time or resources.
2. Balancing Test Duration and Traffic Availability
Website traffic volume plays a significant role in determining the duration of A/B tests. Websites with high traffic can reach the required sample size relatively quickly, enabling shorter testing periods.
On the other hand, websites with lower traffic may need extended testing periods to gather enough data, delaying the time it takes to obtain actionable insights.
Attempting to rush through a test by shortening its duration before reaching the required sample size can lead to unreliable results. This compromises the statistical validity of the A/B test, meaning decisions based on insufficient data can lead to suboptimal website improvements.
3. Accounting for Variability in User Behavior
User behavior is often variable and can be influenced by various factors such as location, device type, time of day, or even the marketing channel that brought the user to the site. This variability can complicate A/B testing and make it difficult to calculate an accurate sample size.
For example, mobile users might behave differently from desktop users, or users from different regions may interact with the website in distinct ways.
Without accounting for this variability, A/B testing results may not reflect the broader audience’s behavior and could lead to skewed conclusions. You may also need to adjust sample size calculations to account for these differences and ensure the results are generalizable to the entire audience.
4. Overlooking Statistical Significance and Test Power
Focusing solely on the sample size without considering statistical significance and test power is a common mistake in A/B testing sample size.
Statistical significance measures the likelihood that the observed results are due to something other than random chance, while test power ensures that the test is sensitive enough to detect real differences between variations.
If the A/B test power is too low, even a large sample size may fail to identify meaningful differences between variations. Ensure a balance between sample size, statistical significance, and test power to obtain accurate results and make informed decisions about website optimizations.
5. Dealing with High Drop-Off Rates
Tests involving complex website elements, such as multi-step forms, lengthy user journeys, or Facebook ads often face high drop-off rates. When users abandon the test midway through, it reduces the effective AB testing sample size, which can impact the reliability of the results.
For example, if a large number of users begin a checkout process but fail to complete it, the data from those users may not provide valuable insights. Adjust for drop-offs by recalculating the sample size or redesigning the test to account for these losses.
This can help ensure that the sample size remains adequate and that the results are not skewed by incomplete data.
6. Handling Multiple Variations
When testing multiple variations of a website element (such as an A/B/n test), the required A/B testing sample size increases. This is because the traffic needs to be evenly distributed across all variations to ensure that the results are statistically reliable.
For example, if you are testing three variations of a landing page, you will need more traffic than if you are testing just two variations.
The additional variations require larger sample sizes to ensure each version has enough data for meaningful comparisons. Failure to account for this can lead to an underpowered test, which makes it harder to detect significant differences.
7. Adjusting for External Influences
External factors like seasonal trends, ongoing marketing campaigns, or algorithm updates can impact A/B test results. For example, a sudden increase in traffic due to a viral campaign might overwhelm the website, skewing the results and leading to inaccurate conclusions.
To mitigate the impact of these factors, you may need to adjust the sample size or extend the test duration. This helps ensure that the test is not affected by short-term fluctuations or anomalies, providing more reliable insights into user behavior.
8. Ensuring Balanced Traffic Distribution
An imbalanced distribution of traffic between variations can distort test results. If one variation receives significantly more traffic than another, the results may be biased, favoring the variation with more data.
This can lead to misleading conclusions about user preferences or the effectiveness of certain website elements.
Ensure proper randomization and tracking mechanisms to ensure that traffic is evenly distributed across all variations. This ensures that each variation has an equal chance of being tested under similar conditions, allowing for more reliable and unbiased results.
9. Avoiding Early Stopping
Another major challenge in A/B testing is preventing early stopping.
Prematurely stopping an A/B test before reaching the required sample size is a dangerous pitfall.
This practice, often driven by impatience or resource constraints, can lead to false positives or negatives. A false positive occurs when a test incorrectly indicates a significant difference when there isn’t one, while a false negative occurs when a real difference is missed.
Ending a test too early can lead to hasty decisions that are not backed by solid data.
10. Reconciling Business Goals with Statistical Rigor
Business priorities often demand quick results, which can conflict with the time needed to obtain statistically significant A/B test results. While businesses may desire rapid insights to implement website changes quickly, rushing the process can undermine the quality of the testing.
Balancing business goals with the need for statistical rigor requires careful planning. Also set clear expectations to ensure that decisions are based on reliable data, without sacrificing speed or accuracy. This can involve setting realistic timelines for A/B testing, allowing enough time to reach the required sample size while still meeting business objectives.
Best Practices for Managing Sample Size in A/B Testing
Managing the sample size in A/B testing is critical to ensuring accurate and reliable results. The right sample size ensures that your findings are statistically valid and can guide effective decision-making for website optimizations.
Below are five best practices for managing the sample size for A/B testing to help maximize A/B test power and avoid common challenges.
Calculate the ideal A/B testing sample size: Calculating the A/B testing sample size accurately ensures reliable results. Consider factors like baseline conversion rates, minimum detectable effect, and AB test power. Use sample size calculators to determine the exact amount of data needed to achieve statistically significant results without wasting resources or time.
Consider test duration and traffic availability: To achieve the required sample size for A/B testing, ensure sufficient traffic is available over an appropriate duration. If traffic is low, extend the test duration. This balances the need for reliable data while preventing rushed decisions that can skew AB test power and results.
Adjust for variability in user behavior: Account for differences in user behavior such as device type or geographic location. Variability can impact the sample size for A/B testing, requiring adjustments to the calculations. A higher sample size may be needed to compensate for behavior discrepancies and ensure AB test power is maintained.
Focus on statistical significance and test power: Ensure that both statistical significance and AB test power are prioritized when determining sample size. A sample size that is too small can lead to inconclusive results, while a large sample size can ensure that even minor changes are detected, increasing the power and reliability of your test.
Monitor drop-off rates and adjust accordingly: High drop-off rates in tests involving multiple steps can reduce the effective sample size. To maintain the integrity of the results, adjust for these losses by increasing the total sample size or redesigning the test. This ensures the sample remains robust, preserving AB test power and data accuracy.
Follow these practices to manage A/B testing sample size and obtain accurate and reliable results in your tests.
Need Help Calculating Your A/B Testing Sample Size?
Executing effective A/B tests that generate the data you need to optimize your website elements and improve your campaigns is never an easy task. If it were easy, everyone would be doing it.
If you’re struggling to determine the best A/B testing sample size or aren’t sure when to start with your AB testing campaigns, our team of experts at Fibr AI are here to help you.
Talk to our CRO experts to see how we can help you.
FAQs
1.What’s the minimum A/B testing sample size needed to deliver accurate tests?
The minimum sample size for A/B testing depends on the desired statistical significance, baseline conversion rate, and expected improvement.
Generally, aim for at least 1,000 users per variation for meaningful results. A small sample can lead to misleading results, which can undermine the test's validity.
You can use an online sample size calculator to get precise estimates tailored to your test parameters for good results.
2.Why does A/B testing sample size matter?
A/B testing sample size ensures the reliability of testing results. A test with few participants risks random variations which can skew outcomes and make it hard to distinguish true performance differences.
Conversely, an adequately sized sample improves statistical confidence which ensures observed changes are due to actual differences between variations, not chance. This accuracy is crucial for making informed decisions based on test results.
3.What is a good sample size for A/B testing?
A good sample size for A/B testing balances accuracy with efficiency. For most scenarios, at least 1,000 conversions per variation is recommended. However, this number varies depending on factors like traffic volume, conversion rate, and the smallest detectable effect. Using a reliable sample size calculator can help you determine the ideal size for your specific test.
4.What is an A/B testing time frame?
The A/B testing time frame refers to the duration required to gather enough data for statistically significant results. It’s influenced by traffic volume, conversion rate, and sample size requirements.