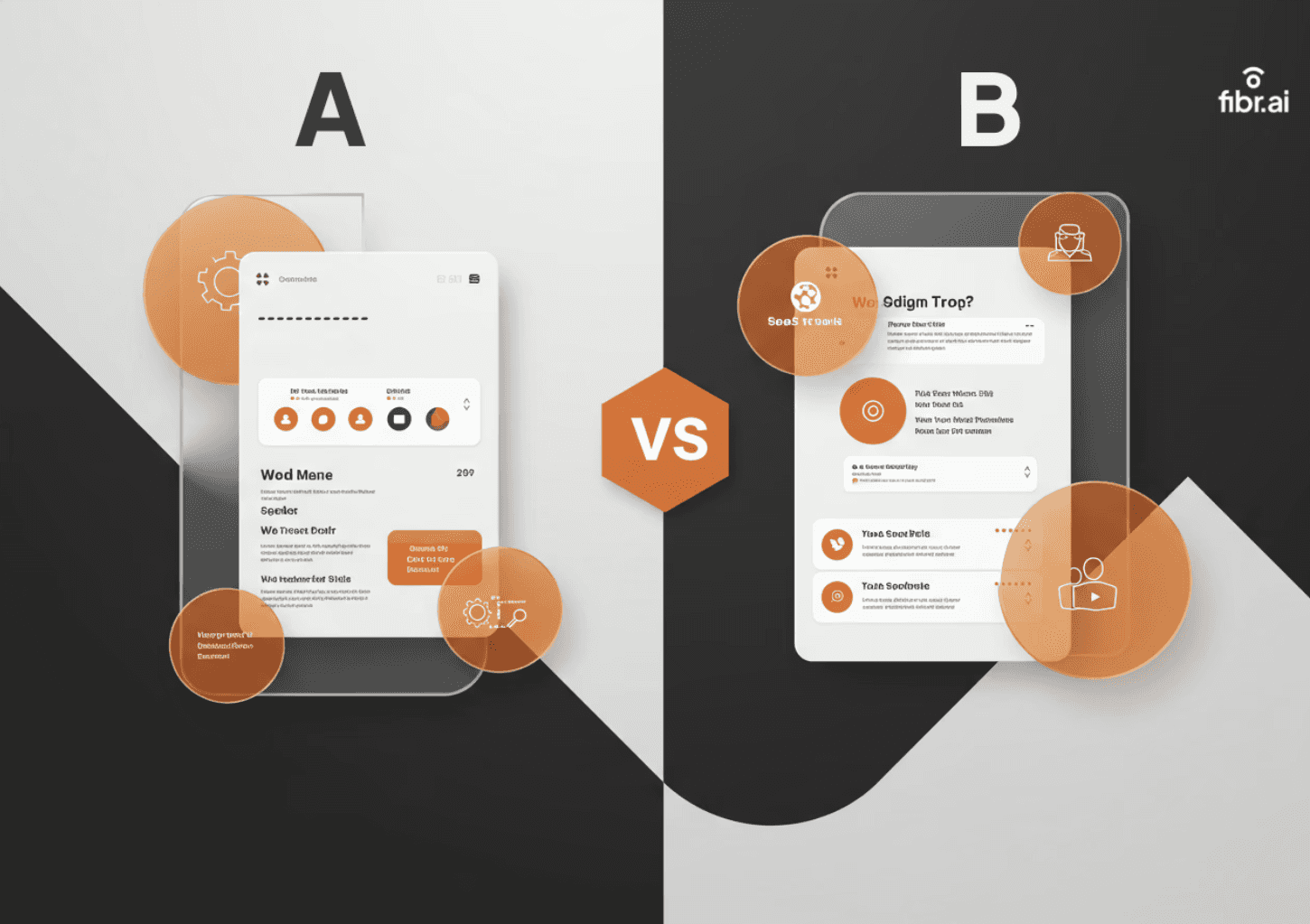

Ankur Goyal
Imagine you’re running an eCommerce website. How would you formulate the price of a product? Would you flip a coin to determine the price or rely on intuition? Your answer would be none, likely.
This is because, in modern marketing, every click, every sentence matters, and businesses cannot just rely on their mood or feelings to formulate growth strategies.
So, what’s the opposite of guesswork in the marketing world? It’s an A/B testing framework: a structured methodology that deploys user preferences effectively through finely refined data.
And no, this is not just reserved for tech-savvy companies; it’s a tool for any business or brand that’s looking at scientific ways and proven solutions to increase their leads and conversions.
What is the A/B testing framework?
A/B testing framework is a structured approach used to compare two versions–A and B of a web page or app by randomly assigning users to each version. The framework helps understand which version is performing better amongst users through metrics like conversions, engagement and more.
The framework ensures businesses have a scientific, data-driven approach to measure user response and drive conversions instead of relying on intuition or guesswork.
Fundamentally, in this process, the traffic is split between two random groups: one group receives the controlled variation or version A while the second group gets the modified variation or version B. Advanced A/B testing tools then track which version records a better response from visitors: whether through higher conversions, click-through rates (CTR), purchases, engagement, or more.
Businesses can leverage A /B frameworks to test and validate headlines, content, CTAs (call-to-action), prices, design, images, and much more. But how can a company design a winning A/B testing framework? Is the same framework applicable to all websites? What factors should be included or excluded?
We answer all your questions below.
Also read: 18 Best A/B Testing Resources In 2025
Why do you need an A/B testing framework?
Have you ever wondered how the likes of Google, Amazon, and Netflix A/B test new features, designs, and more? You’ll agree that it is definitely not random. They most likely leverage a properly defined, sophisticated A/B framework.
But, why do they do this? Or, for that matter, why does any company need an A/B testing framework? Can simply conducting the test not be enough? Actually, no!
Let’s find out why your business (like any other company) needs a proper A/B testing framework
1.Industry type
What works for a retail company will not work for a SaaS, and what works for SaaS will not work for eCommerce. This is simply because each industry has diverse needs, and the same testing strategy cannot be applied to every business type.
And, because companies operate in vastly different industries, there is a need for a tried-and-tested A/B testing framework that can eliminate guesswork and give actionable data. But, what happens when there is no predefined A/B testing framework in place? The experiments most likely will yield skewed results or data that adds no value or direction. Not to mention, it could also lead to a colossal waste of time and money.
You must also remember that A/B testing is not just about experimenting with a feature or CTA. Companies need to be able to innovate and evolve continuously without compromising their baseline or user experience. An A/B testing framework helps achieve this by optimizing the whole process of experimenting, right from allocating resources, to ultimately deriving data that can help formulate future strategies.
🤓 Did you know? In Europe, only 20% of companies A/B test their emails!
2.Planned experimentation
Another reason to favor the A/B testing framework is that it can help refine the experimentation process which typically is chaotic and error-prone. By clearly identifying a problem or opportunity, testing the hypothesis, and variants, and analyzing the results, companies can ensure their A/B testing yields results that actually move the needle.
A/B testing framework can also help define ‘success’ for a company by establishing predefined metrics. For instance, imagine a tech company launching a new feature. Now, is a 7% increase in conversion rates enough to validate and implement the new variation, ensuring all other things remain constant? How does the company come up with the ‘7%’ number? What happens if conversions are in a closer range say ‘5%-6%’ but not 7%? Should a second element be tweaked alongside? What’s the projected revenue if the feature is shipped at a 7% conversion rate vs a 5% conversion rate?
A/B testing framework can help answer all these questions. Without a framework in place, users may get misdirected, and companies would not know what went wrong, where to look, or how to address changes.
A/B tests can make or break your conversions. Partner with Fibr AI to ensure you are deploying the most advanced A/B testing tools without any hassle. To know more, click here.
Also read: Google Ads A/B Testing: A Deep Understanding
3.Improved decision making
A lot of businesses are guilt of relying on their gut when it comes to marketing. But gut feelings cannot drive growth–data can. A properly designed A/B testing frame takes the guesswork out and provides concrete evidence of what works and what does not.
IRather than speculating or assuming user behavior, businesses can deploy data driven strategies that have a 100% better chance of driving up engagement and bringing in over conversions.
4.Cost saving
Can you imagine making a huge change to a feature or webpage element and spending thousands of dollars for it to bring you negative ROI? That’s the reality of 100s of businesses today.
Wasted marketing spend is a silent killer. A/B testing framework helps optimize costs by letting business focus on strategies that actually work. Instead of blindly investing in new designs, features and more, companies can test ideas at a smaller scale before rolling them out.
This prevents expensive mistakes and ensures every marketing dollar is being spent on strategies that bring in the highest ROI.
Short, smarter experiments=Low costs and higher ROI
Also read: What Is Shopify AB Testing & How You Can Do It Too?
Successful A/B testing framework (step-by-step)
Fibr AI presents below a quick A/B testing framework to help you define and scale your A/B testing. We’ll take the example of CTA to help you understand the process better.
Define clear goals
Think of this as the ‘why’ of the whole A/B testing process. Why are you testing the CTA? What is your goal? Is it to increase CTR? Is it to reduce bounce rates? Understand which pricing works the best? Whatever the reason, your goals will quite literally be the center point and guide the whole experiment.
Formulate a hypothesis
The hypothesis takes your goals a step further by refining them. In this example, your hypothesis, for instance, could be: ‘Changing the CTA button color from blue to green can increase conversions by 20% because green is brighter.’
Remember that formulating a hypothesis is central to the A/B test. And for the same reason it has to be backed by research or analysis. Historical data and user feedback can be a good starting point for formulating your hypothesis.
Identify testing variable
Carefully choose the variable you want to test. In our example, it’s the CTA button. Now here’s what it could look like
Color: Blue vs Green
Text: ‘Buy now’ vs ‘ Add to cart’
Placement: ‘Central vs sidebar
Now, here’s the trick part. If you want to study and analyze how color change is pushing conversions, only that variable should be altered or go for testing. If you, for instance, change the color alongside the text, or change the color alongside placement, or change the color, text, and placement altogether, it would be nearly impossible to isolate which variable change actually caused the conversions to move up.
To ensure that the results are not skewed and that the variable test is yielding, ideally opt for only one variable change.
Segment your audience
This is a crucial step in your A/B framework. Segment your audience into two random groups for unbiased results: it could be based on geography, device (mobile vs. desktop), or even behavioral (high spenders vs low spenders).
You can use Fibr AI’s advanced platform or Google Analytics to instantly and scientifically segment your audience.
Create variation
The next step in this A/B testing framework would be to create two variations. Per our example, it would look something like this
Controlled version (A): Blue color to 50% of the website traffic
Modified version (B): Green color to the remaining 50% of website traffic
Determine sample size and test timing
Calculate the ideal sample size for your testing using advanced statistical tools. Too small of a sample size can render results meaningless. Conversely, an overtly large sample size can dilute or give skewed results (not to mention, can waste a lot of time and resources). So, ensure you pick the ideal sample size.
As far as testing time is concerned, for our example, a period anywhere ranging from 3-7 weeks could be ideal to see any meaningful results. Typically you must keep the testing on until you achieve a 90-95% confidence level.
Conduct the test and track results
Launch your test live, in real life. Ensure that external factors like seasonal trends, promotions, or holiday seasons do not influence the test. Next, start tracking the results.
In our case, for CTA, the ideal KPIs would be
Click-through rates
Conversion rates
Time spent on the website
Fibr AI’s efficient and AI-powered systems can help you accurately track and monitor every metric and KPI to ensure your A/B testing is a success.
Analyze the results
At this point, your A/B test is nearly complete. Compare the performance of the controlled version against the modified version.
For instance, if the green button CTA achieved 25% higher conversions at 8000 visitors, against the blue button CTA that had 10% conversion for the same traffic numbers, then the test data can be treated as statistical evidence to implement version B.
Implement and iterate
Your last step is implementing the changes: apply the winning version across the platform. But remember: The process is dynamic. The testing is officially over but, in reality, it’s not. To have continued success, testing must continue.
For instance, the green CTA can be tested alongside a text change. After that, the green button can be tested for placement. After that, the green button can be tested for a permutation and combination of text, color, and placement.
You get the gist, right?
Now, below is a quick summary of the example we discussed above;
Goal: Increase CTR by 20%
Hypothesis: A green button will perform better because it’s brighter
Variable: Button colorSegmentation: 50% of users see the blue button; 50% see the green
Variation creation: Two identical pages except for the button color
Tracking: Set up CTR and conversion metricsSample Size: 8000 users per group for statistical significance
Execution: Launch the test and monitor for external factors
Analysis: Results show the green button achieves a 25% higher conversion
Implementation: Adopt the green button and plan new tests for text optimization
Design an A/B testing framework that is specially personalized for your business.
Sign up with Fibr AI and transform your testing processes today!
How to come up with a winning A/B testing framework for your website?
Good question! After all, no two companies are the same, right?
And while this is true, you don’t want to lose leads and traffic to your competitors. Here’s how you can build a customized A/B testing framework for your business model:
If you’re a SaaS company
If you're a SaaS company, your A/B testing framework could revolve around user onboarding, churn reduction, boosting subscription rates, and such.
You could start by testing your landing page as it’s the first interaction your target user will have with your idea and company. Try testing elements like headlines, CTA placements and colors, images and videos, and more.
When it comes to features, you could test usability and appeal. For instance, if you are trying to test the visual appearance of your template, you could use a controlled version A that shows users a minimalist design and a varied version B with a splash of bright colors. The goal here is to see which version draws better conversion.
If you’re an eCommerce company
If you’re into eCommerce, ideally, your focus should first be on a smooth user experience. For the same, you could start by testing elements, like your cart design; Is the cart design clear and fast? Is there a content or review overload? Here, for instance, you can test version A with a progress bar showing the user how close they are to checking out and version B with minimal designs and faster navigation.
Similar testing styles can be deployed to your CTA buttons, discounts, and coupon cards. After all, discounts and CTAs are powerful boosters and must be designed to push your leads to take action. For example, you could test ‘10% discount’ vs ‘Buy 2 get one free’ to see which version converts better.
💡 Pro tip: Test elements that can actually influence user behavior like headlines, CTA, and more. You can use the Pareto principle or the 80/20 rule (80%of outcomes come from 20% input) to create impactful changes.
If you’re a media and publishing company
If you’re in the media industry, it is a no-brainer that your A/B testing framework’s focus should be on headlines and content.
Try to test different headline versions as they directly impact article click-through rates. For instance, version A could be: ‘10 top ways to save money' and version B could be: ‘Underrated ways to save money and cut expenses.’
If you run a subscription model, your A/B testing framework can include testing CTAs like ‘7-day free trial’ vs. ‘Read 2 articles free’ and others.
🙂 Fun Fact: Website traffic can vary more than 500% depending on the headline!
Also read: What Is Facebook A/B Testing & Why Is It Important?
If you’re a travel or hospitality company
A complicated booking interface can drive users away: so, if you’re a travel company, your A/B testing framework should be centered around a smooth booking experience.
Using A/B testing variation on hotel or cab bookings procedures, videos, visuals CTAs, and more. For instance, you could test direct discount coupons vs pop-ups that offer luxury suites at discounted prices. These smart upselling techniques could help convert more leads and generate more revenue at a fraction of cost.
If you’re a healthcare company
A healthcare company’s priority must be clear messaging and its A/B framework should be focussed around the same.
Healthcare providers can for instance test whether filling out a form vs. prompt display of appointment slots impacts conversions. Similarly, telemedicine platforms too, can test if patients prefer video calls over phone calls.
A/B testing dos and don’ts
We’ve read a lot about what A/B testing is, how the A/B testing framework works, and more. Now, let’s quickly scan some A/B testing dos and don’ts:
Dos
Test one variable at a time:
Multivariate testing can sometimes bring ambiguity to your test results. For instance, if you change the headline and CTA of your landing page simultaneously and do manage to increase conversions, it may be difficult to identify which element caused the increase.
Testing single elements can help isolate the impact and drive more meaningful results. Sure, the process can be more time-consuming but it can help build a solid understanding of your target audience and increase conversion rates more meaningfully.
Ensure statistical significance:
A/B testing can be considered meaningful only if the data has statistical significance–meaning results are data-driven and not fluke. This can only happen if you have sufficient traffic numbers.
For instance, if your baseline conversion is 4% and you want to move it up by 30%, then you may need to have a minimum of ~8000-9000 visitors to draw any meaningful result. If your total traffic, for instance, is just 5000 visitors, the results will most likely be skewed, heavily biased, and not actionable.
Target the right audience:
Segmenting your users based on demographics, preferences, spending patterns, and more are important to ensure A/B testing yields relevant results. For instance, a B2B SaaS would likely want to test advanced features with C-suite level executives, whereas an eCommerce brand would want to run discount campaigns targeting audiences for repeat purchases.
Without understanding your target audience and needs, your A/B testing could be a complete waste of time and money.
Choose the right tools:
The tools or agencies you associate with can have a direct impact on your A/B testing results. Some tools are advanced and can provide detailed analysis through heatmaps and more while others may not.
You can rely on Fibr AI when it comes to A/B testing: with advanced features, and web and ad personalization, you can create, test, and experiment with your marketing assets seamlessly. To know more, click here.
Monitor external factors:
Running testing during events like the ‘Black Friday Sale’ or holiday seasons like Christmas and Thanksgiving can result in extremely biased data as spending patterns fluctuate heavily during such times. Similarly, a small technical change to your website could mask the actual conversions happening.
It is thus important to ensure that external factors are monitored thoroughly during tests and that the experimentation happens in a controlled environment.
Don’ts
Invalidate hypothesis:
Your entire A/B testing framework hinges on the hypothesis as explained above. If your hypothesis is invalidated, there is no point to the whole test. In fact in such situations, your tests could kill conversions. You need to understand what element requires testing and when to make the most of your test.
Changing variable mid-test:
Altering a variable mid-test can be disastrous for your testing. Switching traffic on versions or changing the test variable mid-test can induce biases in the results, rendering the experiment invalid.
Commit to the test once it begins and avoid altering it midway. If you notice an issue, restart the test to ensure you get proper, unbiased, and actionable results.
Technicals to watch out for when designing your A/B testing framework
A/B testing is super technical and even a small change can drastically impact the entire framework. Below are some common technical hiccups that businesses must be aware and steer clear of:
302 redirects
When running A/B tests using different URLs, servers often employ 302 redirects (temporary) to display alternative versions. However incorrect usage of 302 directs instead of 301 (permanent) can directly impact your SEO.
Take for instance an eCommerce website testing two versions of a product page. In the case of a 302 direct, the traffic could get diluted between the variants as both could be indexed in search results, confusing buyers.
Cache and Content Delivery Systems (CDS)
Sometimes CDS and cache can store static versions of your page that can impact A/B testing. If a large set of users access static pages instead of the A and B versions, the test results would be impacted severely.
Page flicker
Ever landed on a page that twitched for a second or two and you then viewed a completely different page or design? That’s what page flickering is. Page flickering can happen due to page loading time, some coding error, or outdated hardware/software. This can impact user experience heavily and can also skew your test results.
Cloaking
Cloaking in simple terms is showing one version of content and design to users/visitors and displaying another version to search engines and bots. It is one of the many black hat-SEO tricks designed to manipulate and exploit the weaknesses of search engines. Google can heavily penalize you for cloaking and can also blacklist your website for the same.
Design a dynamic A/B testing framework with Fibr AI
A/B testing has been made unnecessarily complex. As a business, you want an A/B testing framework that is continuously evolving, dynamic, technology-friendly, and ultimately boosts conversions. But, without the right direction, things can go south pretty fast here, but not when you have Fibr AI as your partner.
Want to test two versions of your product price? Check. Need to test design and CTA versions? Done. Fibr AI! The industry’s leading AI A/B testing platform ensures that every action you take is scientific and data-driven. Design 1000s of personalized web pages, A/B test, iterate and analyze results all in seconds.
Don’t let all that traffic go to waste. With super-efficient AI-powered systems, conduct A/B tests anytime anywhere, whether you are a SaaS company or an upcoming eCommerce brand.
Book a Demo today and boost your conversions!
FAQs
What is an A/B testing framework?
An A/B testing framework is a predefined approach to comparing version A and version B of different elements of a webpage–CTA, image, app feature and more to determine which performs better in achieving a certain goal–higher conversion rate, impressions, or user engagement.
Which tool is used for A/B testing?
Google Analytics is a top analytical tool employed by businesses for A/B testing and more. Many companies also rely on Fibr AI, a top CRO and A/B testing agency for a complete strategy and planning of their ads, landing pages, and marketing experiments.
How to test A/B testing framework?
To test a typical A/B testing framework, you will first need to set clear goals, select appropriate metrics and ensure random assignments of users to variants. Make sure to monitor performance, analyze results statistically, and validate that the framework accurately identifies the more effective variant.